Robust and Self-Evolving IDS for In-Vehicle Network by Enabling Spatiotemporal Information.
HPCC/SmartCity/DSS(2019)
摘要
The Controller Area Network (CAN bus) is the most popular vehicle bus standard for communication between Electronics Control Units (ECUs) and related electronic devices. Modern vehicles are generally equipped with many (dozens of, or even hundreds of) electronic and intelligent devices so that drivers can gain a more comfortable driving experience. Protecting this increasingly complex vehicle ecosystem can be an arduous task, especially as the proliferation of data across different connected devices makes them more vulnerable than ever before. Intrusion detection systems (IDSs) have been found extremely helpful in monitoring CAN traffic and detecting potential intrusions. The paper proposes a novel and robust intrusion detection mechanism for in-vehicle CAN network by using spatiotemporal information enabled time series prediction. The proposed IDS analyzes the CAN traffic generated by the in-vehicle network in real time and identifies the abnormal state of the vehicle practically. Our model utilizes substantially both the time and space information of the instructions information sent by the ECUs in detecting the intrusions and thus outperforms the classical Long Short Term Memory model. The intrusion detection mechanism provides strong robustness in the sense that it can perform fast online learning of packet features (while the vehicle is running) to implement new protocols for intrusion detection (enabling to capture the fresh state of the vehicle) if the underlying communication protocol of the vehicle has changed. In particular, the IDS can evolve by itself as exotic environment factors (such as road conditions, atmospheric conditions, etc.) change and manage its model by itself in a self-organized way. Experiment results demonstrate the performance of the model and the effectiveness against both known and unknown attacks.
更多查看译文
关键词
in-vehicle network, intrusion detection systems, time series prediction
AI 理解论文
溯源树
样例
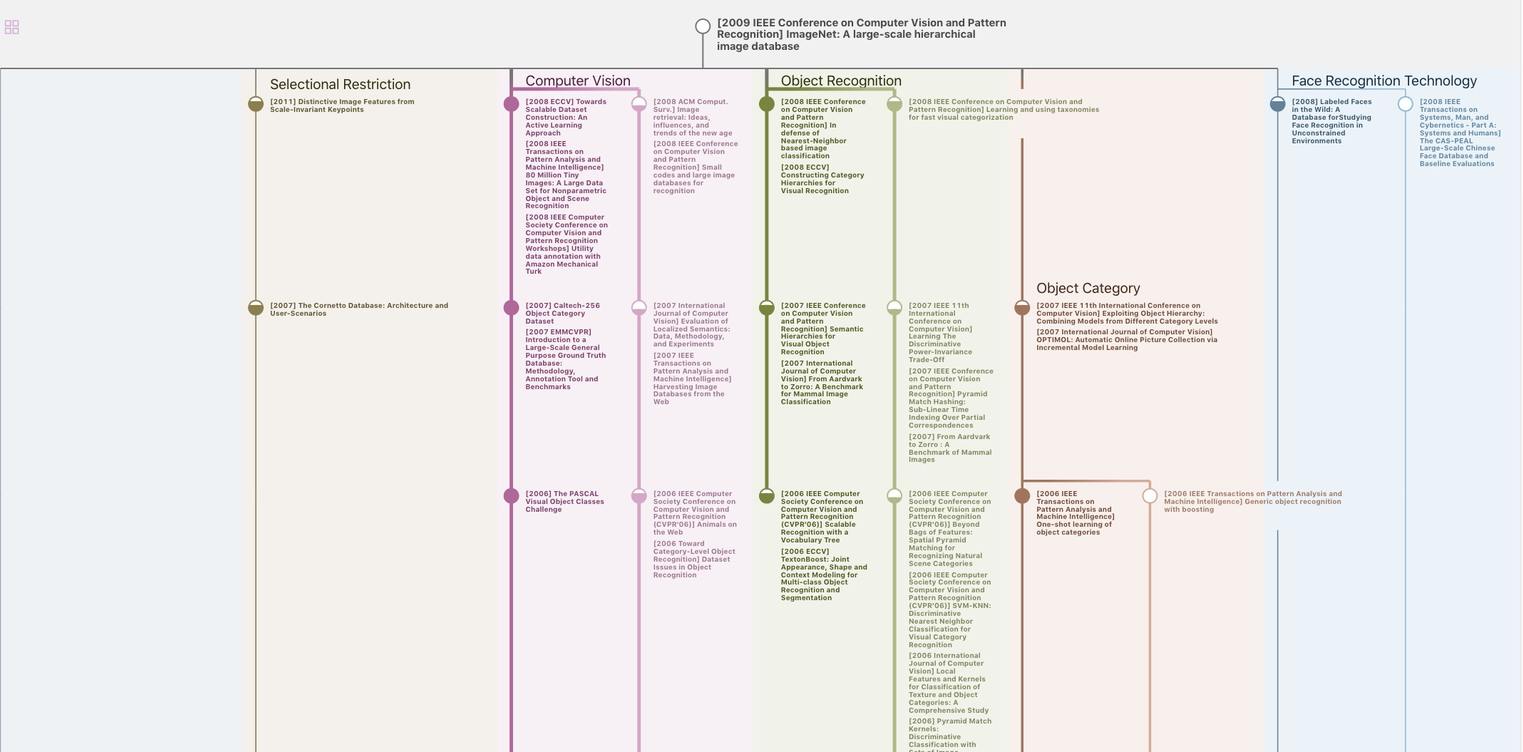
生成溯源树,研究论文发展脉络
Chat Paper
正在生成论文摘要