Partial separability and functional graphical models for multivariate Gaussian processes
BIOMETRIKA(2022)
摘要
The covariance structure of multivariate functional data can be highly complex, especially if the multivariate dimension is large, making extensions of statistical methods for standard multivariate data to the functional data setting challenging. For example, Gaussian graphical models have recently been extended to the setting of multivariate functional data by applying multivariate methods to the coefficients of truncated basis expansions. However, compared with multivariate data, a key difficulty is that the covariance operator is compact and thus not invertible. This paper addresses the general problem of covariance modelling for multivariate functional data, and functional Gaussian graphical models in particular. As a first step, a new notion of separability for the covariance operator of multivariate functional data is proposed, termed partial separability, leading to a novel Karhunen-Loeve-type expansion for such data. Next, the partial separability structure is shown to be particularly useful in providing a well-defined functional Gaussian graphical model that can be identified with a sequence of finite-dimensional graphical models, each of identical fixed dimension. This motivates a simple and efficient estimation procedure through application of the joint graphical lasso. Empirical performance of the proposed method for graphical model estimation is assessed through simulation and analysis of functional brain connectivity during a motor task.
更多查看译文
关键词
Functional brain connectivity, Functional data, Inverse covariance, Separability
AI 理解论文
溯源树
样例
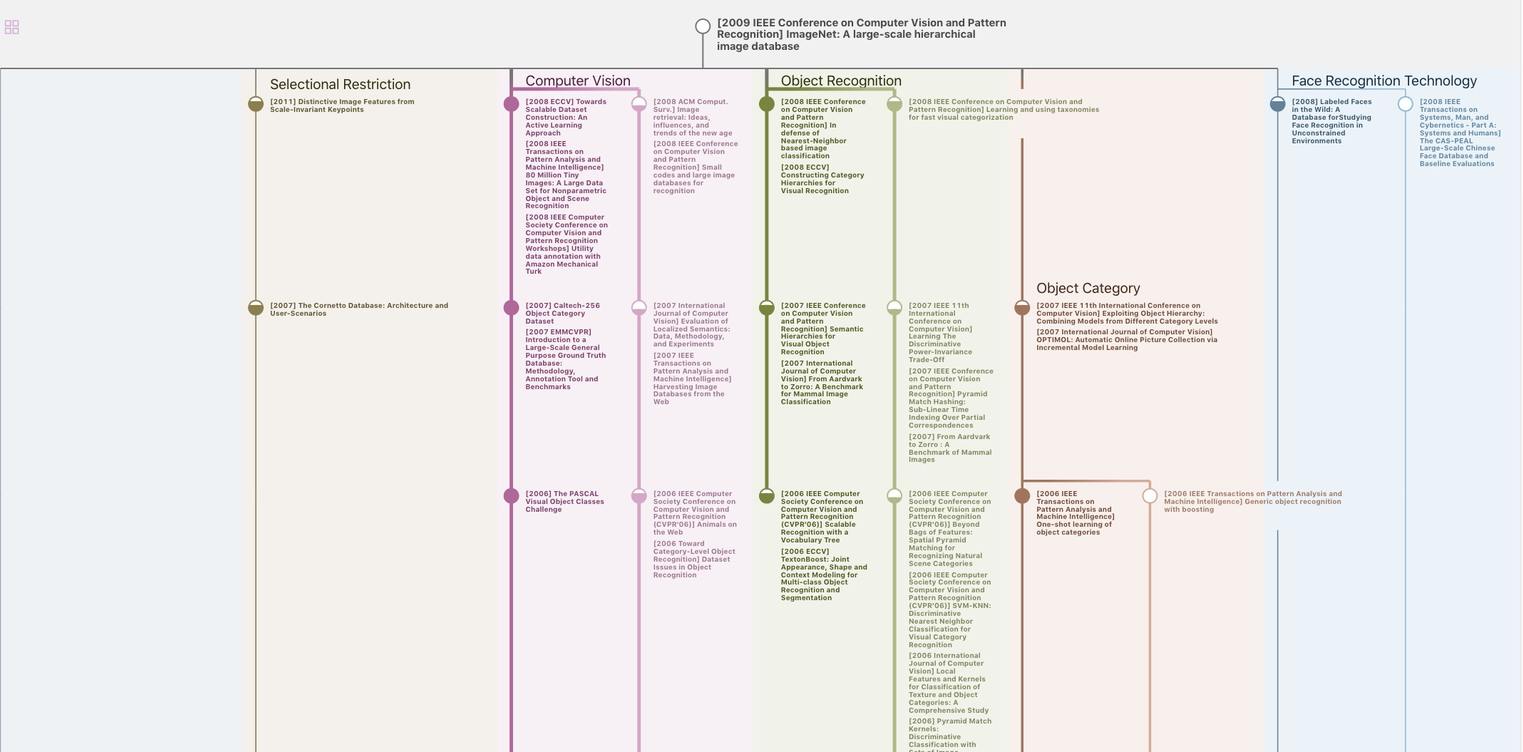
生成溯源树,研究论文发展脉络
Chat Paper
正在生成论文摘要