Scalable Nonparametric Prescreening Method for Searching Higher-Order Genetic Interactions Underlying Quantitative Traits
Genetics(2019)
Key words
Gaussian kernel models,Gaussian process regression,Haseman-Elston regression,acute myeloid leukemia,higher-order gene-by-gene interactions,nonlinear dimension reduction
AI Read Science
Must-Reading Tree
Example
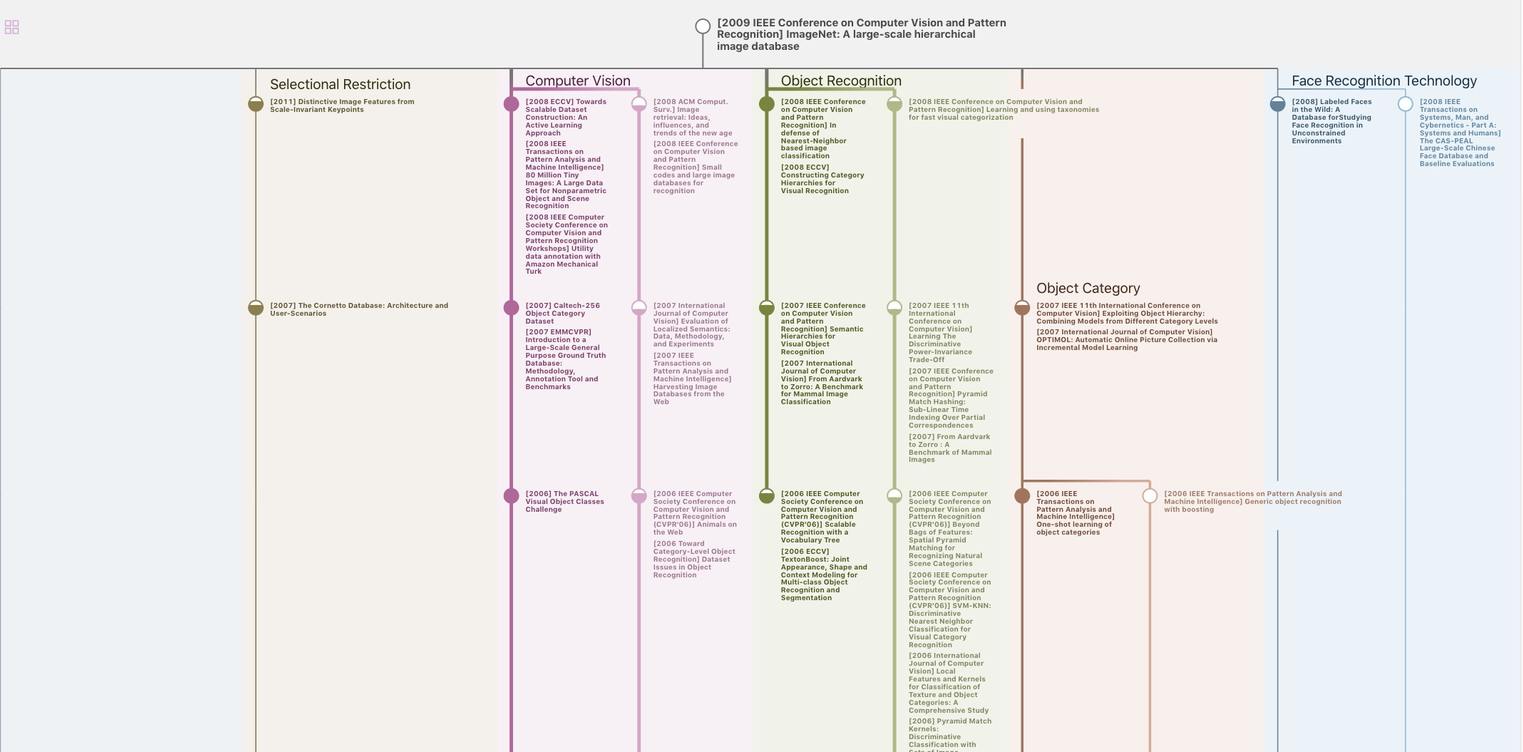
Generate MRT to find the research sequence of this paper
Chat Paper
Summary is being generated by the instructions you defined