Intrusion detection method based on information gain and ReliefF feature selection
2019 International Joint Conference on Neural Networks (IJCNN)(2019)
摘要
Traditional random forest has slow convergence in network intrusion detection and its learning performance is not perfect. In order to eliminate the redundant information in the original intrusion detection data, this paper proposes a random forest intrusion detection method based on the combination of information gain and ReliefF algorithm. The proposed method first uses the information gain to calculate the information gain value of each feature. Then, the ReliefF algorithm is used to calculate the weight of each feature. According to the information gain and the feature weight, the final feature subset is obtained. Finally, this paper uses a random forest classifier for classification. The experiment compares the three feature selection methods, including the proposed method, information gain based method and ReliefF-based method. The experimental results show that the precision, recall rate, and false positive rate of the proposed method are superior to those of the other two methods.
更多查看译文
关键词
network intrusion detection,information gain,feature selection,random forest
AI 理解论文
溯源树
样例
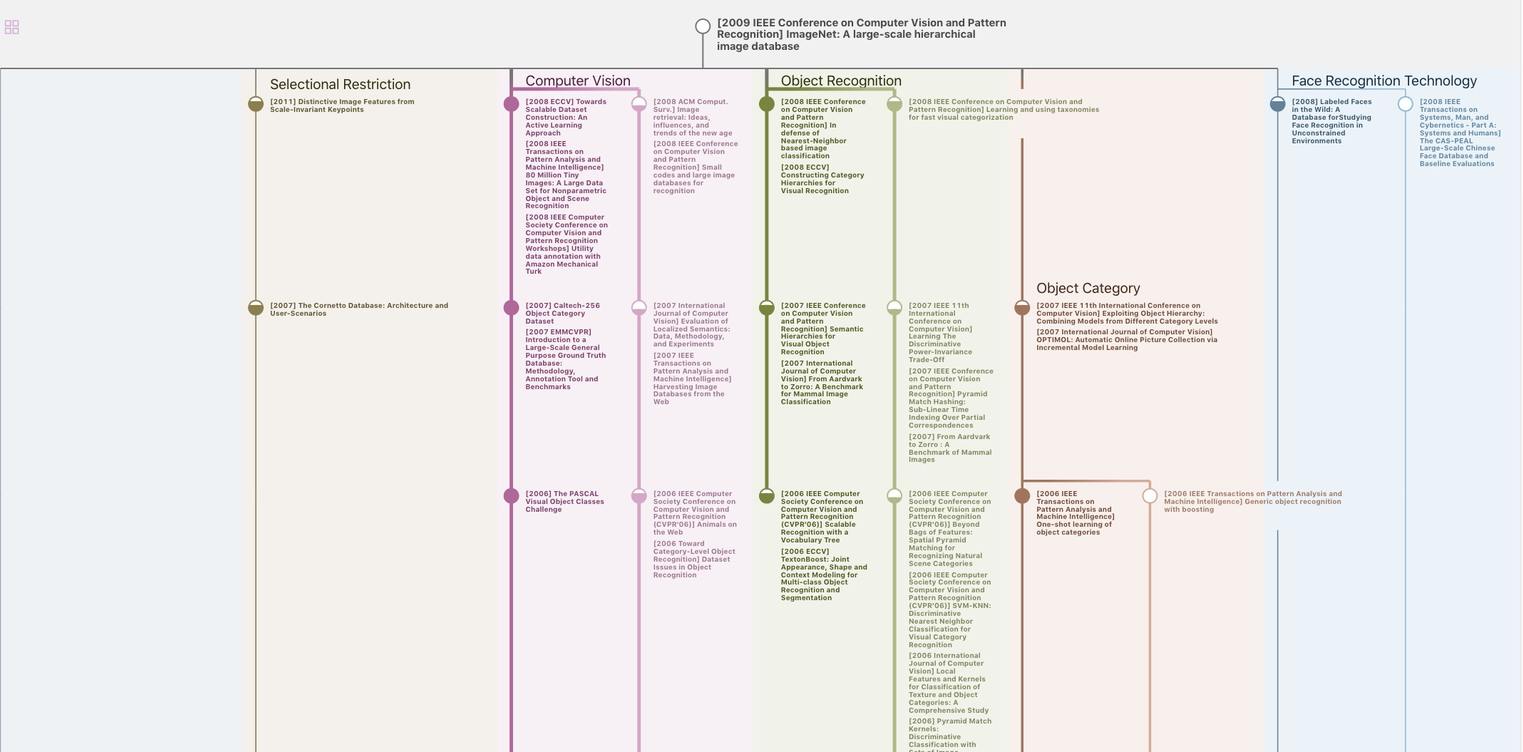
生成溯源树,研究论文发展脉络
Chat Paper
正在生成论文摘要