Image Captioning Based On Sentence-Level And Word-Level Attention
2019 INTERNATIONAL JOINT CONFERENCE ON NEURAL NETWORKS (IJCNN)(2019)
摘要
Existing attention models of image captioning typically extract only word-level attention information. i.e., the attention mechanism extracts local attention information from the image to generate the current word. We propose an image captioning approach based on self-attention to utilize image features more effectively. The self-attention mechanism can extract sentence-level attention information with richer visual representation from images. Furthermore, we propose a double attention model. The model combines sentence-level and word-level attention information to better simulate human perception system. We implement supervision and optimization in the intermediate stage of the model to solve over-fitting and information interference problems, and we apply reinforcement learning to two-stage training to optimize the evaluation metrics of the model. Finally, we evaluate our model on MSCOCO dataset. The experimental results show that our approach can generate more accurate and richer captions, and outperforms many state-of-the-art image captioning approaches on various evaluation metrics.
更多查看译文
关键词
word-level attention information,image captioning approach,image features,self-attention mechanism,sentence-level attention information,local attention information,over-fitting interference problems,information interference problems,MSCOCO dataset,reinforcement learning,visual representation
AI 理解论文
溯源树
样例
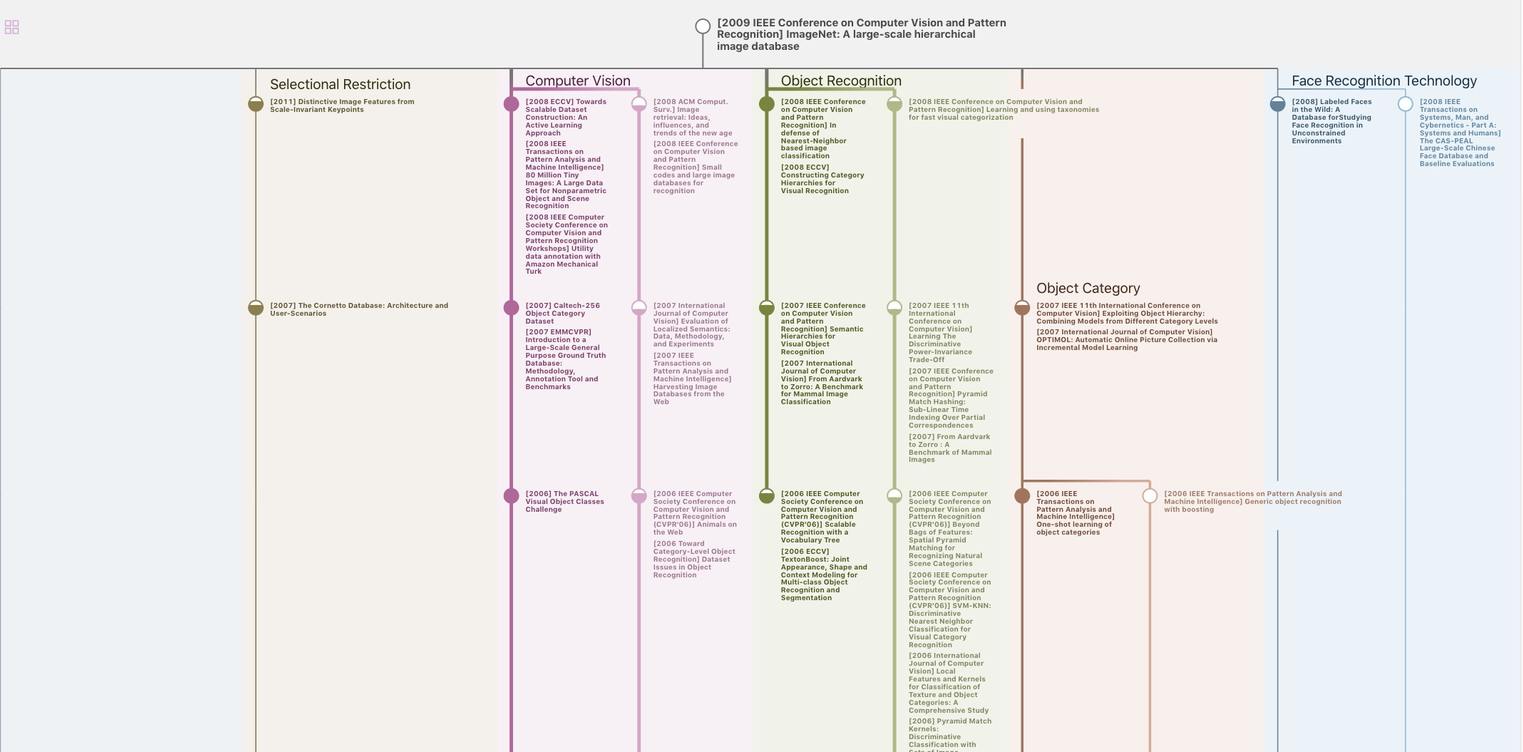
生成溯源树,研究论文发展脉络
Chat Paper
正在生成论文摘要