A Data Stratification Process for Instances Selection in Semi-Supervised Learning
IJCNN(2019)
摘要
This paper presents a study in the field of semi-supervised learning and, more specifically, it proposes changes in the self-training algorithm in order to apply a data stratification method in the labeling process of this algorithm. Therefore, this work proposes a method, called FlexCon-CS, whose objective is to apply data stratification in the inclusion of new instances in the training data set. In this sense, the representativeness and class distribution will be maintained throughout the labeling process, with the same proportions of the initially labeled dataset. In order to evaluate this proposal, we performed experiments on 27 databases with different data distribution features. Each dataset was trained with four different classification algorithms, Naive Bayes, Decision Tree, ripper, and K-Nearest Neighbor classifiers. Moreover, the Friedman statistical test was applied to provide a statistically significant analysis of the obtained results. Our findings indicate that, in most cases, the proposed methods perform better than the original self-training method.
更多查看译文
关键词
Machine learning,Semi-supervised learning,Self-training algorithm
AI 理解论文
溯源树
样例
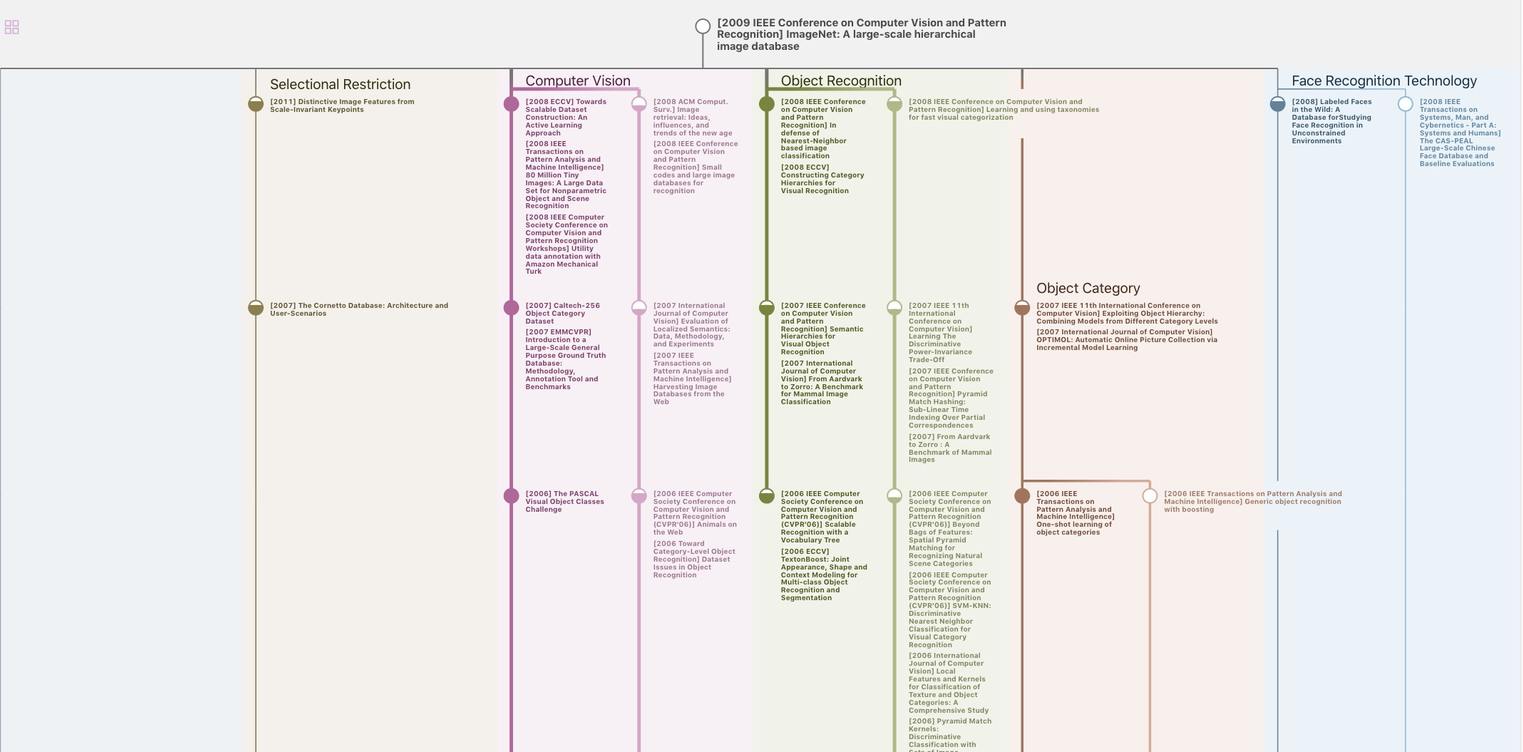
生成溯源树,研究论文发展脉络
Chat Paper
正在生成论文摘要