Efficient And Scalable Exploration Via Estimation-Error
2019 INTERNATIONAL JOINT CONFERENCE ON NEURAL NETWORKS (IJCNN)(2019)
摘要
Exploring efficiently in complex environments is still a challenging problem in reinforcement learning. Recent exploration algorithms based on "optimism in the face of uncertainty" or intrinsic motivation achieved promising performance in sparse reward settings, but they often rely on additional structures which are hard to build in large scale problems. It renders them impractical and hinders the process of combining with reinforcement learning algorithms. Hence, the most state-of-the-art RL algorithms still use the naive action space noise as exploration strategy. In this paper, we model the uncertainty about environment through agent's ability to estimate the value across state and action space. Then, we parameterize the uncertainty by a neural network and regard it as a reward bonus signal to reward uncertain states. In this way, we generate an end-to-end bonus which can scale to complex environments with less computational cost. In order to prove the effectiveness of our method, we evaluate it on the challenging Atari 2600 games. We observed that our method achieves superior or comparable exploratory performance compared to action space noise in all environments, including environments whose rewards are sparse. The results demonstrate that our exploration method can motivate agent to explore effectively even in complex environments and it generally outperforms the naive action space noise.
更多查看译文
关键词
reward bonus signal,uncertain states,complex environments,exploration method,estimation-error,sparse reward settings,reinforcement learning algorithms,exploration strategy,Atari 2600 games,action space noise,neural network
AI 理解论文
溯源树
样例
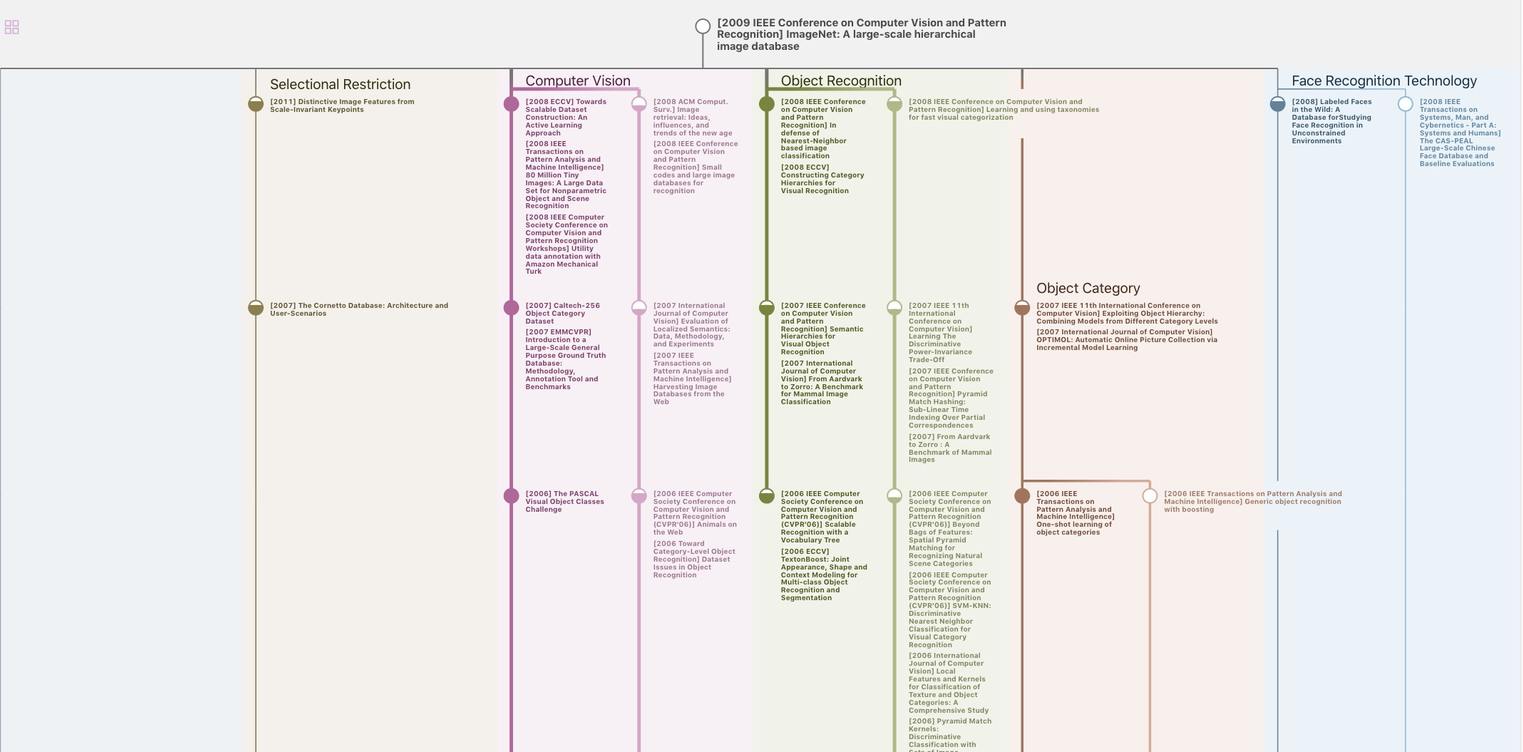
生成溯源树,研究论文发展脉络
Chat Paper
正在生成论文摘要