A Hybrid Convolutional Approach For Parking Availability Prediction
2019 INTERNATIONAL JOINT CONFERENCE ON NEURAL NETWORKS (IJCNN)(2019)
摘要
Parking availability prediction is rapidly gaining interest within the community as an operationally cheap approach to identifying empty parking locations. Parking locations accommodate multiple vehicles and are rarely completely occupied. This makes it difficult to predict occupied locations without the augmentation of external data, as the data becomes highly imbalanced. Existing forecasting models neither encapsulate the heterogeneous modes/types of parking data, nor can handle sparse measurements. The problem is formulated as a binary forecasting task, based on the parking occupancy information. In this paper, we propose a new convolutional hybrid model that is capable of capturing long term temporal dependencies and outperforming conventional time-series forecasting benchmarks on two types of parking data, namely on- and off-street parking. The performance of the proposed model is further boosted by integrating external features such as location identifiers, as well as local/global statistics. An extensive experimental evaluation proves that the proposed model is capable of handling sparse data by maintaining high precision and recall across different sparsity levels, which are controlled by empirically adjusting the occupancy cut-off threshold, as well as for multiple horizons, with an average F1 score improvement of 4.13% over strong off-the-shelf baselines.
更多查看译文
关键词
Data science, parking availabiltiy, time series forecasting, Recurrent neural networks, convolutional neural networks
AI 理解论文
溯源树
样例
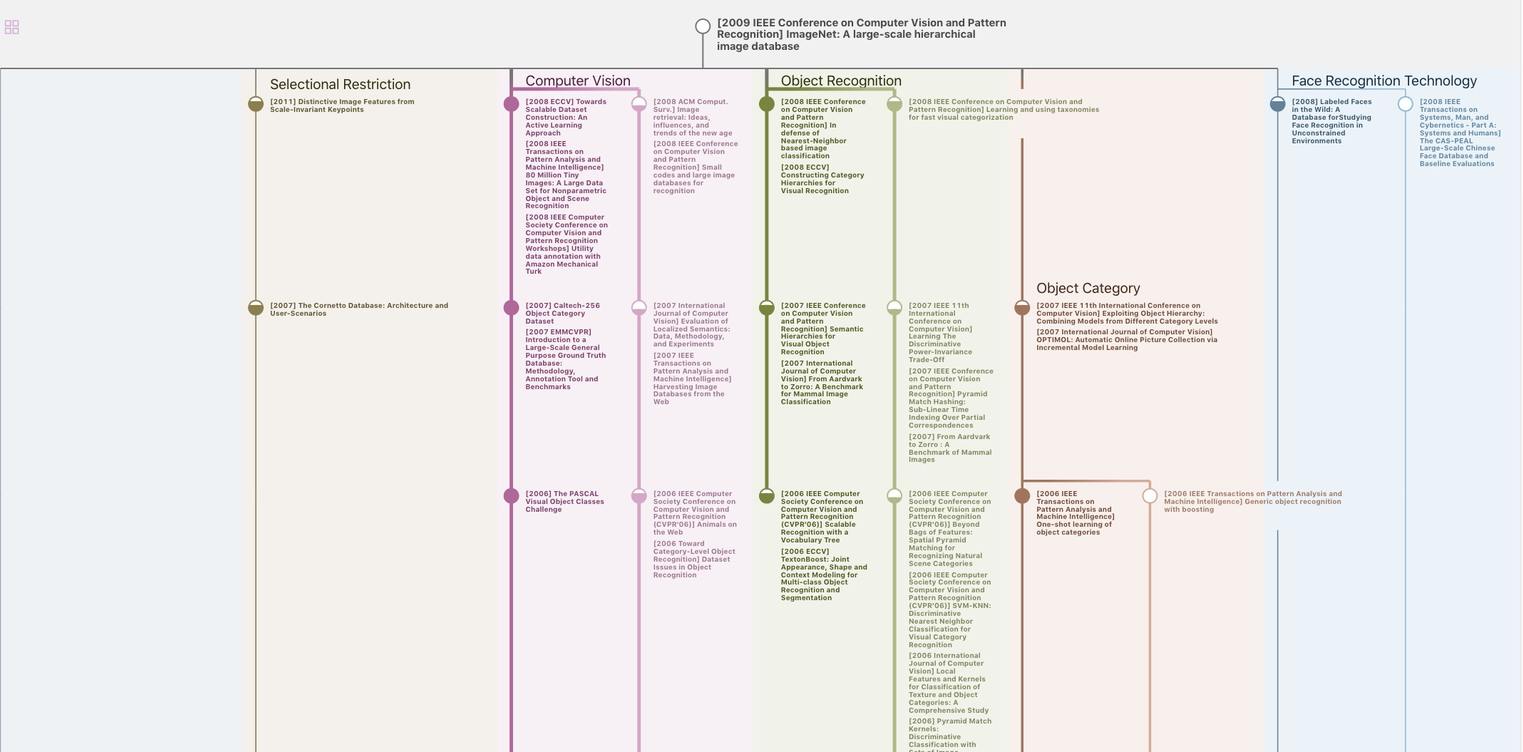
生成溯源树,研究论文发展脉络
Chat Paper
正在生成论文摘要