Removing Movable Objects From Grid Maps Of Self-Driving Cars Using Deep Neural Networks
2019 INTERNATIONAL JOINT CONFERENCE ON NEURAL NETWORKS (IJCNN)(2019)
摘要
We propose a technique for removing traces of movable objects from occupancy grid maps based on deep neural networks, dubbed enhanced occupancy grid map generation (E-OGM-G). In E-OGM-G, we capture camera images synchronized and aligned with LiDAR rays, semantically segment these images, and compute which laser rays of the LiDAR hit pixels segmented as belonging to movable objects. By clustering laser rays that are close together in a 2D projection, we are able to identify clusters that belong to movable objects and avoid using them in the process of generating the OGMs - this allows generating OGMs clean of movable objects. Clean OGMs are important for several aspects of self-driving cars' operation (i.e., localization). We tested E-OGM-G using data obtained in a real-world scenario - a 2.6 km stretch of a busy multi-lane urban road. Our results showed that E-OGM-G can achieve a precision of 81.19% considering the whole OGMs generated, of 89.76% considering a track in these OGMs of width of 12 m, and of 100.00% considering a track of width of 3.4 m. We then tested a self-driving car using the automatically cleaned OGMs. The self-driving car was able to properly localize itself and to autonomously drive itself in the world using the cleaned OGMs. These successful results showed that the proposed technique is effective in removing movable objects from static OGMs.
更多查看译文
关键词
Occupancy Grid Maps, Self-Driving Cars, Deep Neural Networks
AI 理解论文
溯源树
样例
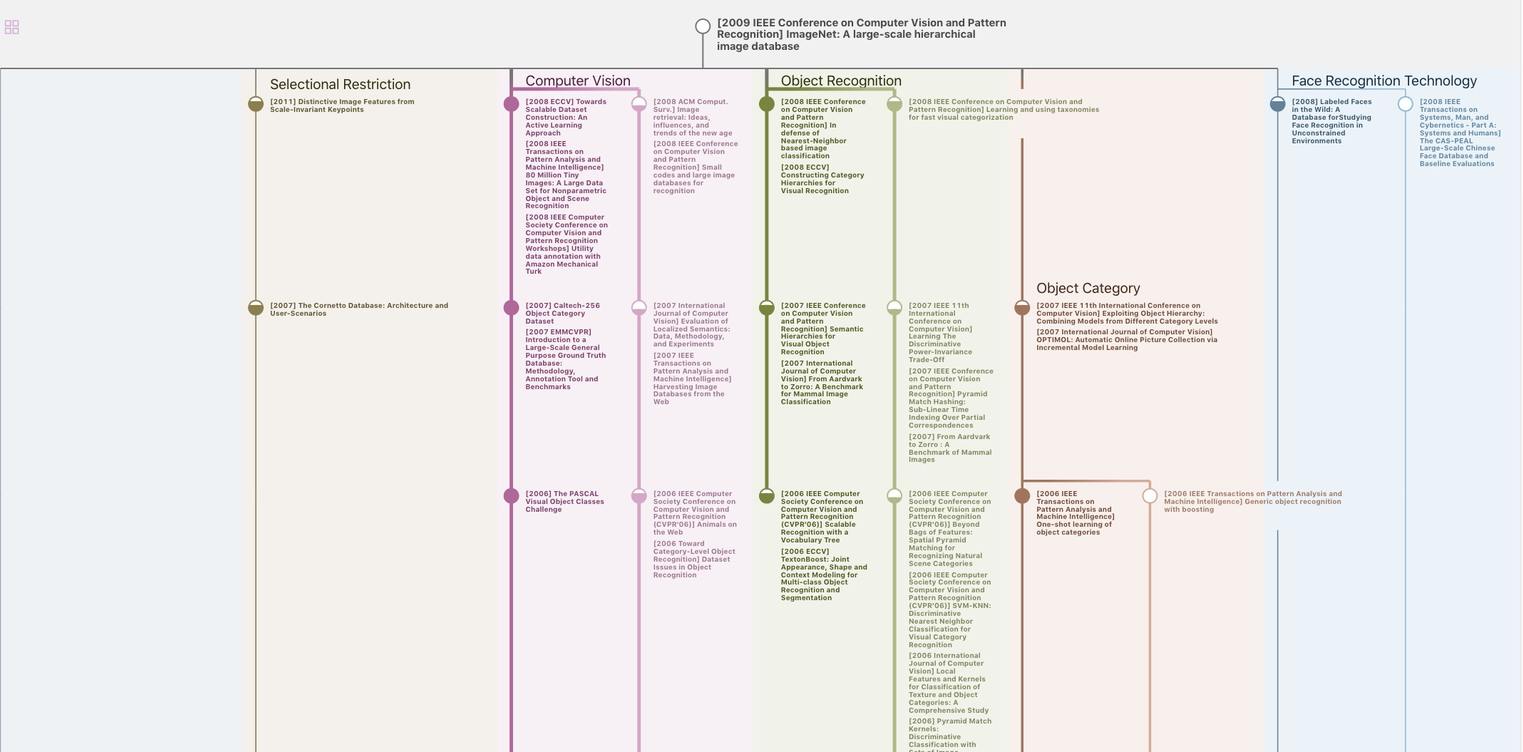
生成溯源树,研究论文发展脉络
Chat Paper
正在生成论文摘要