Training-ValueNet: Data Driven Label Noise Cleaning on Weakly-Supervised Web Images
2019 Joint IEEE 9th International Conference on Development and Learning and Epigenetic Robotics (ICDL-EpiRob)(2019)
Abstract
Manually labelling new datasets for image classification remains expensive and time-consuming. A promising alternative is to utilize the abundance of images on the web for which search queries or surrounding text offers a natural source of weak supervision. Unfortunately the label noise in these datasets has limited their use in practice. Several methods have been proposed for performing unsupervised label noise cleaning, the majority of which use outlier detection to identify and remove mislabeled images. In this paper, we argue that outlier detection is an inherently unsuitable approach for this task due to major flaws in the assumptions it makes about the distribution of mislabeled images. We propose an alternative approach which makes no such assumptions. Rather than looking for outliers, we observe that mislabeled images can be identified by the detrimental impact they have on the performance of an image classifier. We introduce training-value as an objective measure of the contribution each training example makes to the validation loss. We then present the training-value approximation network (Training-ValueNet) which learns a mapping between each image and its training-value. We demonstrate that by simply discarding images with a negative training-value, Training-ValueNet is able to significantly improve classification performance on a held-out test set, outperforming the state of the art in outlier detection by a large margin.
MoreTranslated text
Key words
mislabeled images,image classifier,training-value approximation network,Training-ValueNet,negative training-value,data driven label noise cleaning,weakly-supervised web images,image classification,search queries,surrounding text,unsupervised label noise cleaning,held-out test set,detrimental impact,natural source,outlier detection
AI Read Science
Must-Reading Tree
Example
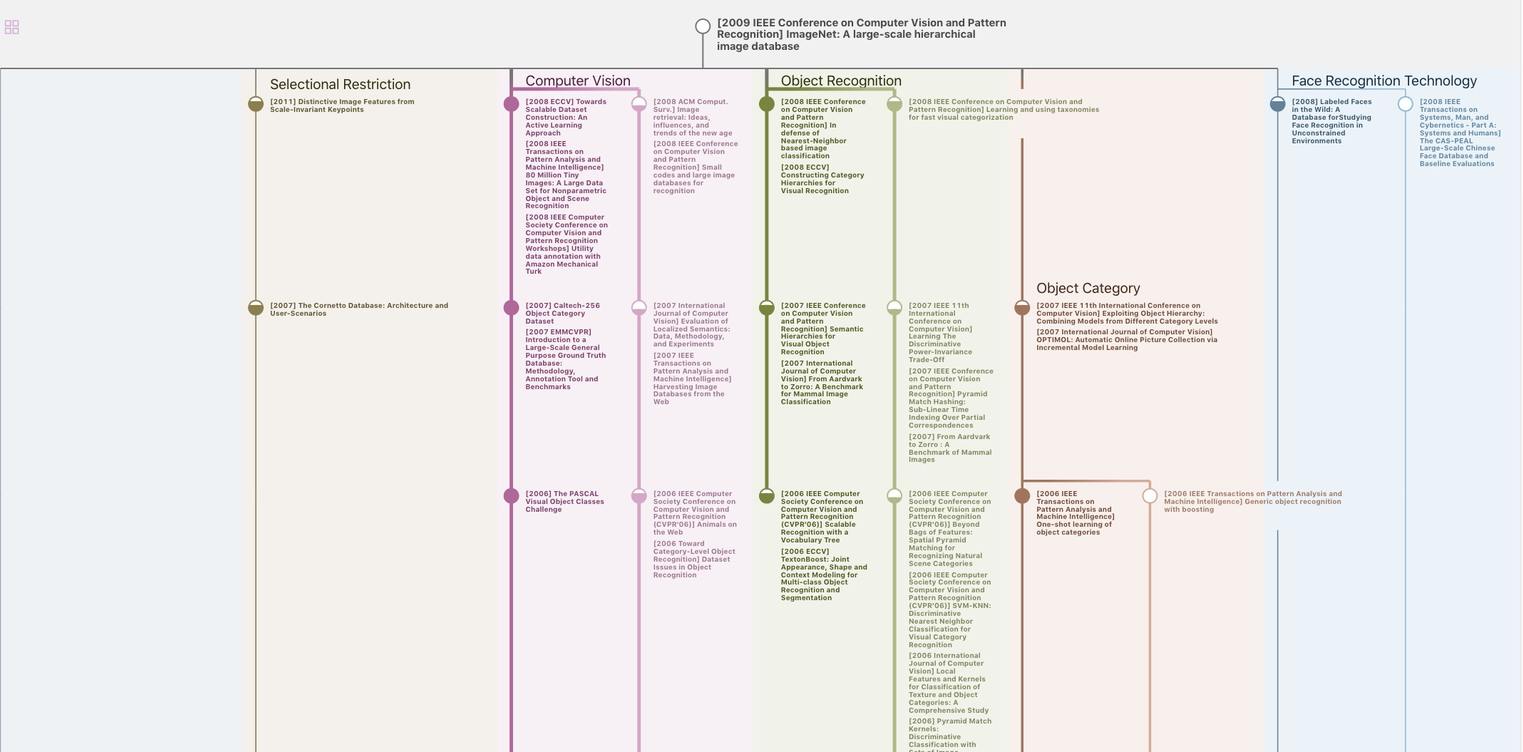
Generate MRT to find the research sequence of this paper
Chat Paper
Summary is being generated by the instructions you defined