Modified PPO-RND Method for Solving Sparse Reward Problem in ViZDoom
CoG(2019)
摘要
ViZDoom is an infamous first-person shooter game. Several studies have been conducted to develop agents that can automatically complete game tasks using a reinforcement learning algorithm. Although these studies yielded substantial progress, models proposed by the previous studies when applied to the "my way home" scenario in ViZDoom presented two problems. The first one is that when an agent walks into a specific room, it appears to be immobile and although it does not move until the time ends, the view constantly changes from left to right. The second problem is the slow learning speed of the model. To address these issues, a time penalty method and a modified neural network construction method are proposed in this study. The experimental results demonstrate that the addition of a time penalty improved the learning rate by 40% compared to the methods in which time penalty was not added. Moreover, the models proposed in previous studies could complete only 73% to 85% of the tasks, whereas the method proposed herein can complete 100% of the tasks.
更多查看译文
关键词
reinforcement learning,deep learning,game AI
AI 理解论文
溯源树
样例
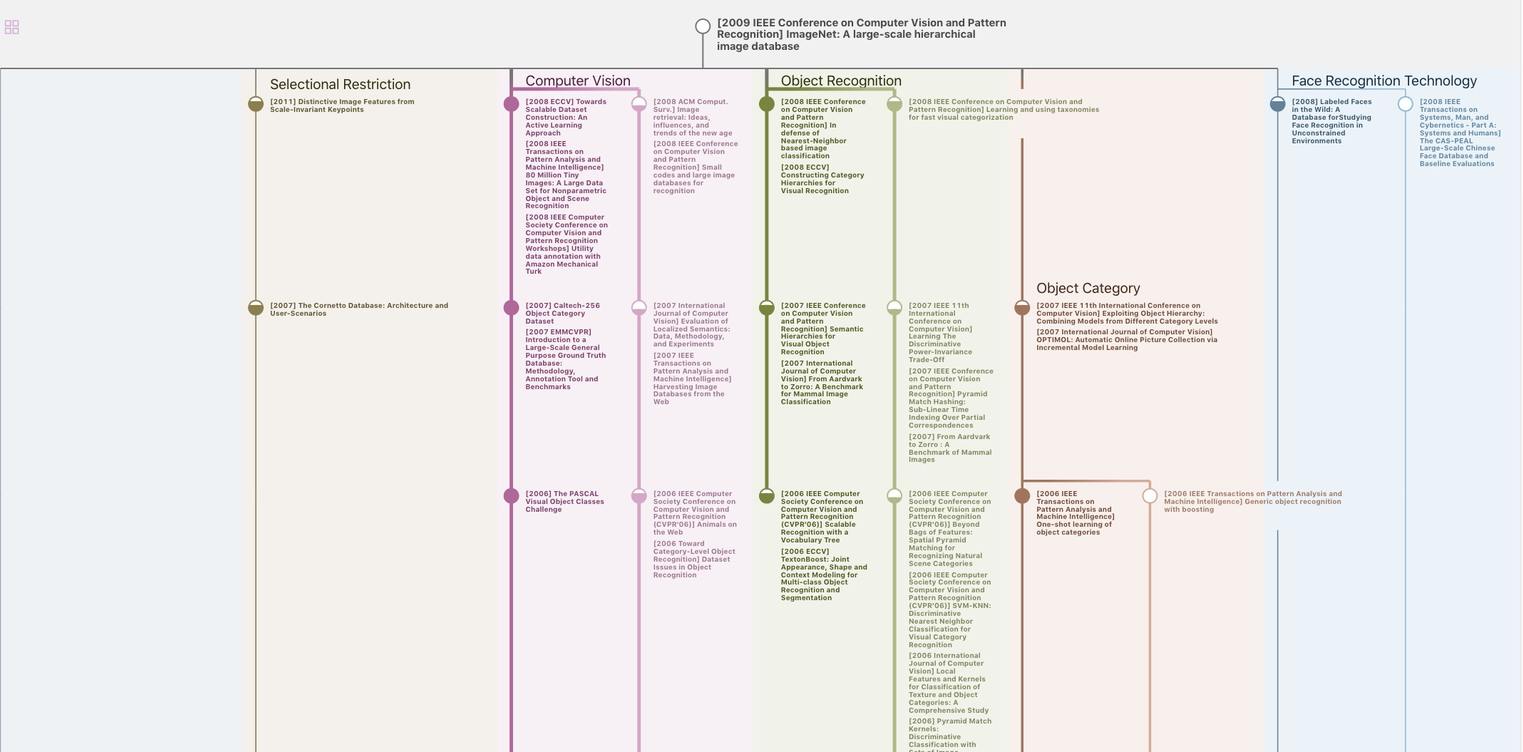
生成溯源树,研究论文发展脉络
Chat Paper
正在生成论文摘要