Learning to Select Mates in Evolving Non-playable Characters
2019 IEEE Conference on Games (CoG)(2019)
摘要
Procedural content generation (PCG) is an active area of research with the potential to significantly reduce game development costs as well as create game experiences meaningfully personalized to each player. Evolutionary methods are a promising method of generating content procedurally. In particular asynchronous evolution of AI agents in an artificial life (A-life) setting is notably similar to the online evolution of non-playable characters in a video game. In this paper, we are concerned with improving the efficiency of evolution via more effective mate selection. In the spirit of PCG, we genetically encode each agent's preference for mating partners and thereby allowing the mate-selection process to evolve. We evaluate this approach in a simple predator-prey A-life environment and demonstrate that the ability to evolve a per-agent mate-selection preference function indeed significantly increases the extinction time of the population. Additionally, an inspection of the evolved preference function parameters shows that agents evolve to favor mates who have survival traits.
更多查看译文
关键词
artificial intelligence,character generation,evolutionary computation,machine learning,multi-agent systems,reinforcement learning
AI 理解论文
溯源树
样例
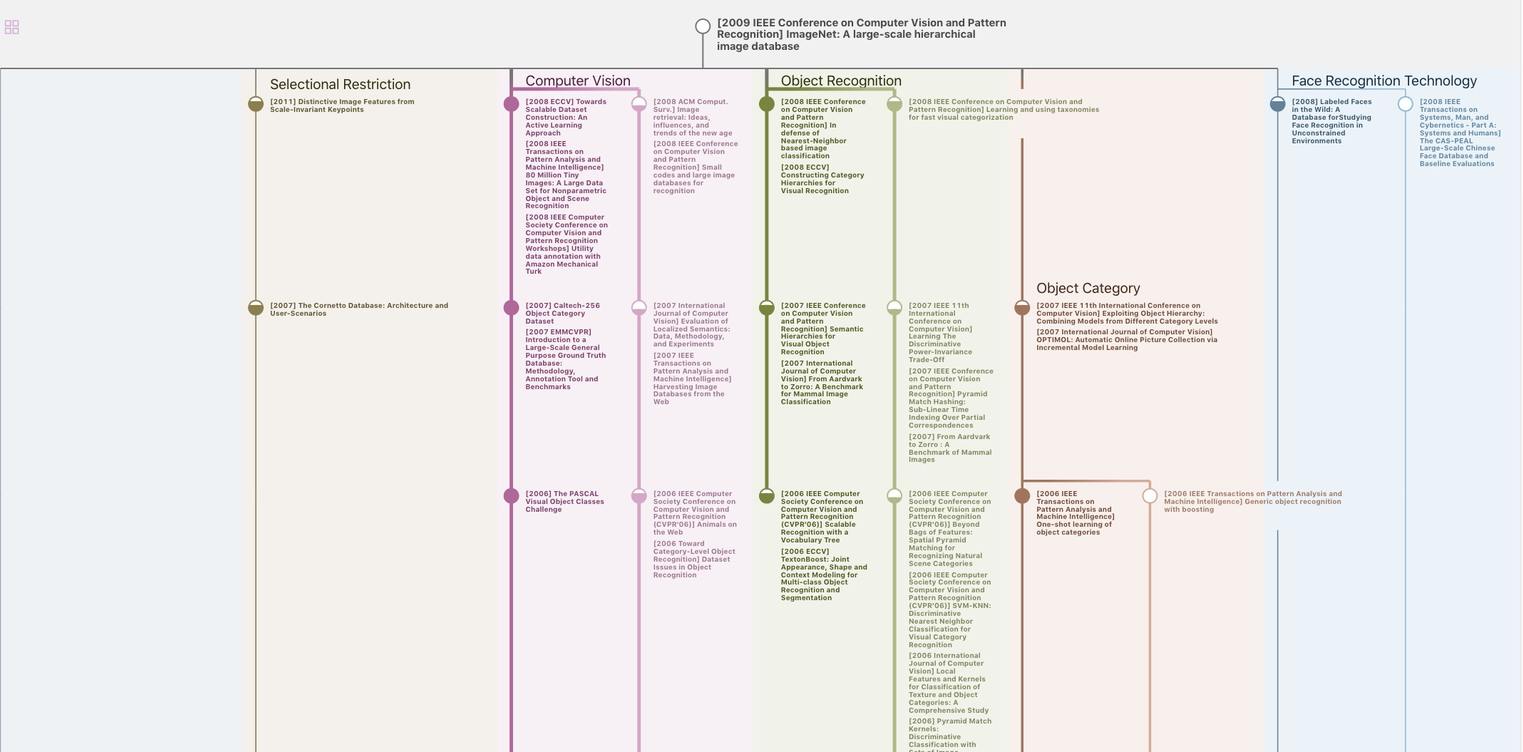
生成溯源树,研究论文发展脉络
Chat Paper
正在生成论文摘要