Learning to estimate label uncertainty for automatic radiology report parsing
arxiv(2019)
摘要
Bootstrapping labels from radiology reports has become the scalable alternative to provide inexpensive ground truth for medical imaging. Because of the domain specific nature, state-of-the-art report labeling tools are predominantly rule-based. These tools, however, typically yield a binary 0 or 1 prediction that indicates the presence or absence of abnormalities. These hard targets are then used as ground truth to train image models in the downstream, forcing models to express high degree of certainty even on cases where specificity is low. This could negatively impact the statistical efficiency of image models. We address such an issue by training a Bidirectional Long-Short Term Memory Network to augment heuristic-based discrete labels of X-ray reports from all body regions and achieve performance comparable or better than domain-specific NLP, but with additional uncertainty estimates which enable finer downstream image model training.
更多查看译文
关键词
automatic radiology report,label uncertainty
AI 理解论文
溯源树
样例
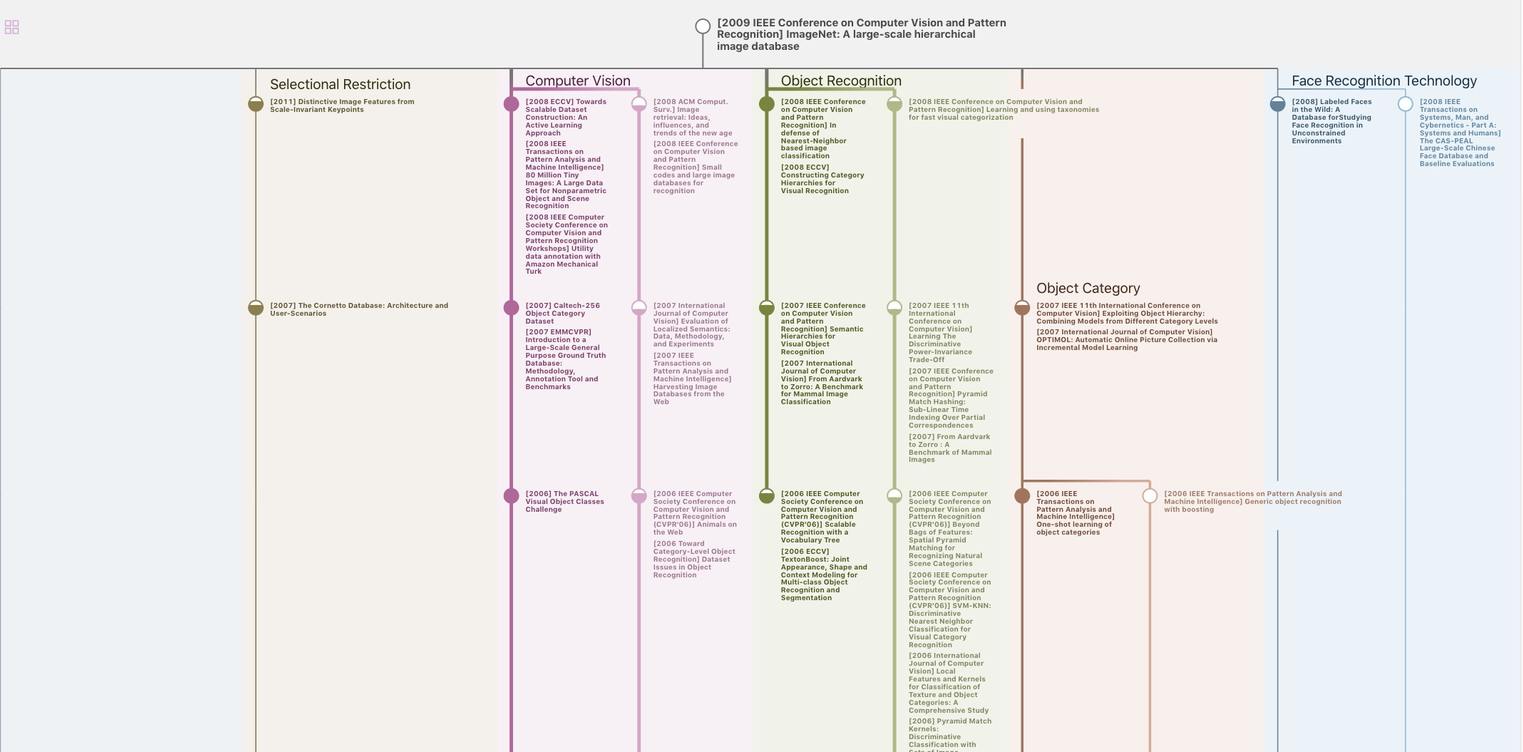
生成溯源树,研究论文发展脉络
Chat Paper
正在生成论文摘要