A Theoretical Analysis of the Number of Shots in Few-Shot Learning
CoRR(2020)
摘要
Few-shot classification is the task of predicting the category of an example from a set of few labeled examples. The number of labeled examples per category is called the number of shots (or shot number). Recent works tackle this task through meta-learning, where a meta-learner extracts information from observed tasks during meta-training to quickly adapt to new tasks during meta-testing. In this formulation, the number of shots exploited during meta-training has an impact on the recognition performance at meta-test time. Generally, the shot number used in meta-training should match the one used in meta-testing to obtain the best performance. We introduce a theoretical analysis of the impact of the shot number on Prototypical Networks, a state-of-the-art few-shot classification method. From our analysis, we propose a simple method that is robust to the choice of shot number used during meta-training, which is a crucial hyperparameter. The performance of our model trained for an arbitrary meta-training shot number shows great performance for different values of meta-testing shot numbers. We experimentally demonstrate our approach on different few-shot classification benchmarks.
更多查看译文
关键词
Few shot learning, Meta Learning, Performance Bounds
AI 理解论文
溯源树
样例
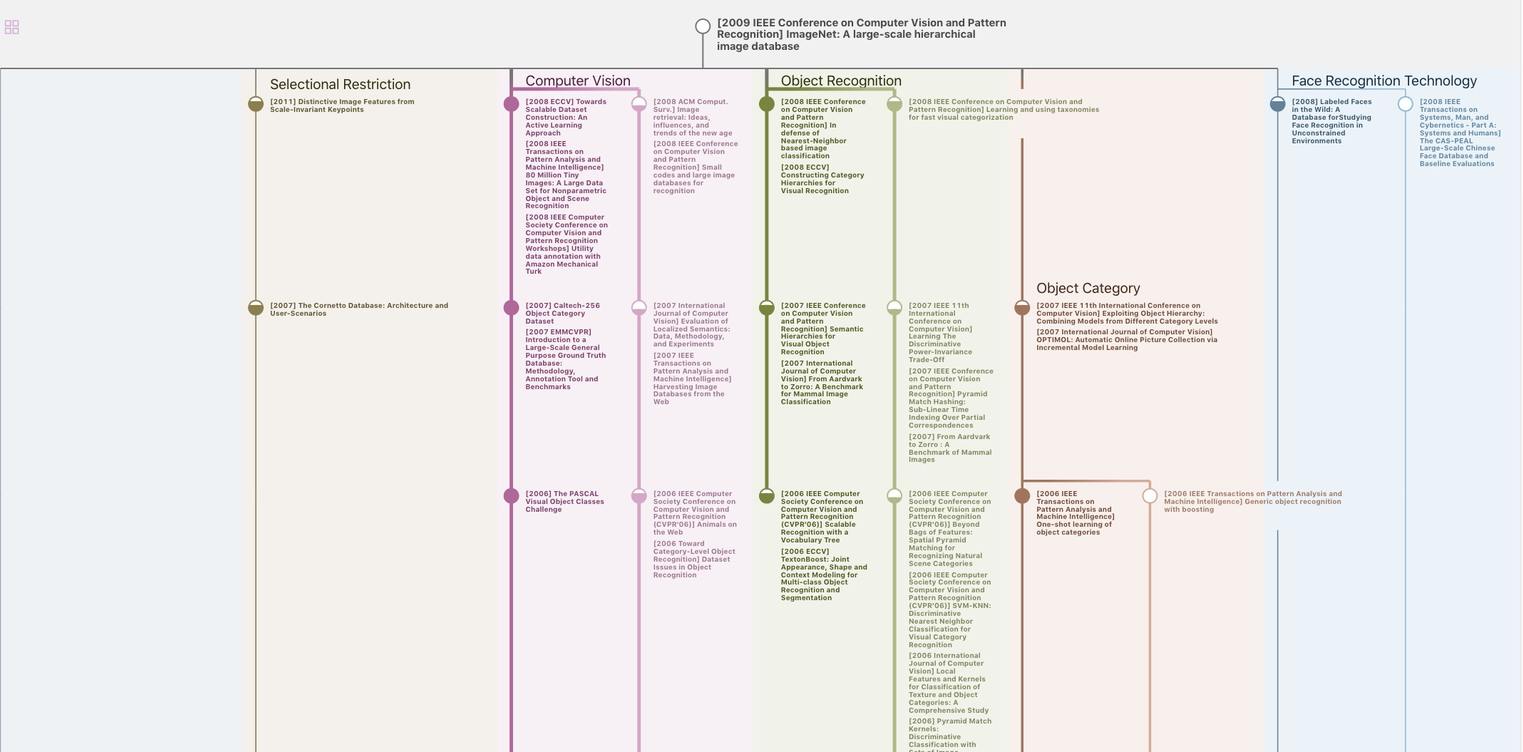
生成溯源树,研究论文发展脉络
Chat Paper
正在生成论文摘要