A Gas Analysis Model with Uninformative Variable Elimination Using Nonlinearity Compensation by RBFNN
2019 IEEE 4th International Conference on Advanced Robotics and Mechatronics (ICARM)(2019)
Abstract
Gas analysis of robotic olfaction is becoming increasingly important for environment recognition. In this work, radial basis function neural network (RBFNN) is introduced to compensate nonlinearity in the uninformative variable elimination algorithm (UVE). A nonlinear partial least squares (NPLS) replaces the partial least squares of UVE as the regression model, which is built by partial least squares integrated with RBFNN. RBFNN is adopted to address the nonlinearity of the datasets. Moreover, the regression coefficients served to evaluate the contribution of variables are calculated by NPLS. Then NPLS is performed on the selected variables which make a bigger contribution. A real flue gas spectral datasets is used to validate the proposed method. The results are compared to the NPLS with all wavelengths method and UVE combined with NPLS method. It is shown that the proposed model achieved a better performance in the variable selection and gas components prediction.
MoreTranslated text
Key words
robotic olfaction,environment recognition,radial basis function neural network,RBFNN,uninformative variable elimination algorithm,UVE,nonlinear partial least squares,regression model,flue gas spectral datasets,NPLS method,variable selection,gas analysis model,nonlinearity compensation,gas component prediction
AI Read Science
Must-Reading Tree
Example
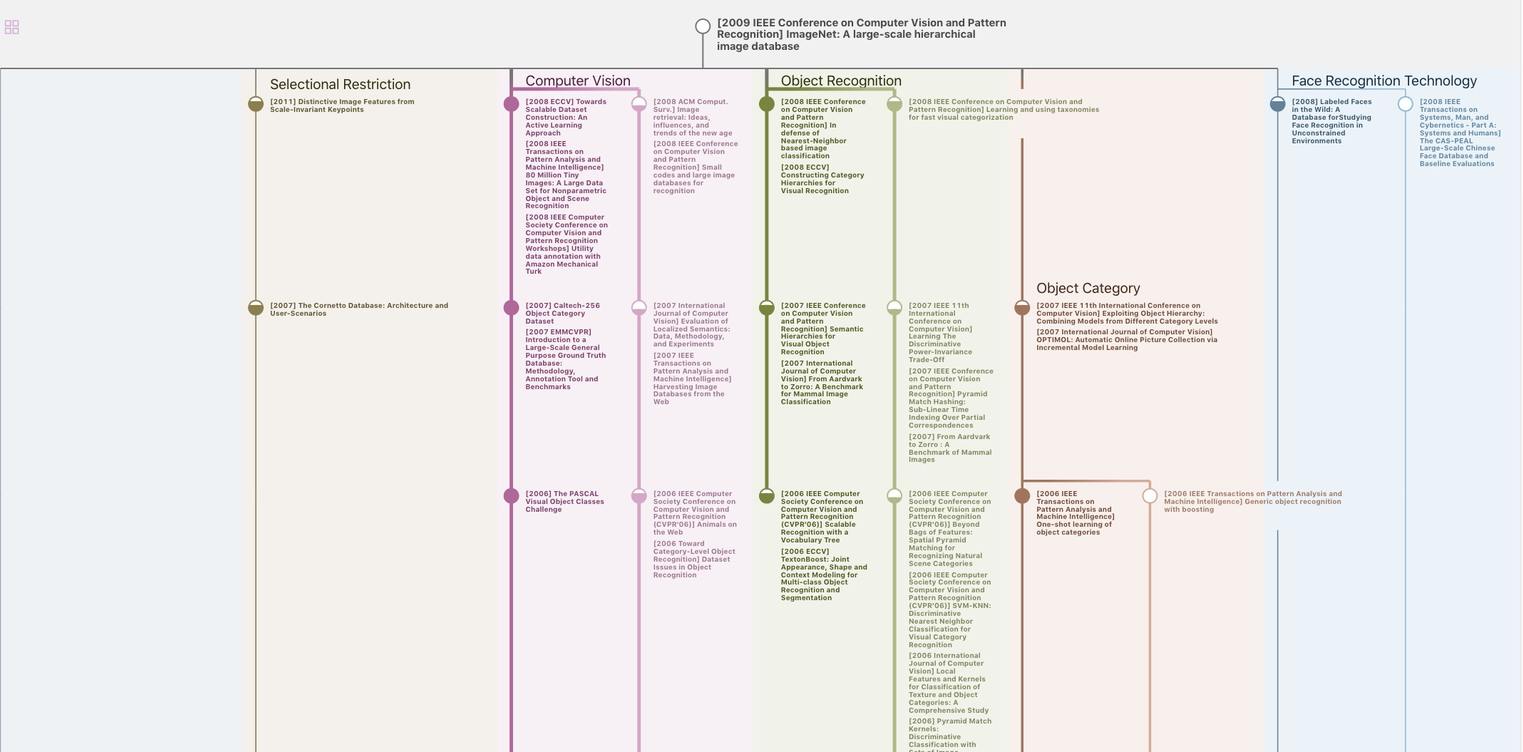
Generate MRT to find the research sequence of this paper
Chat Paper
Summary is being generated by the instructions you defined