Low-rank local tangent space embedding for subspace clustering.
Information Sciences(2020)
摘要
Subspace techniques have gained much attention for their remarkable efficiency in representing high-dimensional data, in which sparse subspace clustering (SSC) and low-rank representation (LRR) are two commonly used prototypes in the fields of pattern recognition, computer vision and signal processing. Both of them aim at constructing a block sparse matrix via linearly representing data to make them be embedded into linear subspaces. However, few datasets satisfy the linear subspace assumption in the real world. In this paper, data are peered from viewpoint of manifold architecture under the framework of sparse representation. A globally low-rank representation with the Frobenius norm minimization is constructed under the constraint of local manifold embedding and a novel low-rank local embedding representation (LRLER) model for subspace clustering of datasets is proposed. In this model, the local as well as global manifold structures of a dataset are concerned. Clusters of a dataset are considered as sub-manifolds embedded in low-dimensional subspaces. To represent and segment samples with hybrid neighbors or interlaced manifold structures, the local tangent space analysis strategy is introduced to characterize the local structure of neighborhood of samples. The coefficients of locally linear embedding are rectified according to the relationship between local tangent spaces of samples and their neighbors. A local tangent space based low-rank local embedding representation model (LRLTSER) is built to deal with data with neighborhood aliasing distortion. Extensive experiments on synthetic datasets and real-world datasets are implemented and experimental results show superior performance of the proposed methods for subspace clustering compared to the state-of-the-art techniques.
更多查看译文
关键词
Low-rank representation,Locally linear embedding,Local tangent space,Subspace clustering,Manifold learning
AI 理解论文
溯源树
样例
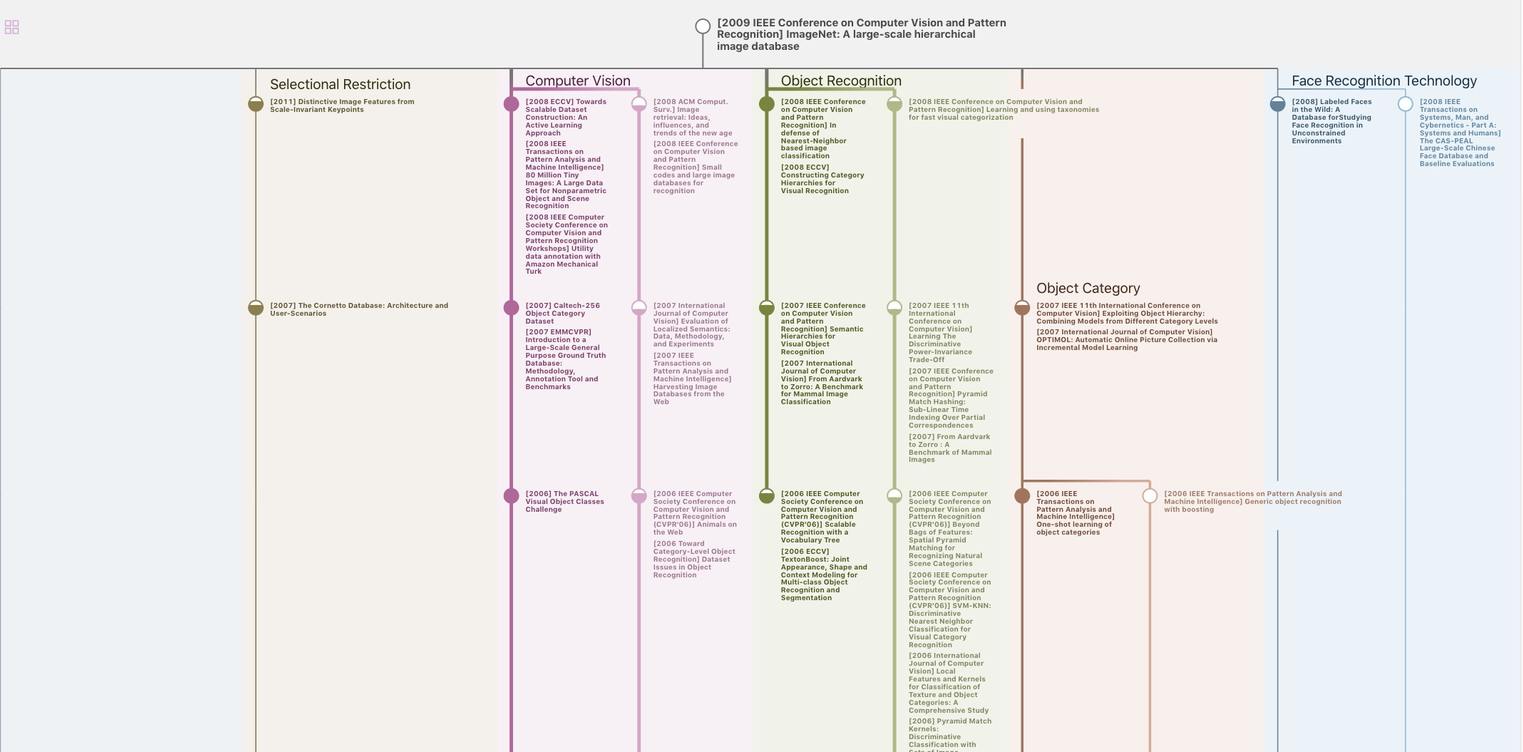
生成溯源树,研究论文发展脉络
Chat Paper
正在生成论文摘要