Machine Learning Pipelines with Modern Big Data Tools for High Energy Physics.
Computing and software for big science(2020)
关键词
Big Data,Machine Learning,HEP,Distributed computing,Parallel computing,GPU
AI 理解论文
溯源树
样例
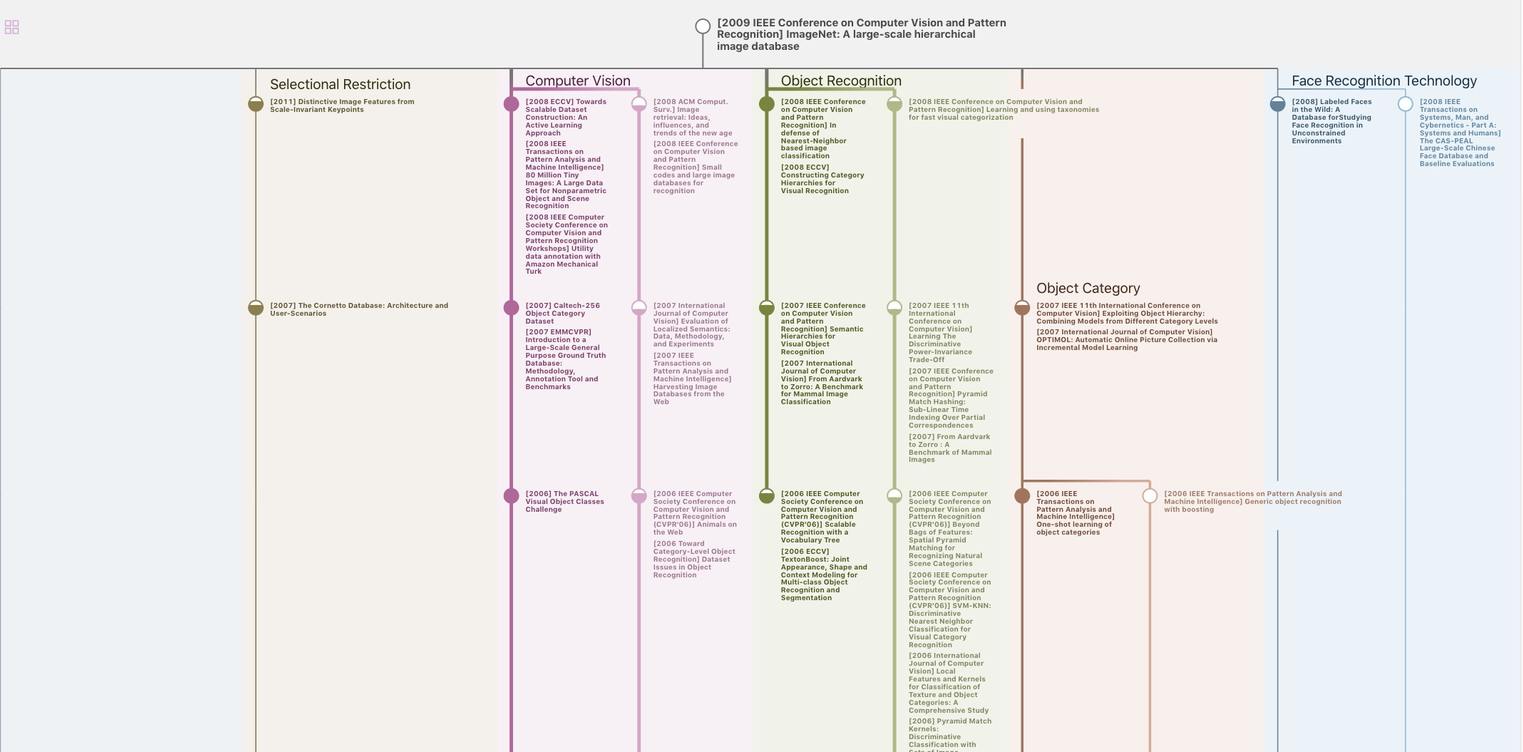
生成溯源树,研究论文发展脉络
Chat Paper
正在生成论文摘要