Sanvis: Visual Analytics For Understanding Self-Attention Networks
2019 IEEE VISUALIZATION CONFERENCE (VIS)(2019)
Abstract
Attention networks, a deep neural network architecture inspired by humans' attention mechanism, have seen significant success in image captioning, machine translation, and many other applications. Recently, they have been further evolved into an advanced approach called multi-head self-attention networks, which can encode a set of input vectors, e.g., word vectors in a sentence, into another set of vectors. Such encoding aims at simultaneously capturing diverse syntactic and semantic features within a set, each of which corresponds to a particular attention head, forming altogether multi-head attention. Meanwhile, the increased model complexity prevents users from easily understanding and manipulating the inner workings of models. To tackle the challenges, we present a visual analytics system called SANVis, which helps users understand the behaviors and the characteristics of multi-head self-attention networks. Using a state-of-the-art self-attention model called Transformer, we demonstrate usage scenarios of SANVis in machine translation tasks. Our system is available at http://short.sanvis.org.
MoreTranslated text
Key words
Deep neural networks, visual analytics, natural language processing, interpretability, self-attention networks
AI Read Science
Must-Reading Tree
Example
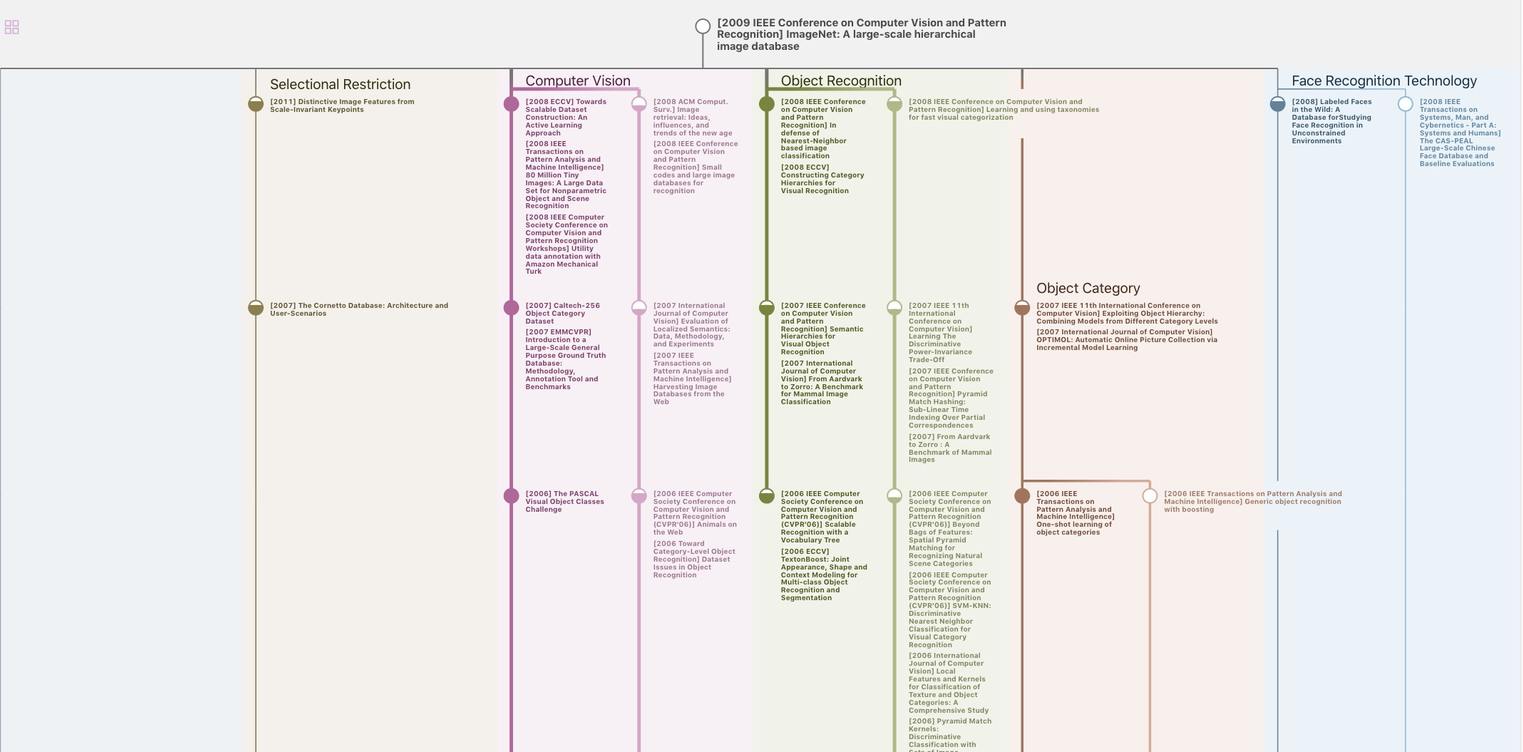
Generate MRT to find the research sequence of this paper
Chat Paper
Summary is being generated by the instructions you defined