Unsupervised Shape Co-segmentation Based on Transformation Network
Arabian Journal for Science and Engineering(2019)
摘要
Unsupervised co-segmentation is one type of shape segmentation. It segments a set of 3D shapes into meaningful parts and creates a correspondence between parts simultaneously without any labeled data. Clustering-based co-segmentation is based on the correlation analysis in a descriptor space and has received increasing attention. In this paper, we propose a co-segmentation method, in which a transformation network for data representation is trained by extreme learning machine, embedding shape primitives into more discriminant feature spaces, so as to achieve better segmentation performance. Thus, co-segmentation can be implemented by clustering on lower dimensions based on the transformation network, so the execution is more efficient. Moreover, once the transformation network is trained, it can be applied to the data representation acquisition process without re-computing similarity parameters. In order to create and train the transformation network, the correlation of shape primitives is utilized. Therefore, an affinity matrix construction method based on parameter-free and high-efficiency simplex sparse representation is introduced. This construction of correlation avoids the blindness of parameter setting. Experimental results show that the proposed co-segmentation method is effective and efficient. In addition, it also can deal with incremental co-segmentation when the dataset is expanded.
更多查看译文
关键词
3D shape co-segmentation, Unsupervised extreme learning machine, Simplex sparse representation, Data representation, Transformation network
AI 理解论文
溯源树
样例
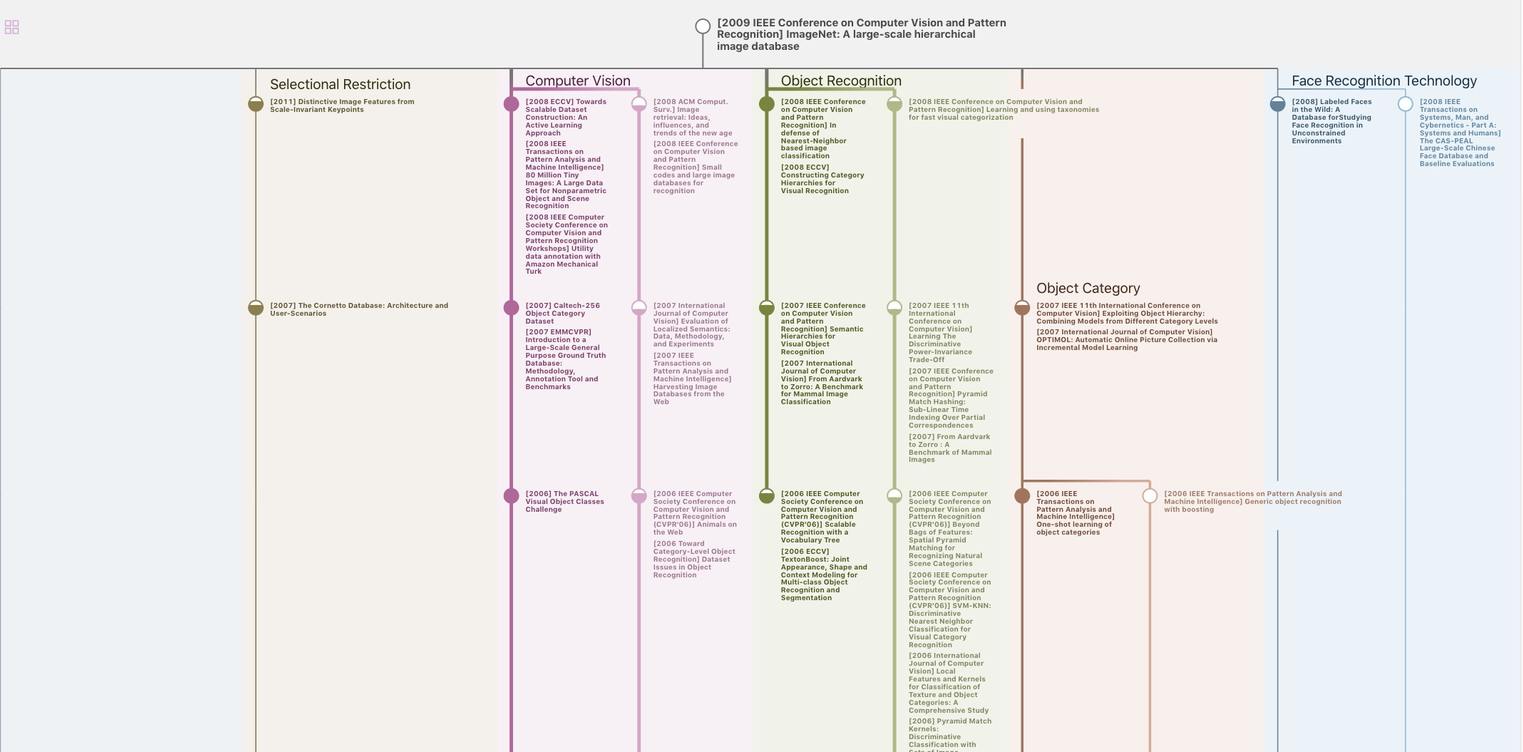
生成溯源树,研究论文发展脉络
Chat Paper
正在生成论文摘要