Exploiting the Auto-Encoder Residual Error for Intrusion Detection
2019 IEEE European Symposium on Security and Privacy Workshops (EuroS&PW)(2019)
摘要
Intrusion Detection Systems aim to address the problem of correctly identifying unforeseen network attacks. The attack detection problem has been already tackled through supervised and unsupervised machine learning approaches. While the former methods lead to models very accurate on already seen samples, the latter provide models robust on unforeseen samples by trading-off a high accuracy on seen ones. In this paper, we combine deep unsupervised neural networks with supervised neural networks aiming at improving the classification accuracy on unforeseen attacks. Auto-encoders neural networks are used both for feature engineering and as anomaly detectors. Experimental results on a challenging dataset prove the validity of the proposed approach when compared to other state-of-the-art methods.
更多查看译文
关键词
Intrusion-detection,-Autoencoding,-Supervised-and-unsupervised-deep-learning,-Classification,-Anomaly-detection
AI 理解论文
溯源树
样例
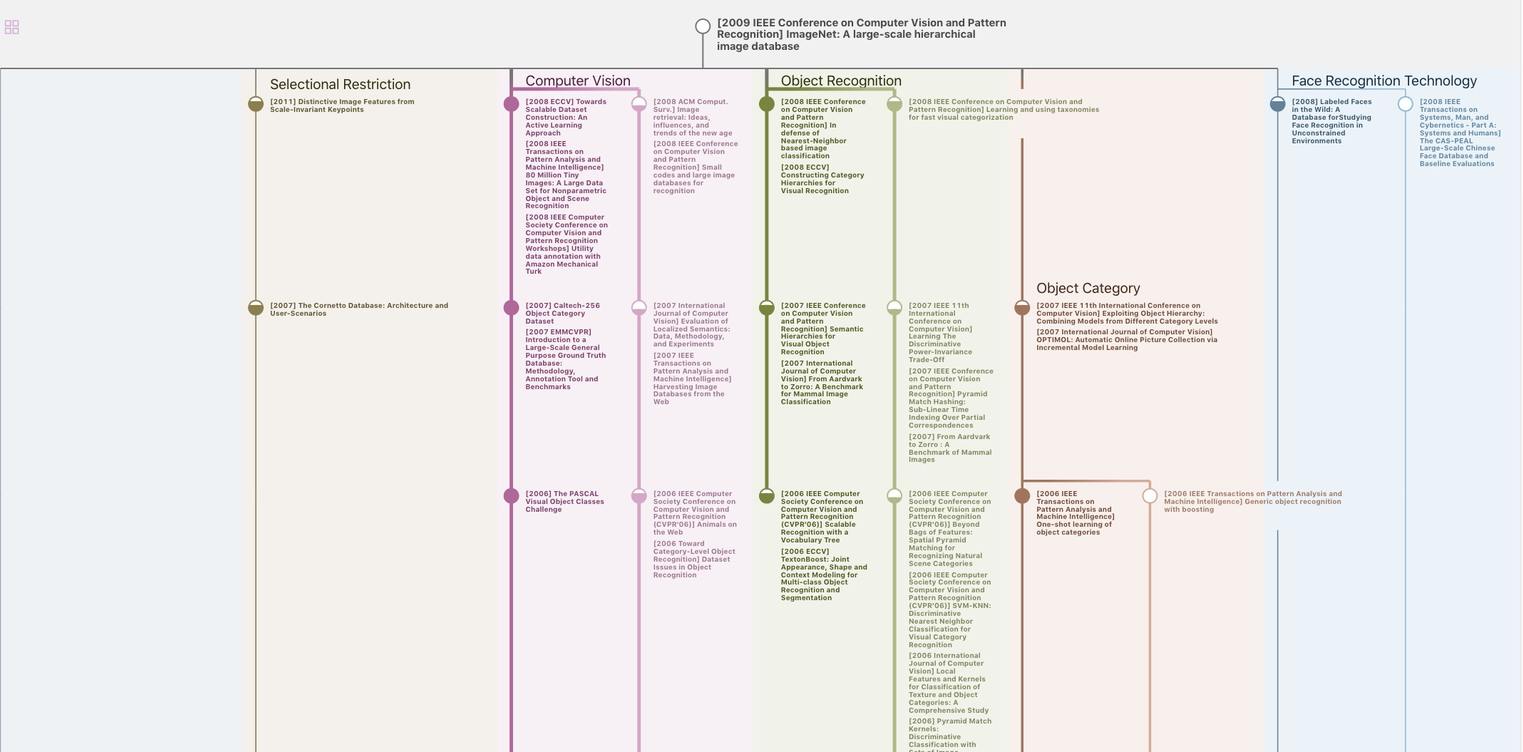
生成溯源树,研究论文发展脉络
Chat Paper
正在生成论文摘要