A Heterogeneous Domain Adversarial Neural Network for Trans-Domain Behavioral Targeting.
PAKDD(2019)
摘要
To realize trans-domain behavioral targeting, which targets interested potential users of a source domain (e.g. E-Commerce) based on their behaviors in a target domain (e.g. Ad-Network), heterogeneous transfer learning (HeTL) is a promising technique for modeling behavior linkage between domains. It is required for HeTL to learn three functionalities: representation alignment, distribution alignment, and classification. In our previous work, we prototyped and evaluated two typical transfer learning algorithms, but neither of them jointly learns the three desired functionalities. Recent advances in transfer learning include a domain-adversarial neural network (DANN), which jointly learns distribution alignment and classification. In this paper, we extended DANN to be able to learn representation alignment by simply replacing its shared encoder with domain-specific types, so that it jointly learns the three desired functionalities. We evaluated the effectiveness of the joint learning of the three functionalities using real-world data of two domains: E-Commerce, which is set as the source domain, and Ad Network, which is set as the target domain.
更多查看译文
关键词
Behavioral targeting, Heterogeneous transfer learning, Domain adversarial training
AI 理解论文
溯源树
样例
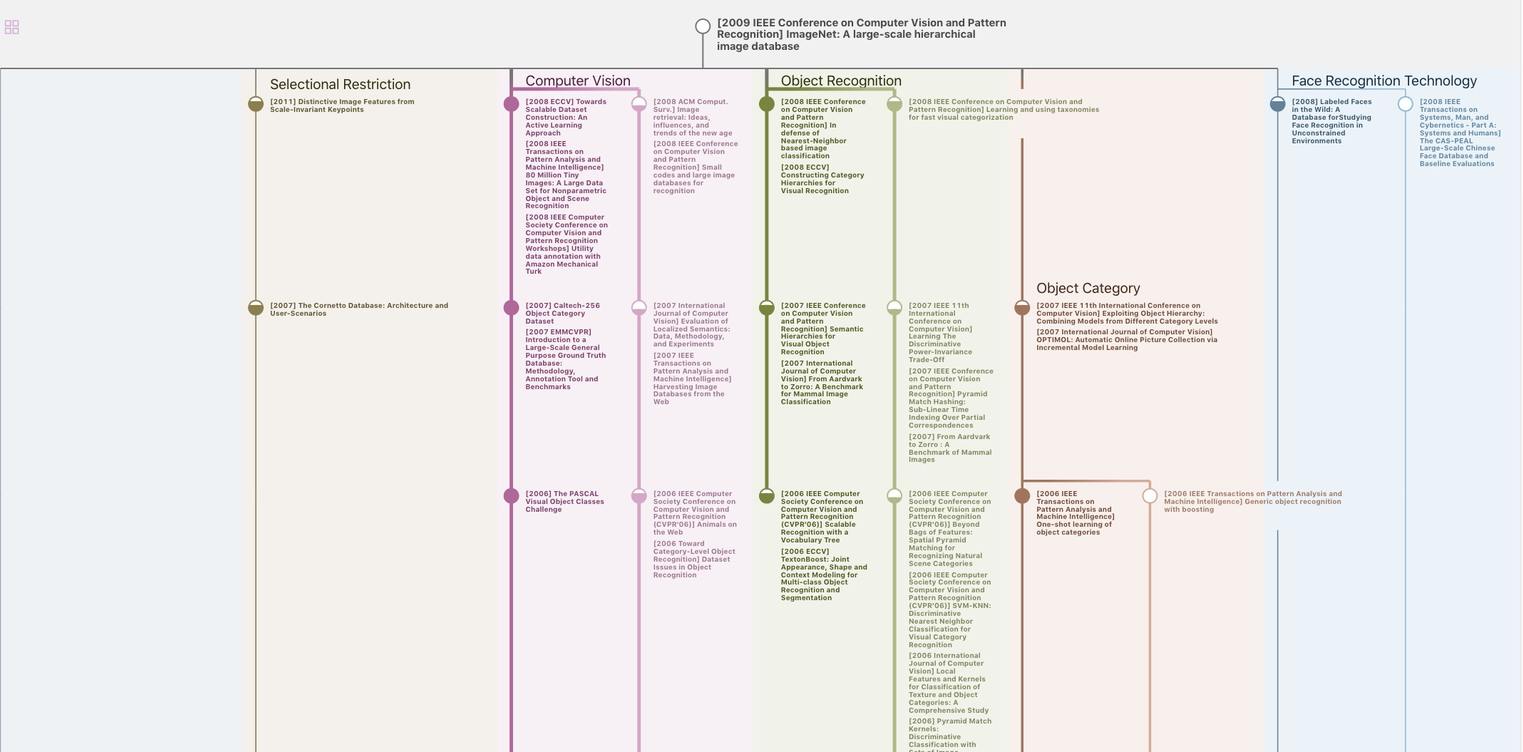
生成溯源树,研究论文发展脉络
Chat Paper
正在生成论文摘要