Time to lane change and completion prediction based on Gated Recurrent Unit Network
2019 IEEE Intelligent Vehicles Symposium (IV)(2019)
摘要
A lot of research has been done to model and predict a driver's behaviors to improve driving safety. Inferring lane change maneuver can be a critical one among them. However, the lane change prediction problem is generally treated as a classification task in which the labels represent the probability of whether the driver will make a lane change in the upcoming few seconds. In our work, we formulate this problem as a regression task. The process of lane change behavior is analyzed to build a Gated Recurrent Units (GRU) network for predicting two time points during lane change behavior: a) When the driver will shift lane. b) When the lane change will be completed. We make a comparison of Long Short Term Memory (LSTM) network and Support Vector Machine (SVM) regression performance to show that our method can give a more precise prediction time. This work can be used to improve the safety performance of driver assistance systems and help other traffic participants having a safer environment.
更多查看译文
关键词
lane change prediction problem,lane change behavior,completion prediction,gated recurrent unit network,lane change maneuver inference,driver behaviors,regression task
AI 理解论文
溯源树
样例
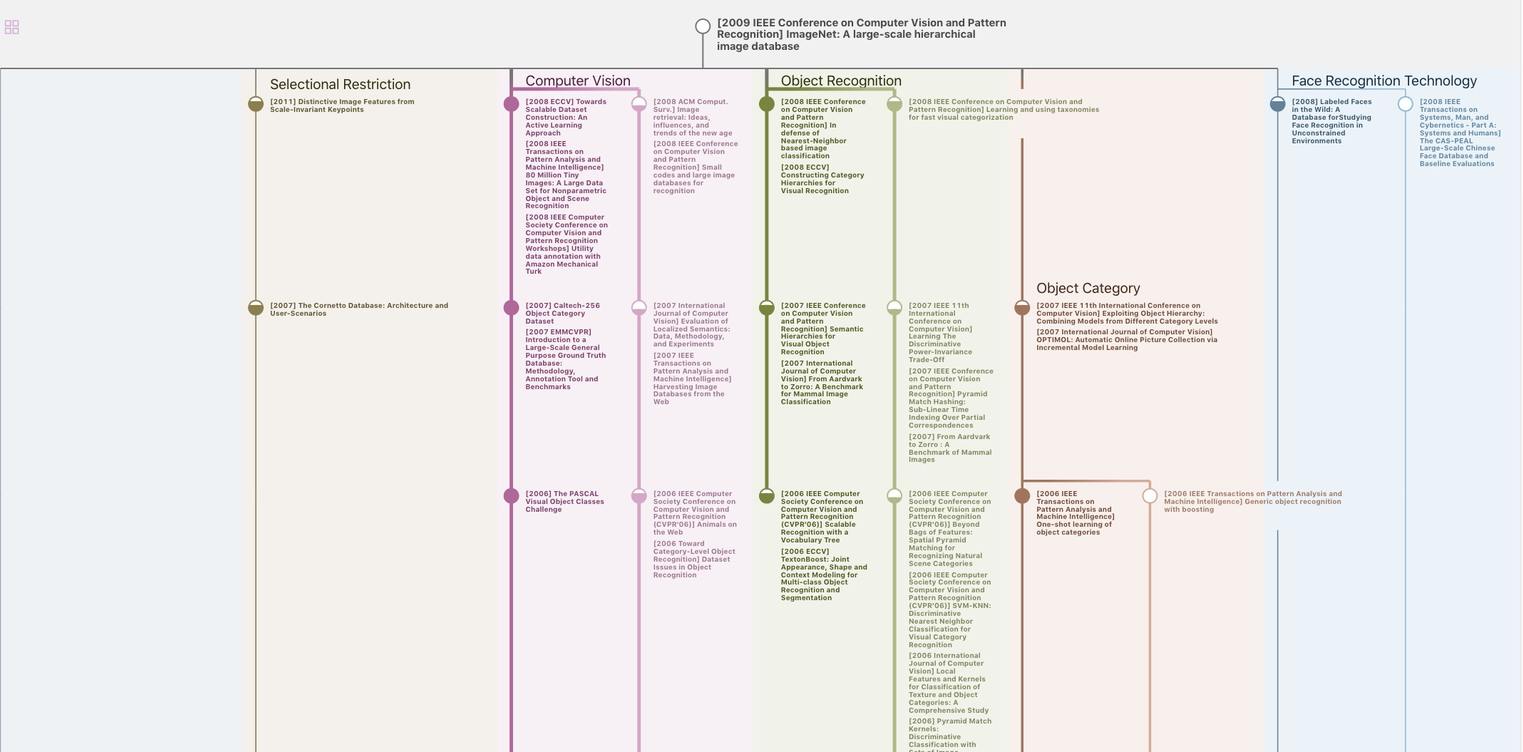
生成溯源树,研究论文发展脉络
Chat Paper
正在生成论文摘要