Vehicle Detection based on Deep Learning Heatmap Estimation
2019 IEEE Intelligent Vehicles Symposium (IV)(2019)
摘要
The detection and localization of objects on point cloud provided by LiDAR sensors have various applications, especially for autonomous driving. As methods are being more efficient in terms of performance and execution speed, they operate in an end-to-end fashion, not allowing in case of errors the evaluation of which areas could cause failures. We propose in this paper a simple method that delivers from a simple statistical voxel encoding an intermediate top view heatmap that illustrates the global state of the scene seen by the network. Completely detected vehicles are represented on this map as elliptic blobs. Difficult cases such as occluded cars may not illustrate a complete spot, however their mark may still indicate that a possible hazard, allowing planification algorithms to decide a reduction of the velocity as something may suddenly appear. Furthermore, thanks to the alternative representation of objects, detections in terms of bounding boxes can be extracted even from the intermediate map. In addition to its implementation simplicity, the proposed architecture reaches high level of performance on the KITTI detection benchmark.
更多查看译文
关键词
occluded cars,planification algorithms,KITTI detection benchmark,vehicle detection,deep learning heatmap estimation,point cloud,LiDAR sensors,autonomous driving,intermediate top view heatmap,global state,elliptic blobs,object detection,statistical voxel encoding,velocity reduction,object representation,bounding boxes
AI 理解论文
溯源树
样例
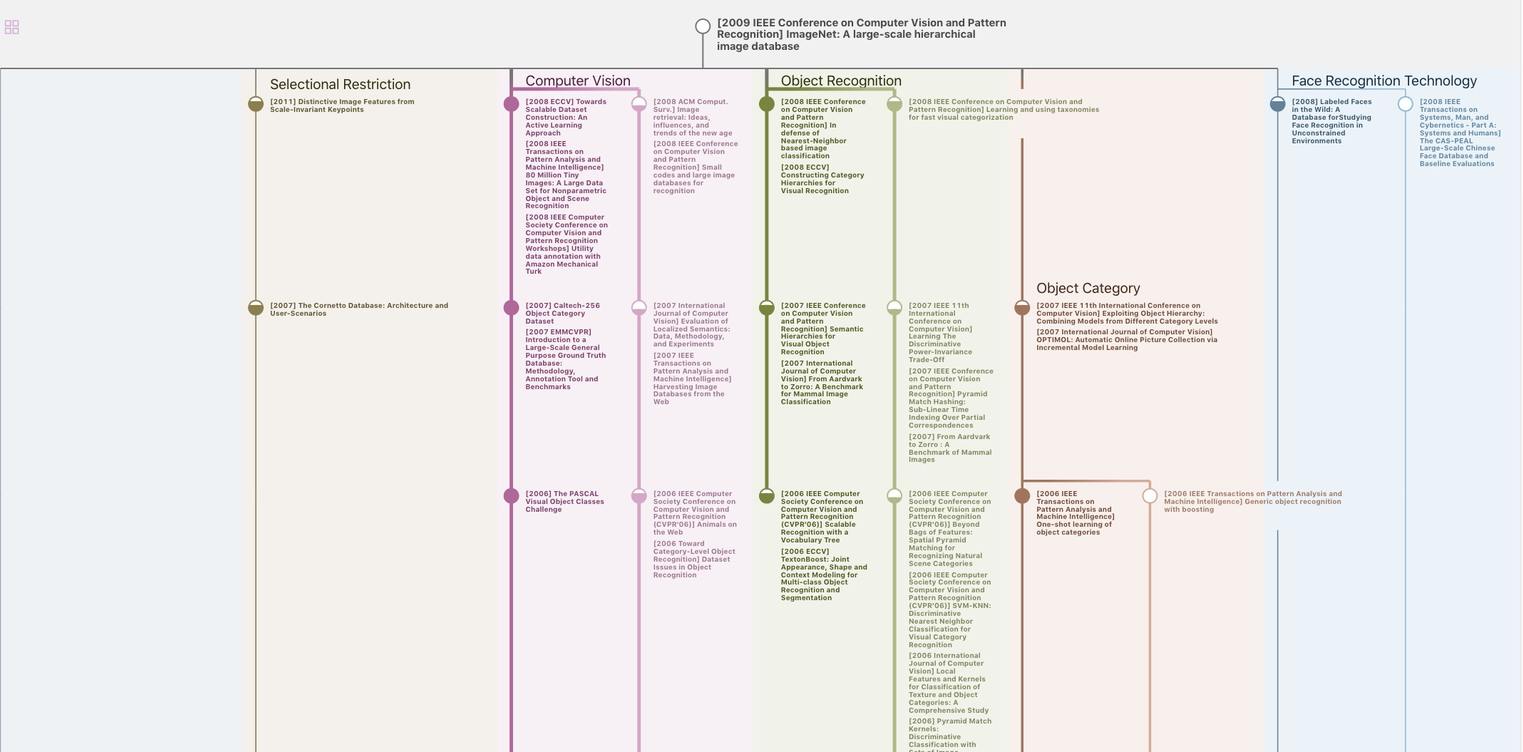
生成溯源树,研究论文发展脉络
Chat Paper
正在生成论文摘要