Predicting the broadly neutralizing antibody susceptibility of the HIV reservoir.
JCI INSIGHT(2019)
摘要
Broadly neutralizing antibodies (bNAbs) against HIV-1 are under evaluation for both prevention and therapy. HIV-1 sequence diversity observed in most HIV-infected individuals and archived variations in critical bNAb epitopes present a major challenge for the clinical application of bNAbs, as preexistent resistant viral strains can emerge, resulting in bNAb failure to control HIV. In order to identify viral resistance in patients prior to antibody therapy and to guide the selection of effective bNAb combination regimens, we developed what we believe to be a novel Bayesian machine-learning model that uses HIV-1 envelope protein sequences and foremost approximated glycan occupancy information as variables to quantitatively predict the half-maximal inhibitory concentrations (IC50) of 126 neutralizing antibodies against a variety of cross Glade viruses. We then applied this model to peripheral blood mononuclear cell-derived proviral Env sequences from 25 HIV-1-infected individuals mapping the landscape of neutralization resistance within each individual's reservoir and determined the predicted ideal bNAb combination to achieve WO% neutralization at ICSO values <1 mu g/ml. Furthermore, predicted cellular viral reservoir neutralization signatures of individuals before an analytical antiretroviral treatment interruption were consistent with the measured neutralization susceptibilities of the respective plasma rebound viruses, validating our model as a potentially novel tool to facilitate the advancement of bNAbs into the clinic.
更多查看译文
关键词
AIDS/HIV,Bioinformatics,Diagnostics,Immunotherapy,Therapeutics
AI 理解论文
溯源树
样例
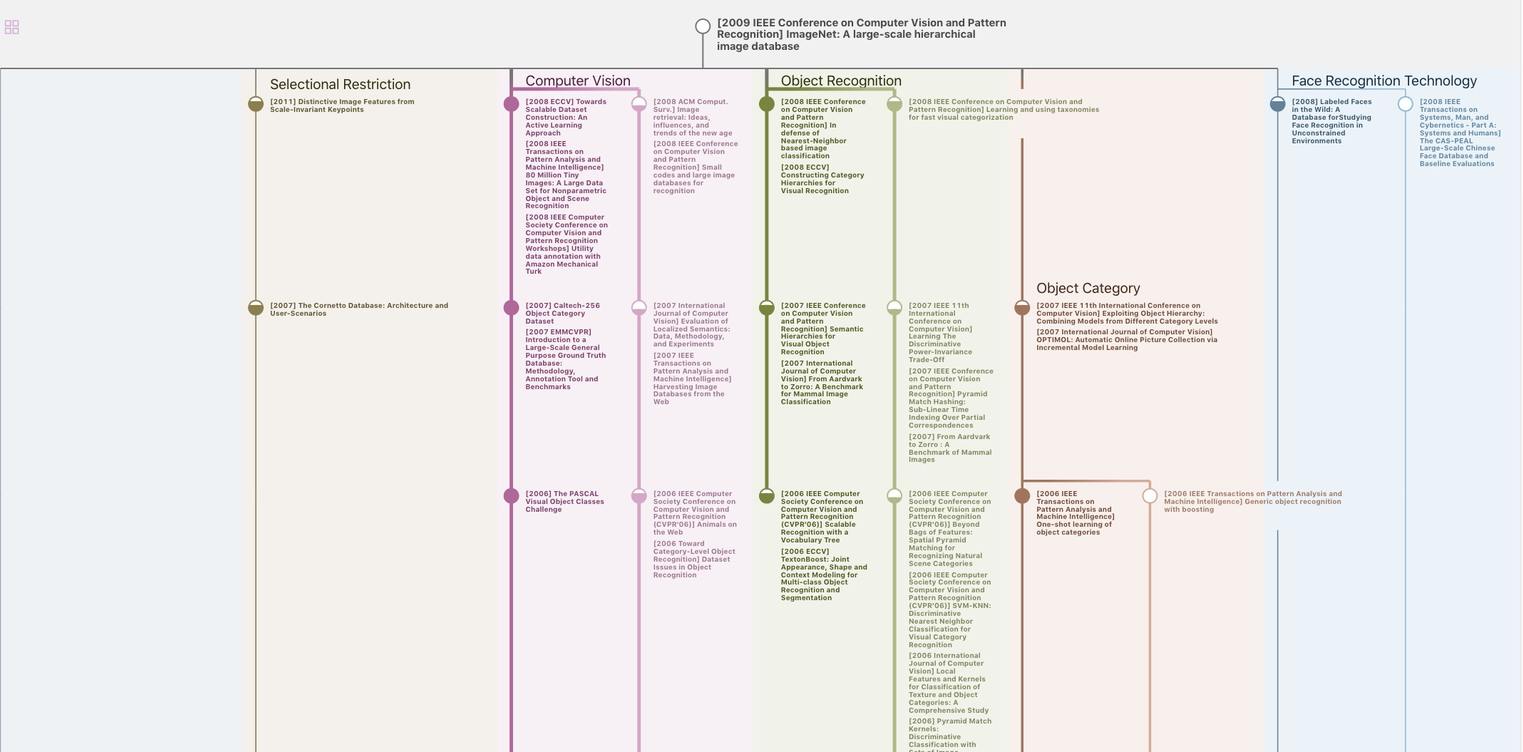
生成溯源树,研究论文发展脉络
Chat Paper
正在生成论文摘要