Low-Precision Neural Network Decoding of Polar Codes
2019 IEEE 20th International Workshop on Signal Processing Advances in Wireless Communications (SPAWC)(2019)
摘要
Neural Network (NN) polar decoders have been getting much attention as a viable replacement for conventional decoders in 5G New Radio (NR). Despite scalability issues, the NN-based decoder is a promising technology as it can improve the latency of the standard Successive Cancellation (SC) decoder. It was shown that the Neural Successive Cancellation (NSC) decoder has an improved theoretical latency compared to the standard SC decoder. However, in contrast to SC, the NSC decoder uses large floating-point weight matrices which do not fit in CPU caches, leading to higher energy usage and lower computational performance due to the increased memory traffic. Additionally such higher memory requirement would be expensive to implement in hardware and require complex floating-point arithmetic. This paper presents a new low-precision NN decoder that can replace memory-heavy NN decoders inside the NSC decoder. We show that up to 54 times weights' size reduction can be achieved with the wireless performance degradation varying between 0.1dB and 0.4dB compared to the floating-point implementation. Moreover, we show a reduction of up to respectively 438× and 555× in L1 and L2 data cache misses in our prototype software implementation.
更多查看译文
关键词
Polar codes,deep learning,weights quantization,neural network
AI 理解论文
溯源树
样例
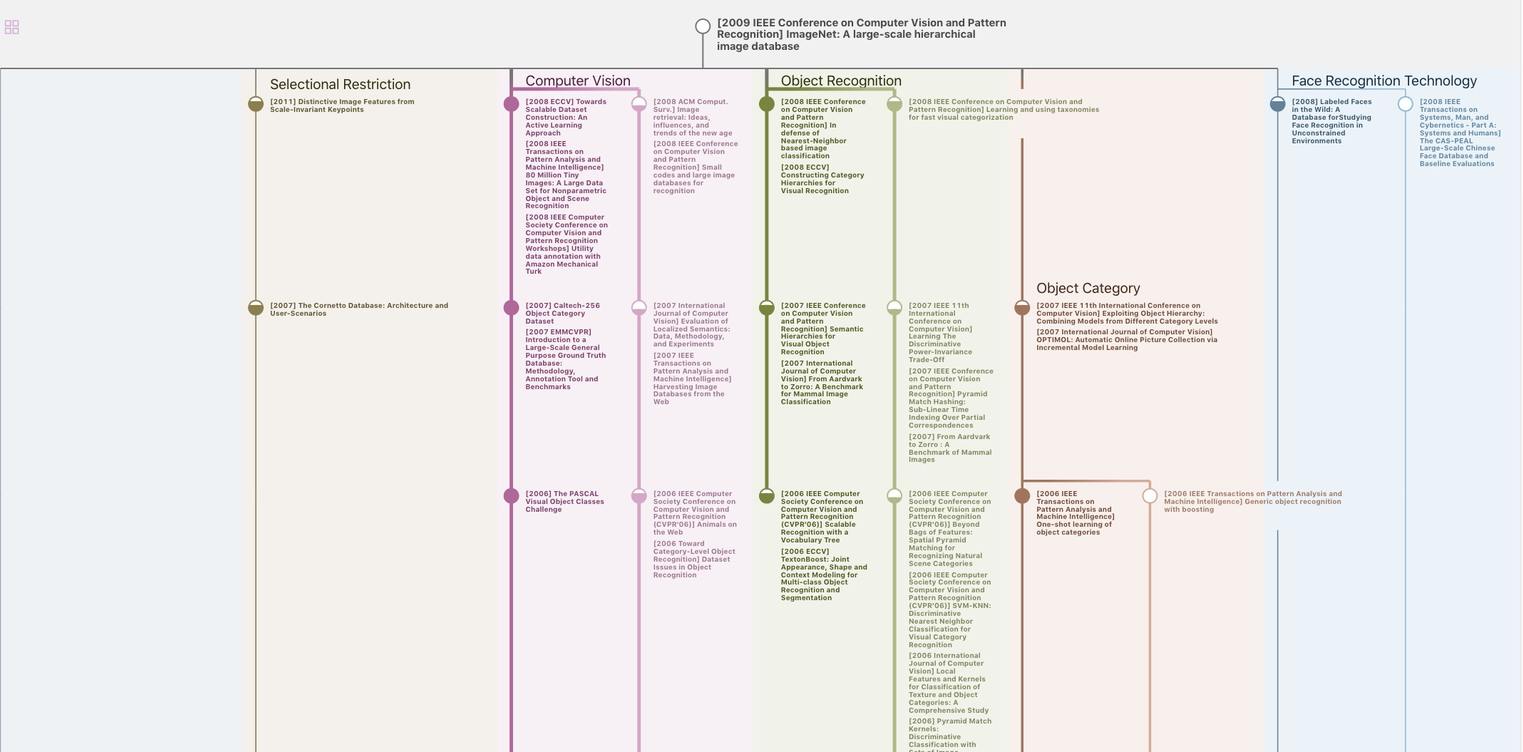
生成溯源树,研究论文发展脉络
Chat Paper
正在生成论文摘要