Remaining Useful Life Estimation by Empirical Mode Decomposition and Ensemble Deep Convolution Neural Networks
2019 IEEE International Conference on Prognostics and Health Management (ICPHM)(2019)
Abstract
Bearing remaining useful life (RUL) prediction plays a key role in guaranteeing safe operation and reducing maintenance costs. In this paper, we present a novel deep learning method for RUL estimation approach through time Empirical Mode Decomposition (EMD) and Convolutional Neural Network (CNN). EMD can reveal the nonstationary property of bearing degradation signals effectively. After acquiring time-series degradation signals, namely Intrinsic Mode Functions (IMF), we can utilize the featured information as the input of Convolution layer of models. Here, we introduce an EMD-CNN model structure, which keeps the global and local information synchronously compared to a traditional CNN. In order to get a more accurate prediction, an ensemble model with several weighting methods are proposed, where the experiment indicates an improvement of performance.
MoreTranslated text
Key words
Neural Networks,Ensemble Learning,Empirical Mode Decomposition,Remaining Useful Life
AI Read Science
Must-Reading Tree
Example
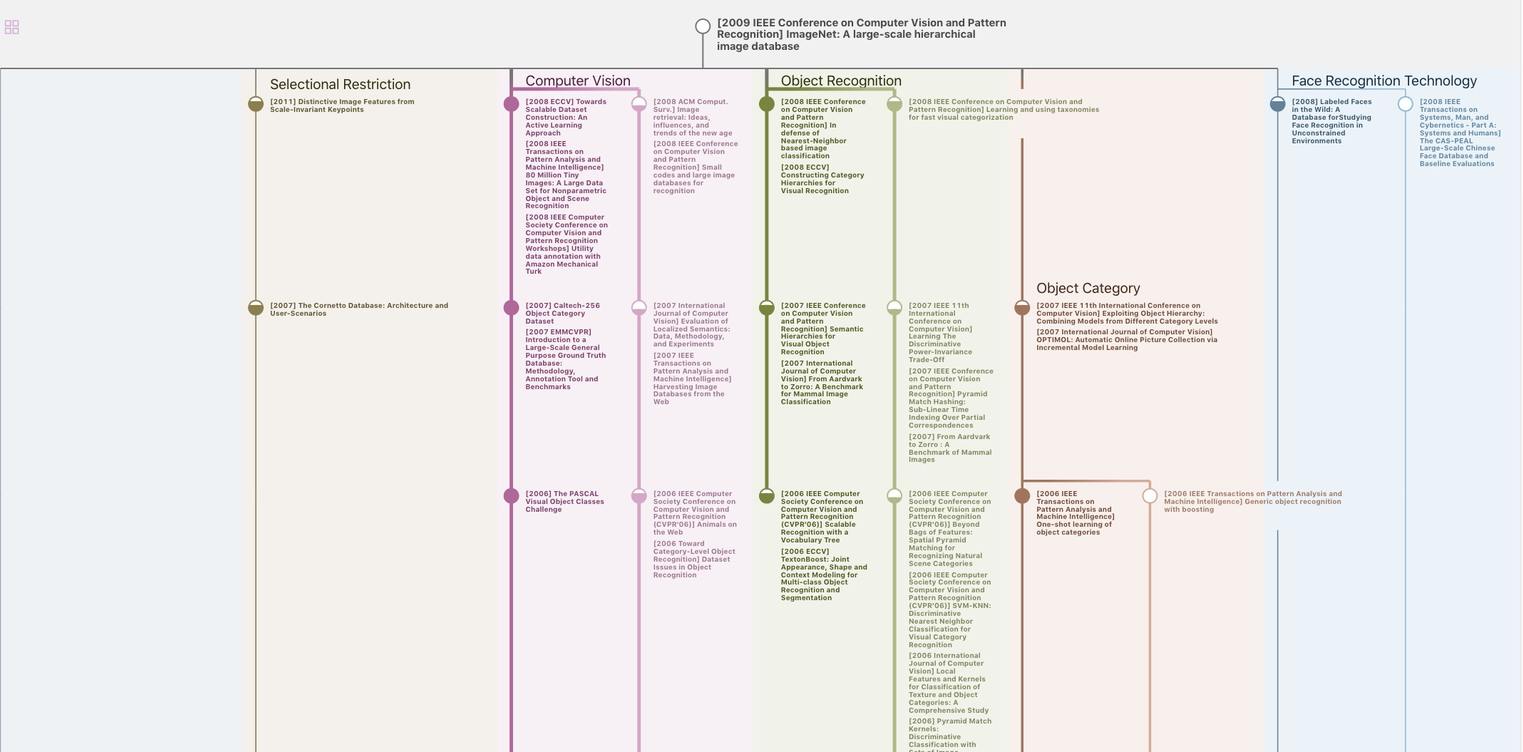
Generate MRT to find the research sequence of this paper
Chat Paper
Summary is being generated by the instructions you defined