Part Name Normalization
2019 IEEE International Conference on Prognostics and Health Management (ICPHM)(2019)
摘要
Parts information plays a key role in prognostics and health management. However, expressions of parts often have a wide range of variations, spawned by typos, ad hoc abbreviations, acronyms, and incomplete names. Normalization of such terms is crucial for many applications. Part names post a major challenge also because they tend to be in the form of multi-word terms. In this paper, we propose a novel normalization method UNAMER (Unification and Normalization Analysis, Misspelling Evaluation and Recognition). It is a general method for identifying term variants, including multi-word term variants, and normalizing them under a canonical name. UNAMER does not rely on a predefined set of canonical terms, which is often hard to obtain. Given a term, UNAMER first identifies candidate variants by exploiting contextual information. It then uses a supervised machine learning model, trained using easy-to-generate examples, that leverages both contextual and lexical features to predict actual variants from the candidates. UNAMER further extends its capability to normalize multi-word parts, such as part names like `lt pnl', `letf pnl' and `lft panal' for `left panel' using a specialized linguistically motivated term alignment approach. UNAMER has been deployed in practical applications to normalize part names in the aerospace domain. We will use examples from these real-life applications to demonstrate and illustrate results from UNAMER.
更多查看译文
关键词
part name extraction,information extraction,maintenance log analysis,text normalization,text unification
AI 理解论文
溯源树
样例
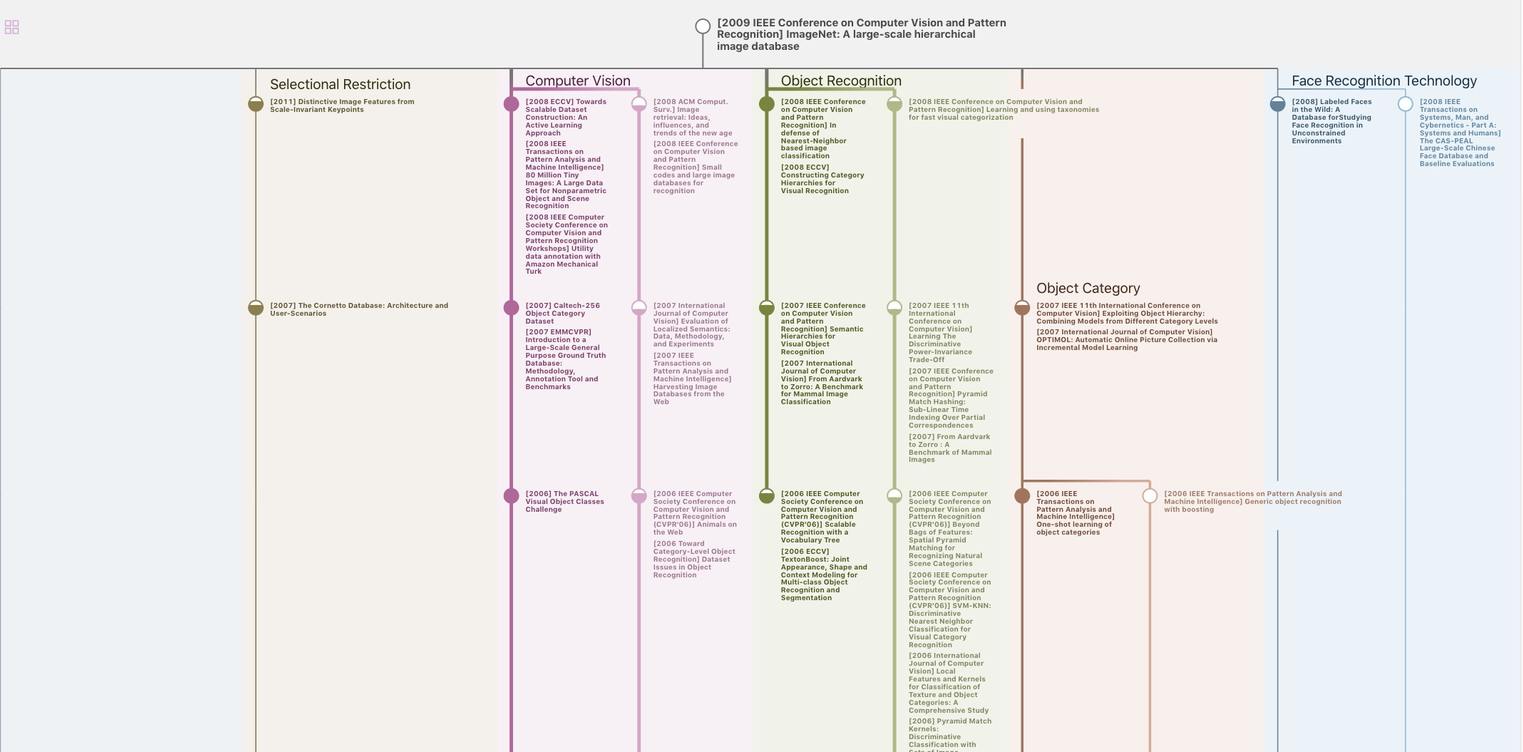
生成溯源树,研究论文发展脉络
Chat Paper
正在生成论文摘要