Inferring species interactions using Granger causality and convergent cross mapping
Theoretical Ecology(2020)
摘要
Identifying directed interactions between species from time series of their population densities has many uses in ecology. This key statistical task is equivalent to causal time series inference, which connects to the Granger causality (GC) concept: x causes y if x improves the prediction of y in a dynamic model. However, the entangled nature of nonlinear ecological systems has led to question the appropriateness of Granger causality, especially in its classical linear multivariate autoregressive (MAR) model form. Convergent cross mapping (CCM), a nonparametric method developed for deterministic dynamical systems, has been suggested as an alternative. Here, we show that linear GC and CCM are able to uncover interactions with surprisingly similar performance, for predator-prey cycles, 2-species deterministic (chaotic), or stochastic competition, as well as 10- and 20-species interaction networks. We found no correspondence between the degree of nonlinearity of the dynamics and which method performs best. Our results therefore imply that Granger causality, even in its linear MAR( p ) formulation, is a valid method for inferring interactions in nonlinear ecological networks; using GC or CCM (or both) can instead be decided based on the aims and specifics of the analysis.
更多查看译文
关键词
Time series, Interaction network, Causal inference, Feedback, Food web, Community dynamics
AI 理解论文
溯源树
样例
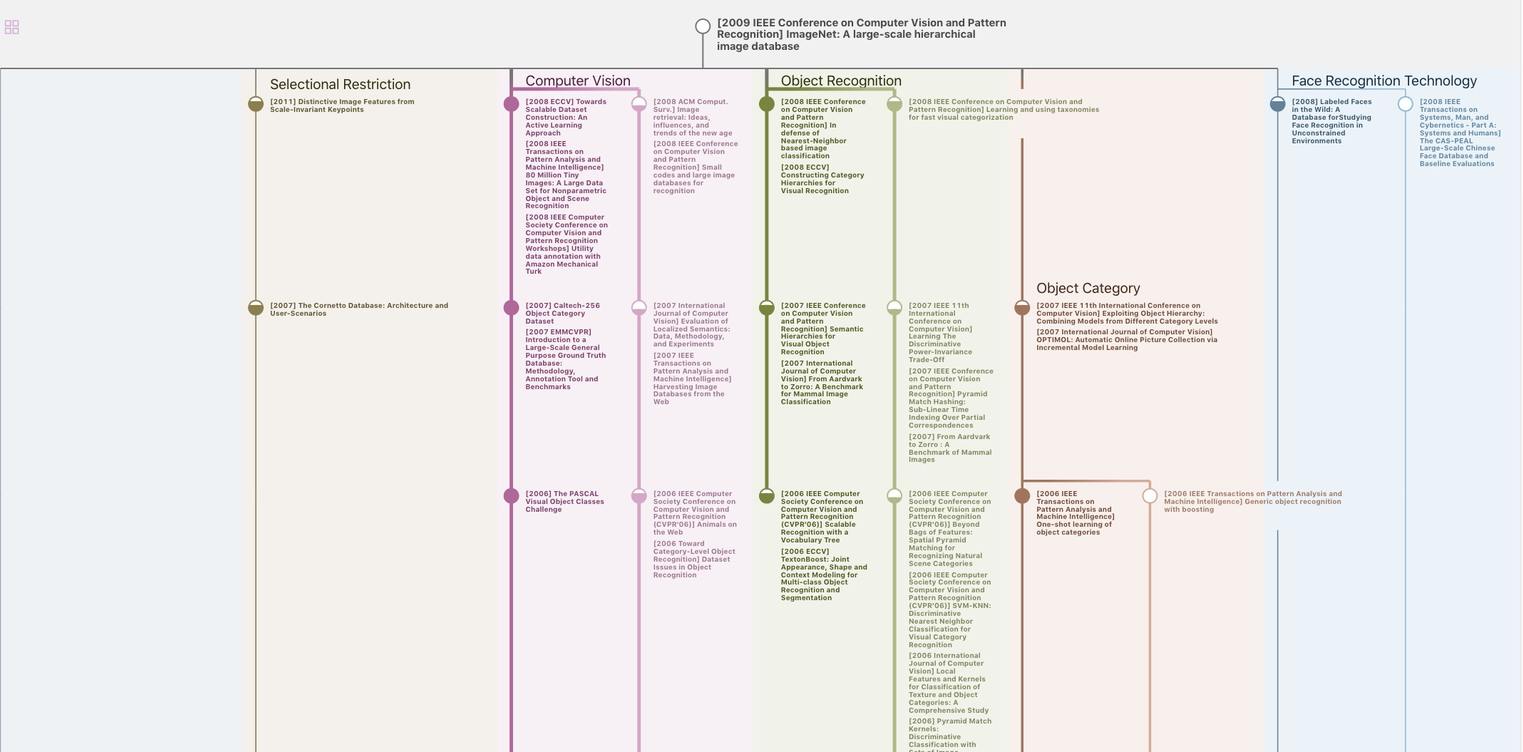
生成溯源树,研究论文发展脉络
Chat Paper
正在生成论文摘要