Near-optimal Approximate Discrete and Continuous Submodular Function Minimization.
PROCEEDINGS OF THE THIRTY-FIRST ANNUAL ACM-SIAM SYMPOSIUM ON DISCRETE ALGORITHMS (SODA'20)(2020)
摘要
In this paper we provide improved running times and oracle complexities for approximately minimizing a submodular function. Our main result is a randomized algorithm, which given any submodular function defined on n-elements with range [−1, 1], computes an ε-additive approximate minimizer in Õ(n/ε2) oracle evaluations with high probability. This improves over the Õ(n5/3/ε2) oracle evaluation algorithm of Chakrabarty et al. (STOC 2017) and the Õ(n3/2/ε2) oracle evaluation algorithm of Hamoudi et al..
Further, we leverage a generalization of this result to obtain efficient algorithms for minimizing a broad class of nonconvex functions. For any function f with domain [0, 1]n that satisfies [MATH HERE] for all i ≠ j and is L-Lipschitz with respect to the L∞-norm we give an algorithm that computes an ε-additive approximate minimizer with Õ(n · poly(L/ε)) function evaluation with high probability.
更多查看译文
AI 理解论文
溯源树
样例
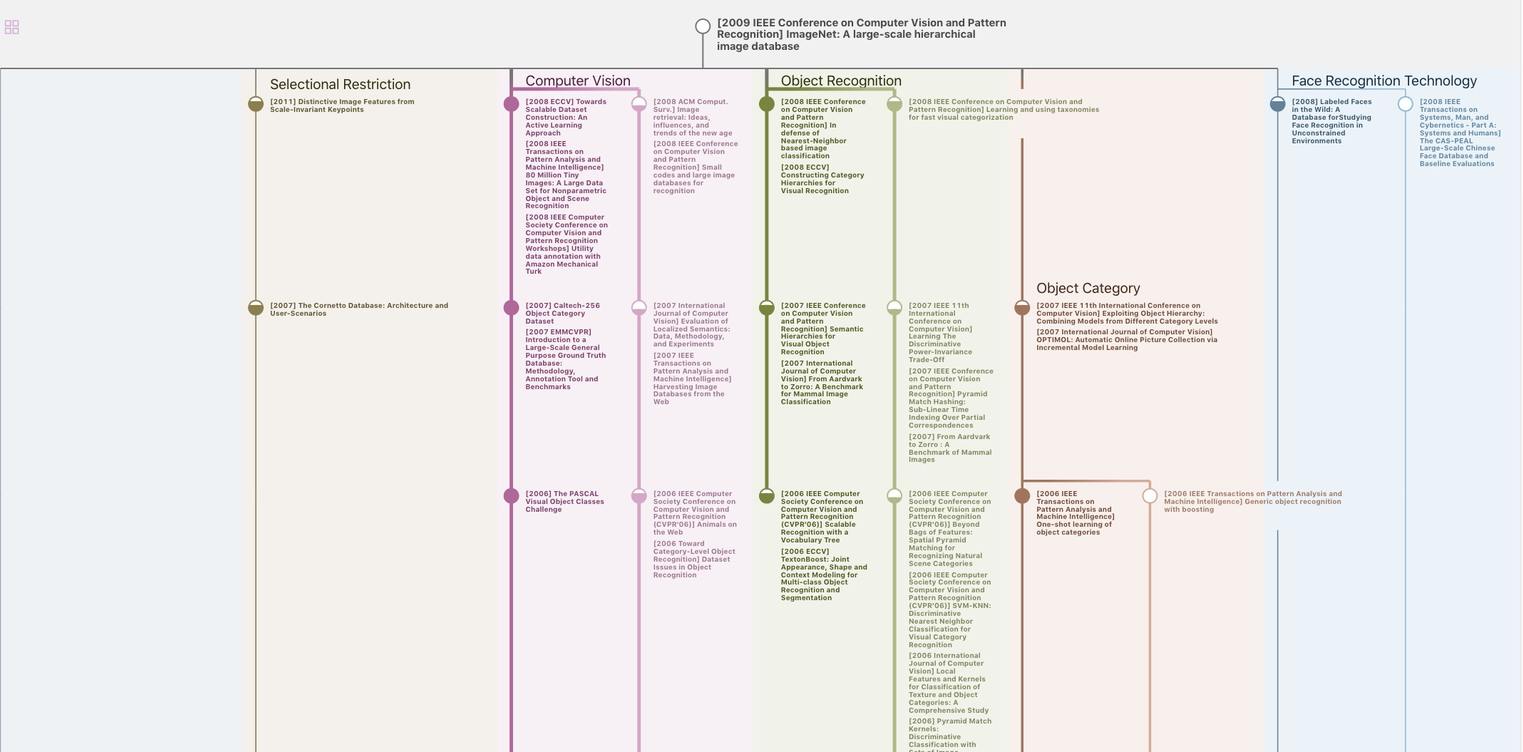
生成溯源树,研究论文发展脉络
Chat Paper
正在生成论文摘要