Learning in Online Advertising
Marketing science(2019)
Abstract
Prior literature on pay-per-click advertising assumes that publishers know advertisers' click-through rates (CTR). This information, however, is not available when a new advertiser first joins a publisher. The new advertiser's CTR can be learned only if its ad is shown to enough consumers, i.e., the advertiser wins enough auctions. Since publishers use CTRs to calculate payments and allocations, the lack of information about a new advertiser can affect the advertisers' bids. Using a game theory model, we analyze advertisers' strategies, their payoffs, and the publisher's revenue in a learning environment. Our results indicate that a new advertiser always bids higher (sometimes above valuation) in the beginning. The incumbent advertiser's strategy depends on its valuation and CTR. A strong incumbent increases its bid to deter the publisher from learning the new advertiser's CTR, whereas a weak incumbent decreases its bid to facilitate learning. Interestingly, the publisher may benefit from not knowing the new advertiser's CTR because its ignorance could induce advertisers to bid more aggressively. Nonetheless, the publisher's revenue sometimes decreases because of this lack of information. The publisher can mitigate this loss by lowering the reserve price of, offering advertising credit to, or boosting the bids of new advertisers.
MoreTranslated text
Key words
game theory,learning,competitive analysis,bidding strategy,online advertising,mechanism design,exploration-exploitation
AI Read Science
Must-Reading Tree
Example
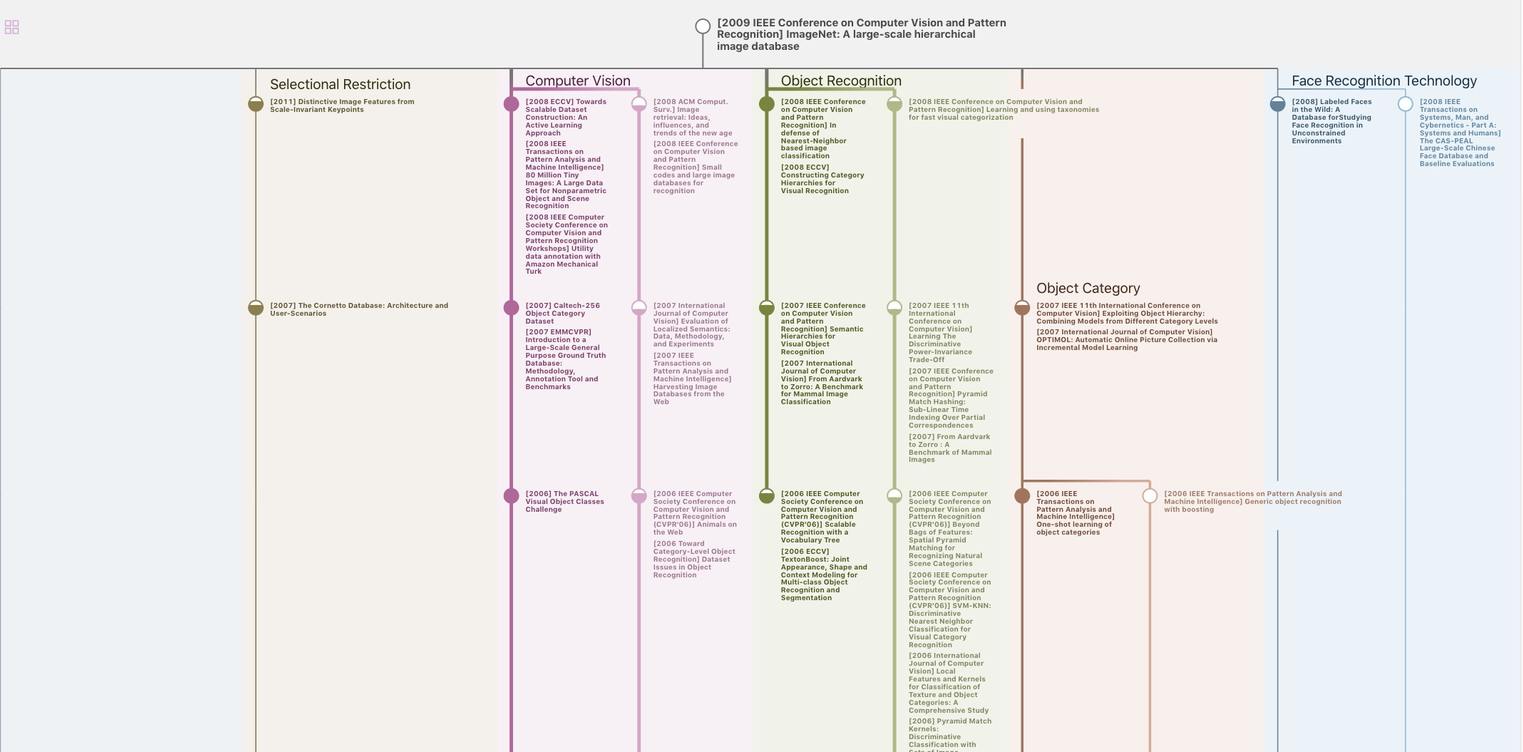
Generate MRT to find the research sequence of this paper
Chat Paper
Summary is being generated by the instructions you defined