Real-Time Activity And Fall Risk Detection For Aging Population Using Deep Learning
2018 9TH IEEE ANNUAL UBIQUITOUS COMPUTING, ELECTRONICS & MOBILE COMMUNICATION CONFERENCE (UEMCON)(2018)
Abstract
Each year, 2.8 million older people are treated in emergency departments for fall injuries, according to CDC (Centers for Disease Control and Prevention). And human activities including fall also relate to many severe issues such as heart attack, stroke, and mobility disorders. Driven by this society issue, in this paper, we propose a new multi-view deep learning framework, to enable cellphone-based real-time activity and fall risk detection for aging population. More specially, we apply a deep convolutional neural network to automatically unveil deeply-hidden non-linear patterns from raw 3-axis accelerometer streams; we treat the multiple time series data as multi-view 1D images, which are then fed into the convolutional network; we also demonstrate that the proposed framework can robustly detect human activities and fall risks on a challenging dataset, which includes diverse daily activities, user behaviors and a broad age range to cover elderly people over 50. Experimental results show a detection rate of 91.5%, based on a very challenging dataset 11,770 activities, 17 types of activities, 30 subjects, a wide range of ages (over and under 50), and diverse user behaviors. This research is expected to contribute to cellphone-based activity and fall detection for the aging population, in the era of pervasive smart health monitoring and management.
MoreTranslated text
Key words
Smart Health, Aging Population, Fall Detection, Activity Monitoring Deep Learning, Convolutional Neural Network
AI Read Science
Must-Reading Tree
Example
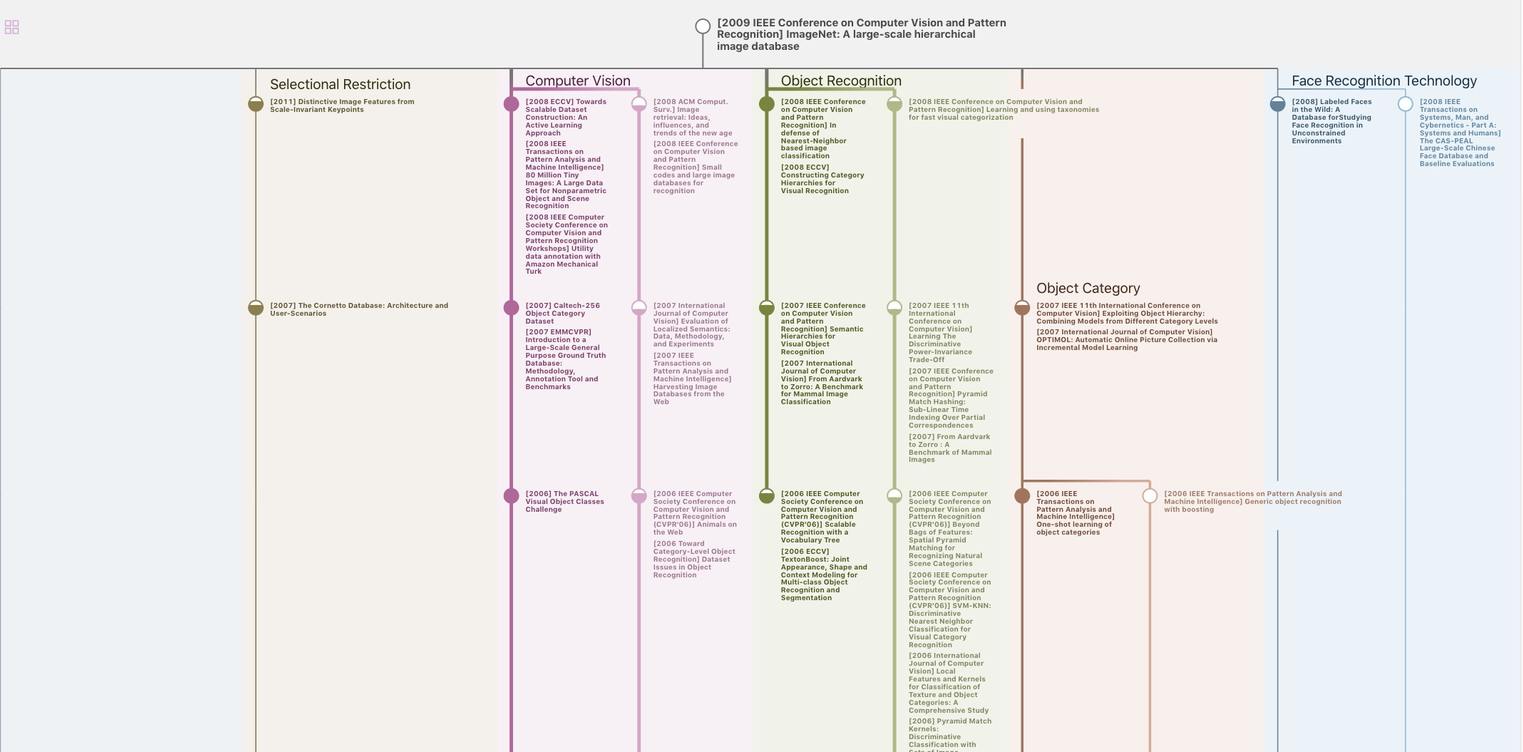
Generate MRT to find the research sequence of this paper
Chat Paper
Summary is being generated by the instructions you defined