Stochastic Approximation Algorithm with Randomization at the Input for Unsupervised Parameters Estimation of Gaussian Mixture Model with Sparse Parameters
Automation and Remote Control(2019)
Abstract
We consider the possibilities of using stochastic approximation algorithms with randomization on the input under unknown but bounded interference in studying the clustering of data generated by a mixture of Gaussian distributions. The proposed algorithm, which is robust to external disturbances, allows us to process the data “on the fly” and has a high convergence rate. The operation of the algorithm is illustrated by examples of its use for clustering in various difficult conditions.
MoreTranslated text
Key words
clustering, unsupervised learning, randomization, stochastic approximation, Gaussian mixture model
AI Read Science
Must-Reading Tree
Example
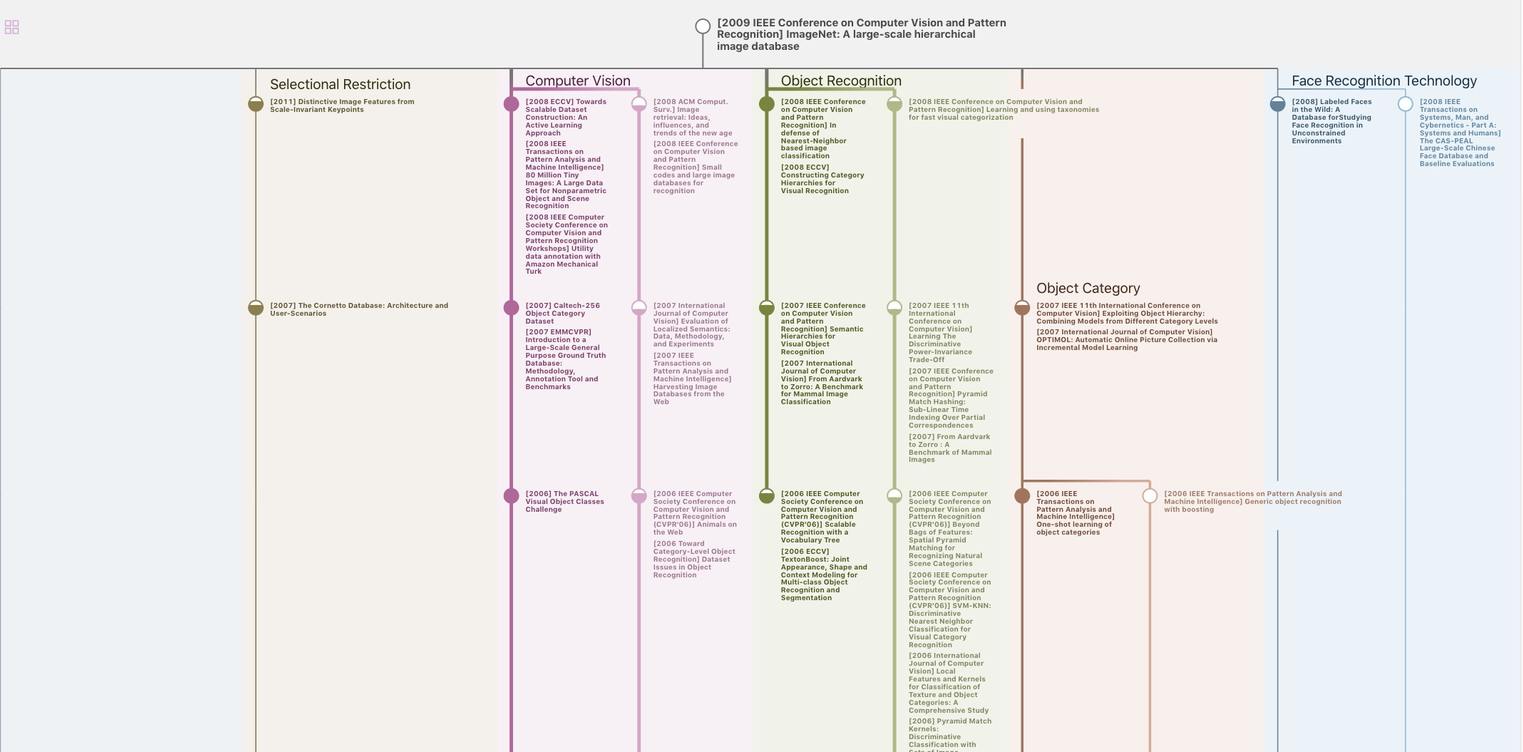
Generate MRT to find the research sequence of this paper
Chat Paper
Summary is being generated by the instructions you defined