Applying Machine Learning Optimization Methods To The Production Of A Quantum Gas
MACHINE LEARNING-SCIENCE AND TECHNOLOGY(2020)
摘要
We apply three machine learning strategies to optimize the atomic cooling processes utilized in the production of a Bose-Einstein condensate (BEC). For the first time, we optimize both laser cooling and evaporative cooling mechanisms simultaneously. We present the results of an evolutionary optimization method (differential evolution), a method based on non-parametric inference (Gaussian process regression) and a gradient-based function approximator (artificial neural network). Online optimization is performed using no prior knowledge of the apparatus, and the learner succeeds in creating a BEC from completely randomized initial parameters. Optimizing these cooling processes results in a factor of four increase in BEC atom number compared to our manually-optimized parameters. This automated approach can maintain close-to-optimal performance in long-term operation. Furthermore, we show that machine learning techniques can be used to identify the main sources of instability within the apparatus.
更多查看译文
关键词
ultracold quantum matter, machine learning, artificial neural networks, Bose-Einstein condensates
AI 理解论文
溯源树
样例
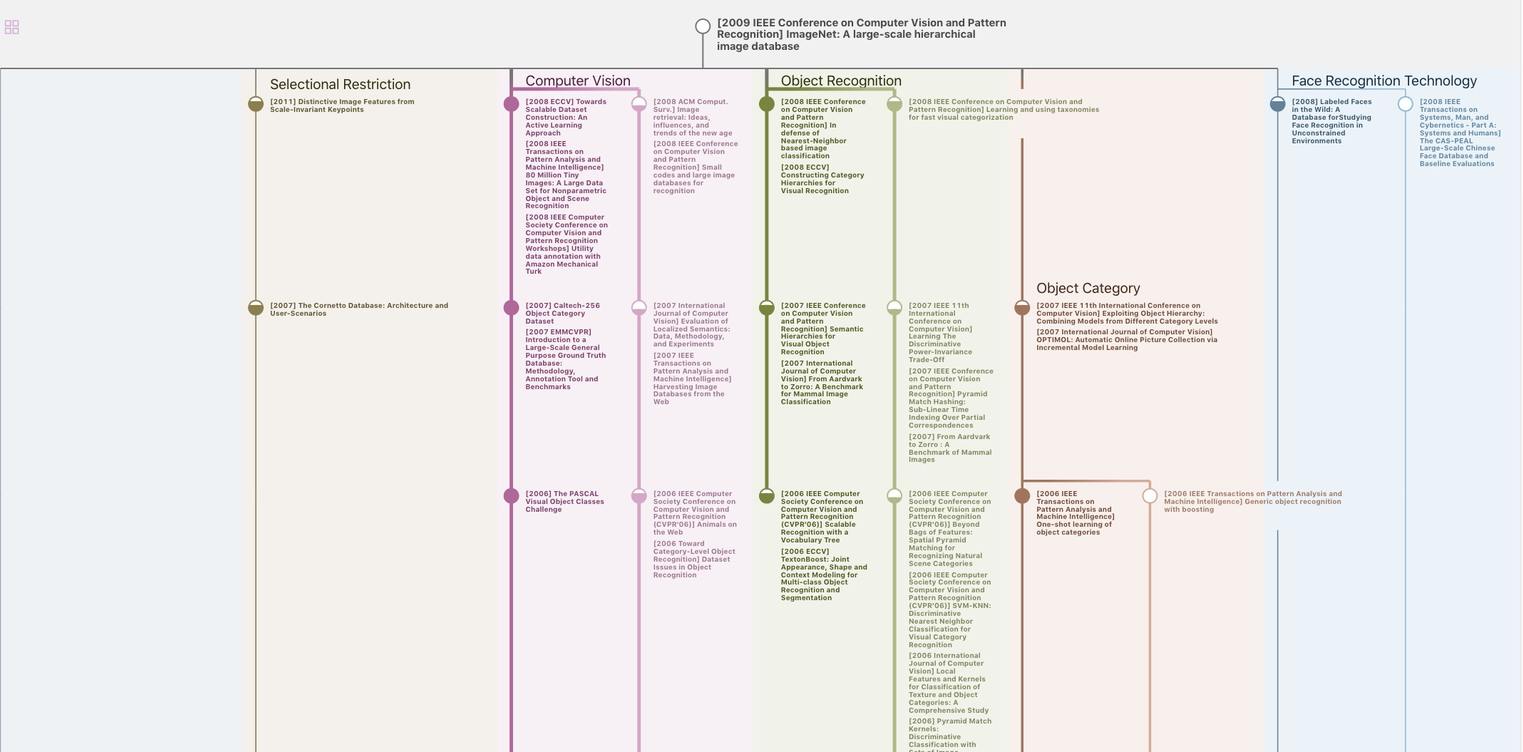
生成溯源树,研究论文发展脉络
Chat Paper
正在生成论文摘要