P2L: Predicting Transfer Learning for Images and Semantic Relations
computer vision and pattern recognition(2020)
Abstract
We describe an efficient method to accurately estimate the effectiveness of a previously trained deep learning model for use in a new learning task. We use this method, "Predict To Learn" (P2L), to predict the most likely "source" dataset to produce effective transfer for training on a "target" dataset. We validate our approach extensively across 21 tasks, including image classification tasks and semantic relationship prediction tasks in the linguistic domain. The P2L approach selects the best transfer learning model on 62% of the tasks, compared with a baseline of 48% of cases when using a heuristic of selecting the largest source dataset and 52% of cases when using a distance measure between source and target datasets. Further, our work results in an 8% reduction in error rate. Finally, we also show that a model trained from merging multiple source model datasets does not necessarily result in improved transfer learning. This suggests that performance of the target model depends upon the relative composition of the source dataset as well as their absolute scale, as measured by our novel method we term `P2L'.
MoreTranslated text
Key words
P2L,trained deep learning model,learning task,effective transfer,target dataset,image classification tasks,semantic relationship prediction tasks,linguistic domain,transfer learning model,largest source dataset,target datasets,multiple source model datasets,improved transfer learning,transfer learning prediction
AI Read Science
Must-Reading Tree
Example
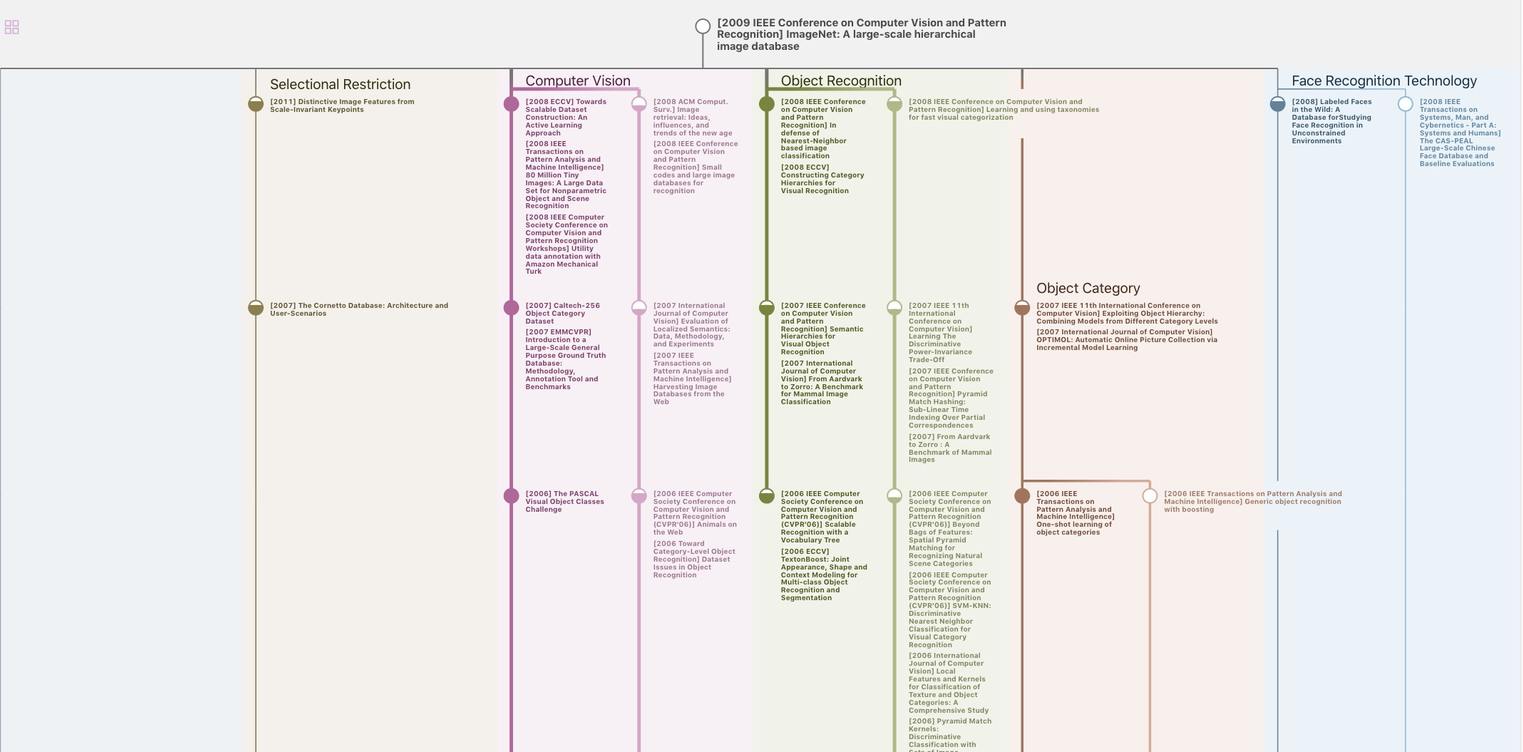
Generate MRT to find the research sequence of this paper
Chat Paper
Summary is being generated by the instructions you defined