Density estimation in representation space to predict model uncertainty
arxiv(2019)
摘要
Deep learning models frequently make incorrect predictions with high confidence when presented with test examples that are not well represented in their training dataset. We propose a novel and straightforward approach to estimate prediction uncertainty in a pre-trained neural network model. Our method estimates the training data density in representation space for a novel input. A neural network model then uses this information to determine whether we expect the pre-trained model to make a correct prediction. This uncertainty model is trained by predicting in-distribution errors, but can detect out-of-distribution data without having seen any such example. We test our method for a state-of-the art image classification model in the settings of both in-distribution uncertainty estimation as well as out-of-distribution detection. We compare our method to several baselines and set the state-of-the art for out-of-distribution detection in the Imagenet dataset.
更多查看译文
关键词
model uncertainty,representation space,estimation
AI 理解论文
溯源树
样例
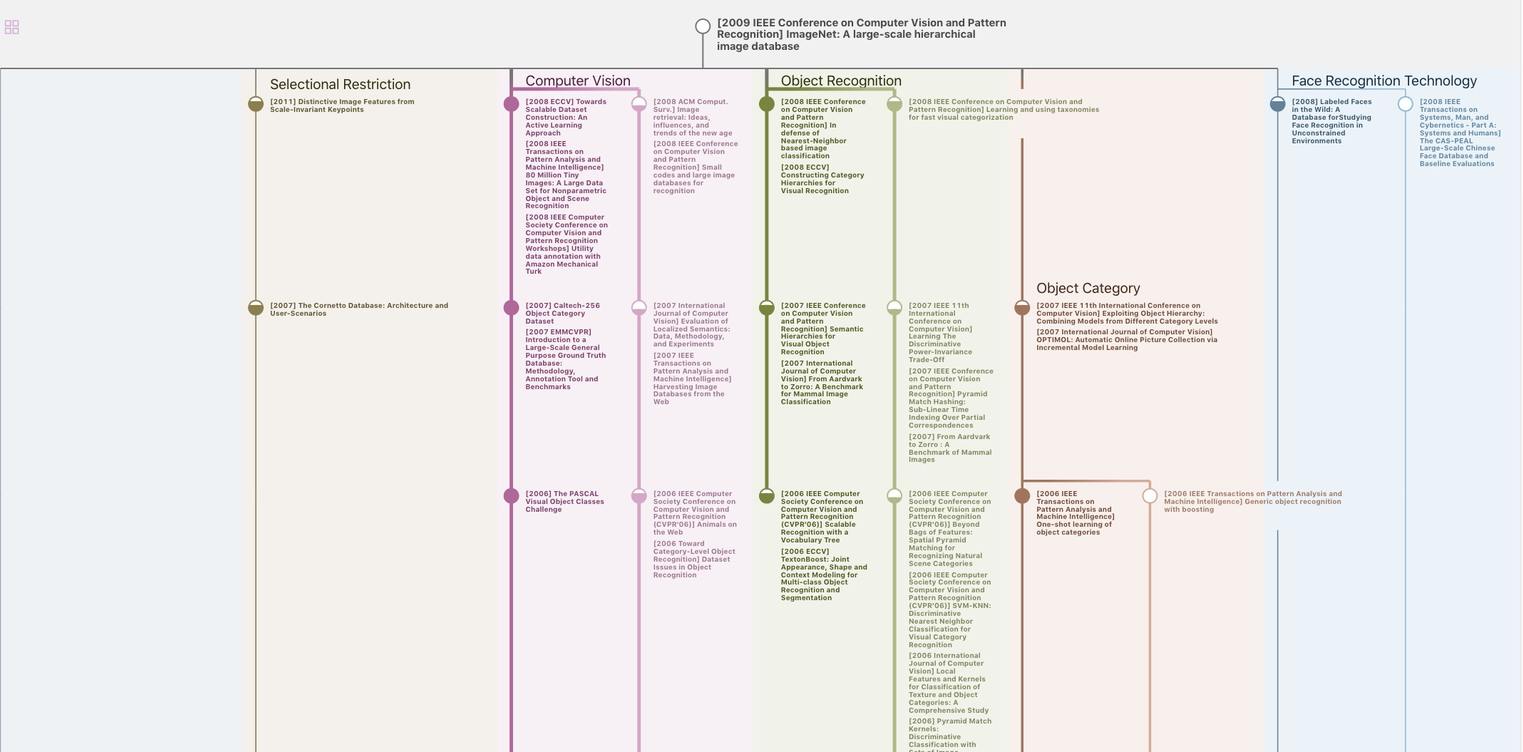
生成溯源树,研究论文发展脉络
Chat Paper
正在生成论文摘要