Error analysis of distributed least squares ranking.
Neurocomputing(2019)
摘要
Learning theory of distributed kernel methods has attracted much attentions recently. However, the existing theory analysis is limited to the kernel regression with pointwise losses. It is not clear whether theory guarantees can be obtained for distributed kernel methods with pairwise losses. To answer this question, this paper considers a new pairwise ranking algorithm, called distributed regularized least squares ranking (DRLSRank), under the divide and conquer strategy. Rather than minimizing the empirical pairwise risk associated the whole data, the proposed DRLSRank obtains the individual ranking functions based on data subsets and takes their weighted average as a final predictor. Theoretically, we derive the generalization bounds in expectation via integral operator approximation technique. Our results show that DRLSRank can achieve a satisfactory learning rate and the additional unlabeled data are crucial for relaxing the restriction on the number of data subsets, which fills the theoretical gap on learning theory for distributed pairwise ranking.
更多查看译文
关键词
Generalization error,Ranking,Divide and conquer,Reproducing kernel Hilbert space,Integral operator
AI 理解论文
溯源树
样例
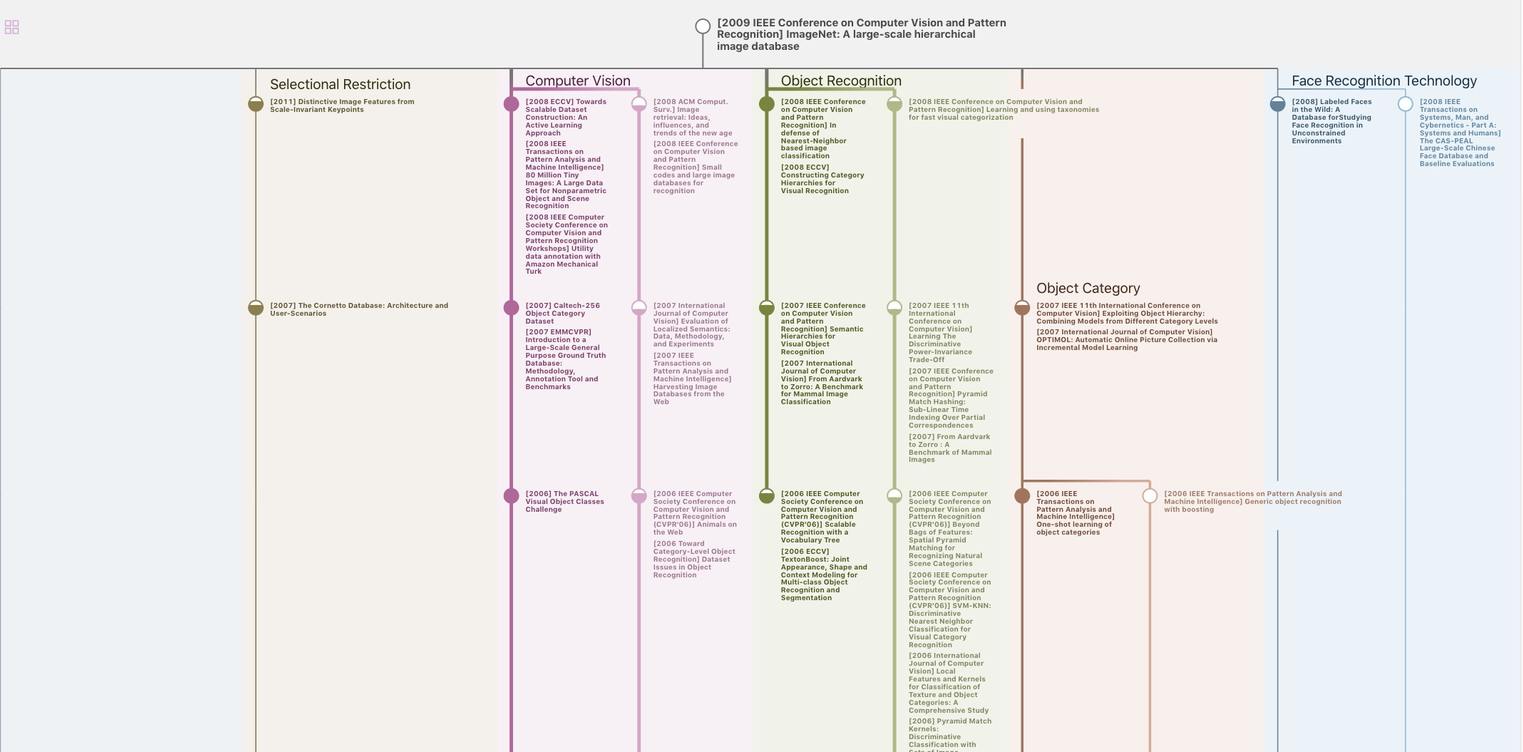
生成溯源树,研究论文发展脉络
Chat Paper
正在生成论文摘要