Modified fuzzy clustering with segregated cluster centroids.
Neurocomputing(2019)
摘要
In this paper, we firstly propose a novel strategy to fuse k-means and fuzzy c-means objective functions from a multi-objective problem into a unified model, such that extreme difficulty of choosing the optimal level of the cluster fuzziness can be avoided (level of the cluster fuzziness vanishes). Accordingly, fused k-means and fuzzy c-means clustering (FKMFCM) problem is achieved with a brand new form. Based on the proposed FKMFCM, novel degrees of membership could be further derived with the closed forms. Additionally, a penalty problem is further introduced to the proposed FKMFCM, such that the fuzzy cluster centroids are modified to be more segregated from each other. Consequently, FKMFCM with the modified cluster centroid (FKMFCM-MCC) problem is represented as a bi-objective optimization for the efficient clustering. To address the proposed FKMFCM-MCC problem, an original characteristic function is introduced, such that the corresponding algorithm converges to the global optimum of the proposed FKMFCM-MCC problem. Eventually, theoretical analysis along with the empirical result is provided to validate the effectiveness of the proposed FKMFCM-MCC approach.
更多查看译文
关键词
Fuzzy c-means,K-means,Level of fuzziness,Modified cluster centroid,Classifier fusion,Fuzzy clustering
AI 理解论文
溯源树
样例
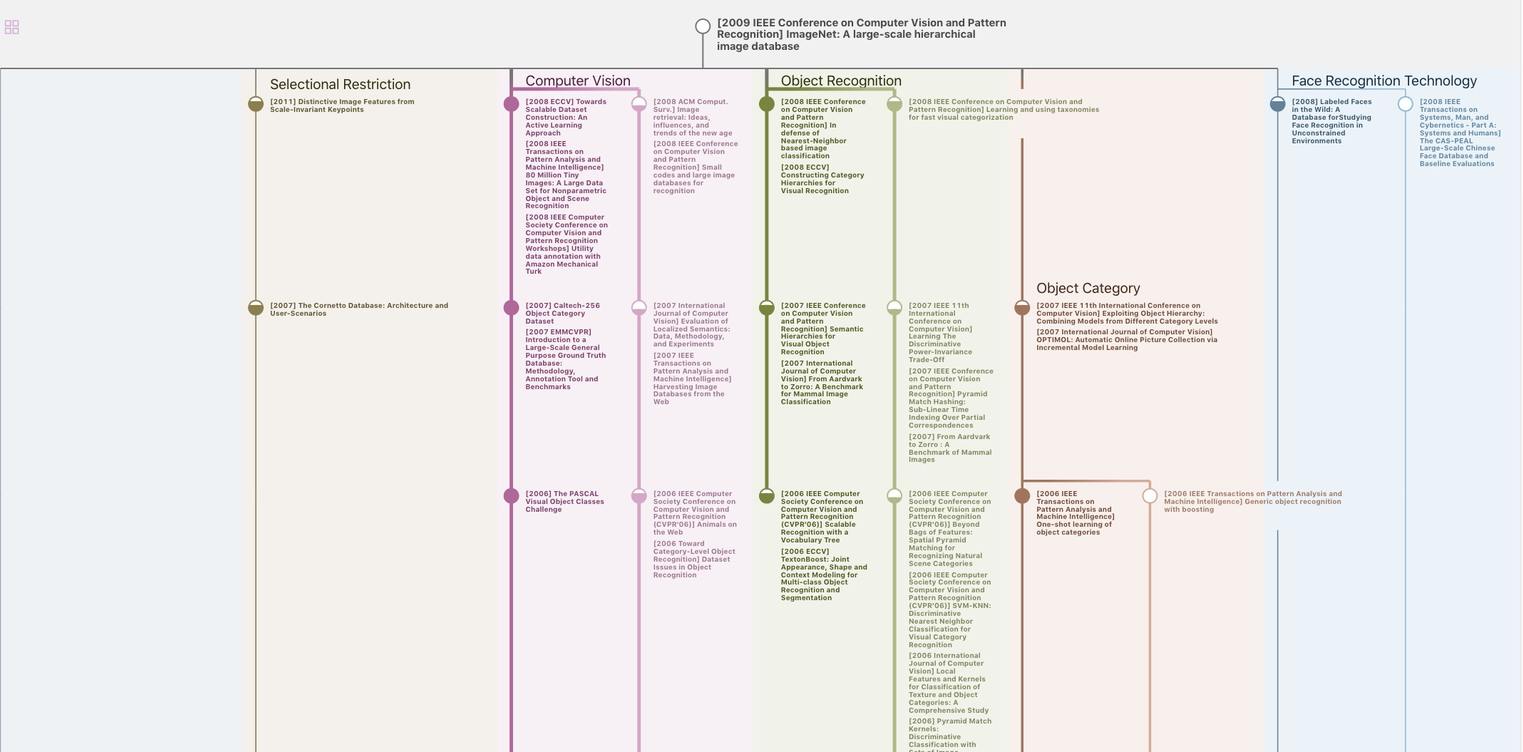
生成溯源树,研究论文发展脉络
Chat Paper
正在生成论文摘要