End To End Learning Of A Multi-Layered Snn Based On R-Stdp For A Target Tracking Snake-Like Robot
2019 INTERNATIONAL CONFERENCE ON ROBOTICS AND AUTOMATION (ICRA)(2019)
摘要
This paper introduces an end-to-end learning approach based on Reward-modulated Spike-Timing-Dependent Plasticity (R-STDP) for a multi-layered spiking neural network (SNN). As a case study, a snake-like robot is used as an agent to perform target tracking tasks on the basis of our proposed approach. Since the key of R-STDP is to use rewards to modulate synapse strengthens, we first propose a general way to propagate the reward back through a multi-layered SNN. Upon the proposed approach, we build up an SNN controller that drives a snake-like robot for performing target tracking tasks. We demonstrate the practicability and advantage of our approach in terms of lateral tracking accuracy by comparing it to other state-of-the-art learning algorithms for SNNs based on R-STDP.
更多查看译文
关键词
multilayered SNN,target tracking snake-like robot,end-to-end learning approach,R-STDP,SNN controller,target tracking tasks,reward-modulated spike-timing-dependent plasticity,multilayered spiking neural network,learning algorithms,lateral tracking
AI 理解论文
溯源树
样例
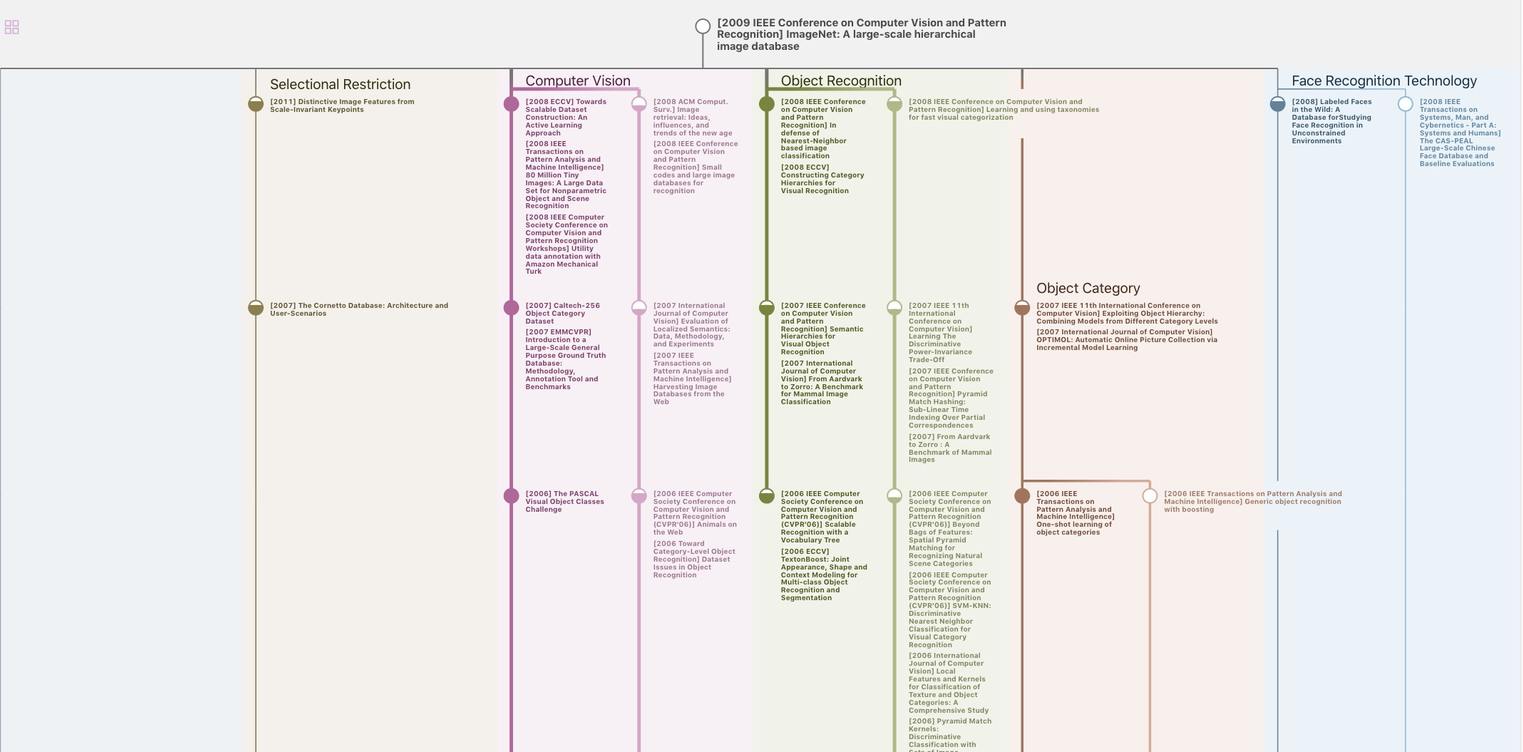
生成溯源树,研究论文发展脉络
Chat Paper
正在生成论文摘要