A Personal Location Prediction Method to Solve the Problem of Sparse Trajectory Data
2019 20TH INTERNATIONAL CONFERENCE ON MOBILE DATA MANAGEMENT (MDM 2019)(2019)
摘要
The rapid development of information and communication technology and the popularization of mobile devices have generated a large number of spatiotemporal trajectory data. Trajectory data can be applied to location prediction, which is significant for urban traffic planning and location-based service. Although various methods for personal location prediction have been proposed, the historical trajectory data of some users is always sparse in practical applications, resulting in poor prediction precision of prediction models based on personal historical data for those sparse users. Targeting on this challenge, we propose an "Individual trajectory-Group trajectory assist Individual trajectory" location prediction model (ITGTAIT) by utilizing the group travel patterns to assist in predicting personal locations. First, the model conducts a spatial clustering algorithm on trajectory points to construct the clustering link. Second, the clustering link and Fano's inequality are used to estimate the predictability of the next location. Third, a Variable Order Markov Model that named Prediction by Partial Match (PPM) was adopted to predict the clustering link based on the individual trajectory for users with sufficient data. For users with sparse samples, the PPM utilizes the pattern of group travels, which using the group trajectory to assist individual trajectory. Finally, our method was evaluated by using 608,712 trajectory points from 5000 volunteers at Shenzhen city, China. The result shows that a) with the increase of training data, the precision of the ITGTAIT is gradually stable, b) for users with over four days of data, the highest precision is 87.11%, stable at about 82%, c) for users with only 1-3 days of data, the prediction precision is 55.15%, 67.04%, and 76.86% respectively, which introduces approximately 10.76%, 10.98% and 6.83% performance gains on location predictions respectively by utilizing the group characters.
更多查看译文
关键词
location prediction, spatiotemporal trajectory, Variable Order Markov Model, Prediction by Partial Match (PPM), sparse samples
AI 理解论文
溯源树
样例
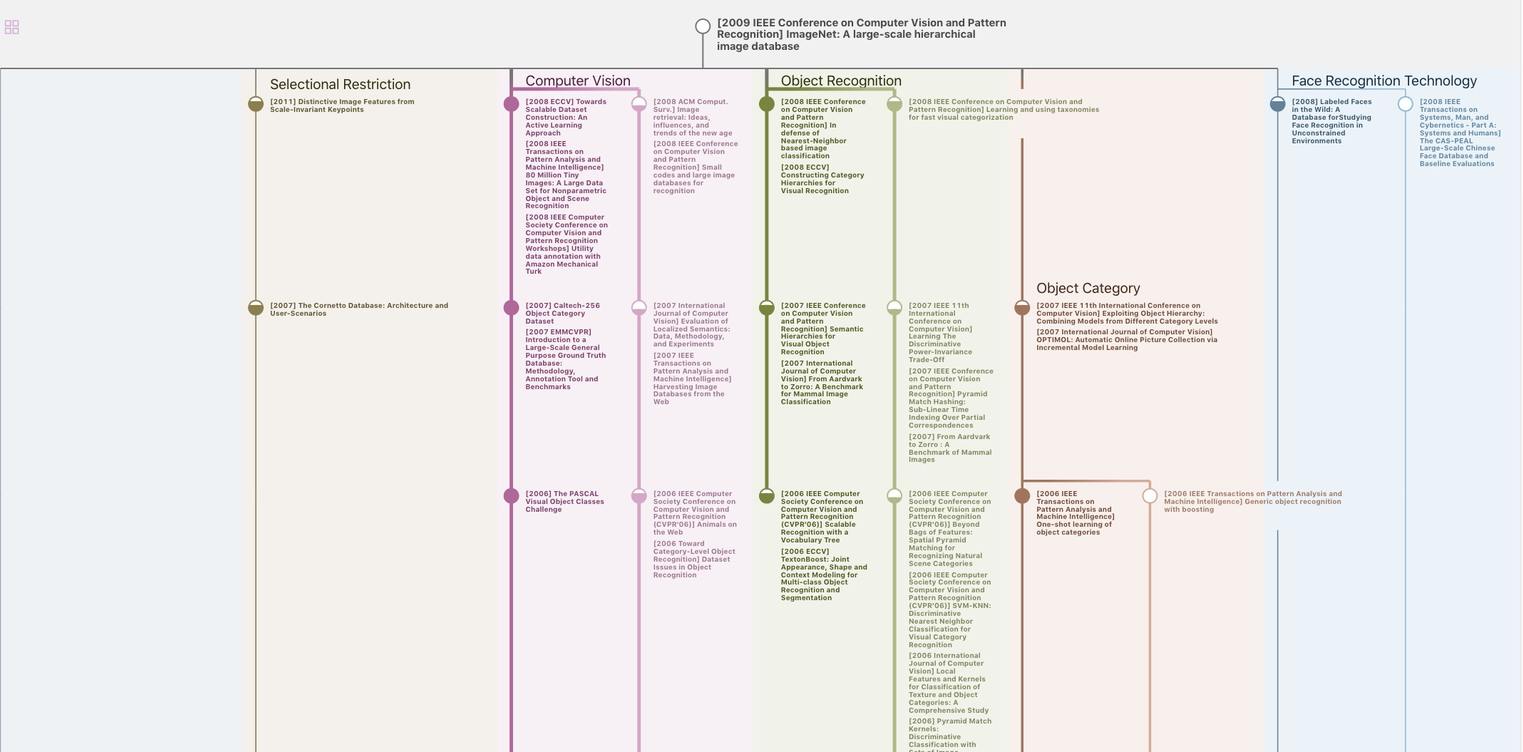
生成溯源树,研究论文发展脉络
Chat Paper
正在生成论文摘要