Bayesian kernel machine regression-causal mediation analysis
STATISTICS IN MEDICINE(2022)
摘要
Greater understanding of the pathways through which an environmental mixture operates is important to design effective interventions. We present new methodology to estimate natural direct and indirect effects and controlled direct effects of a complex mixture exposure on an outcome through a mediator variable. We implement Bayesian Kernel Machine Regression (BKMR) to allow for all possible interactions and nonlinear effects of (1) the co-exposures on the mediator, (2) the co-exposures and mediator on the outcome, and (3) selected covariates on the mediator and/or outcome. From the posterior predictive distributions of the mediator and outcome, we simulate counterfactuals to obtain posterior samples, estimates, and credible intervals of the mediation effects. Our simulation study demonstrates that when the exposure-mediator and exposure-mediator-outcome relationships are complex, BKMR-Causal Mediation Analysis performs better than current mediation methods. We applied our methodology to quantify the contribution of birth length as a mediator between in utero co-exposure to arsenic, manganese, and lead, and children's neurodevelopmental scores, in a prospective birth cohort in Bangladesh. Among younger children, we found a negative (adverse) association between the metal mixture and neurodevelopment. We also found evidence that birth length mediates the effect of exposure to the metal mixture on neurodevelopment for younger children. If birth length were fixed to its 75th percentile value, the harmful effect of the metal mixture on neurodevelopment is attenuated, suggesting nutritional interventions to help increase fetal growth, and thus birth length, could potentially block the harmful effect of the metal mixture on neurodevelopment.
更多查看译文
关键词
children's neurodevelopment, environmental mixture, mixture, multipollutant exposure
AI 理解论文
溯源树
样例
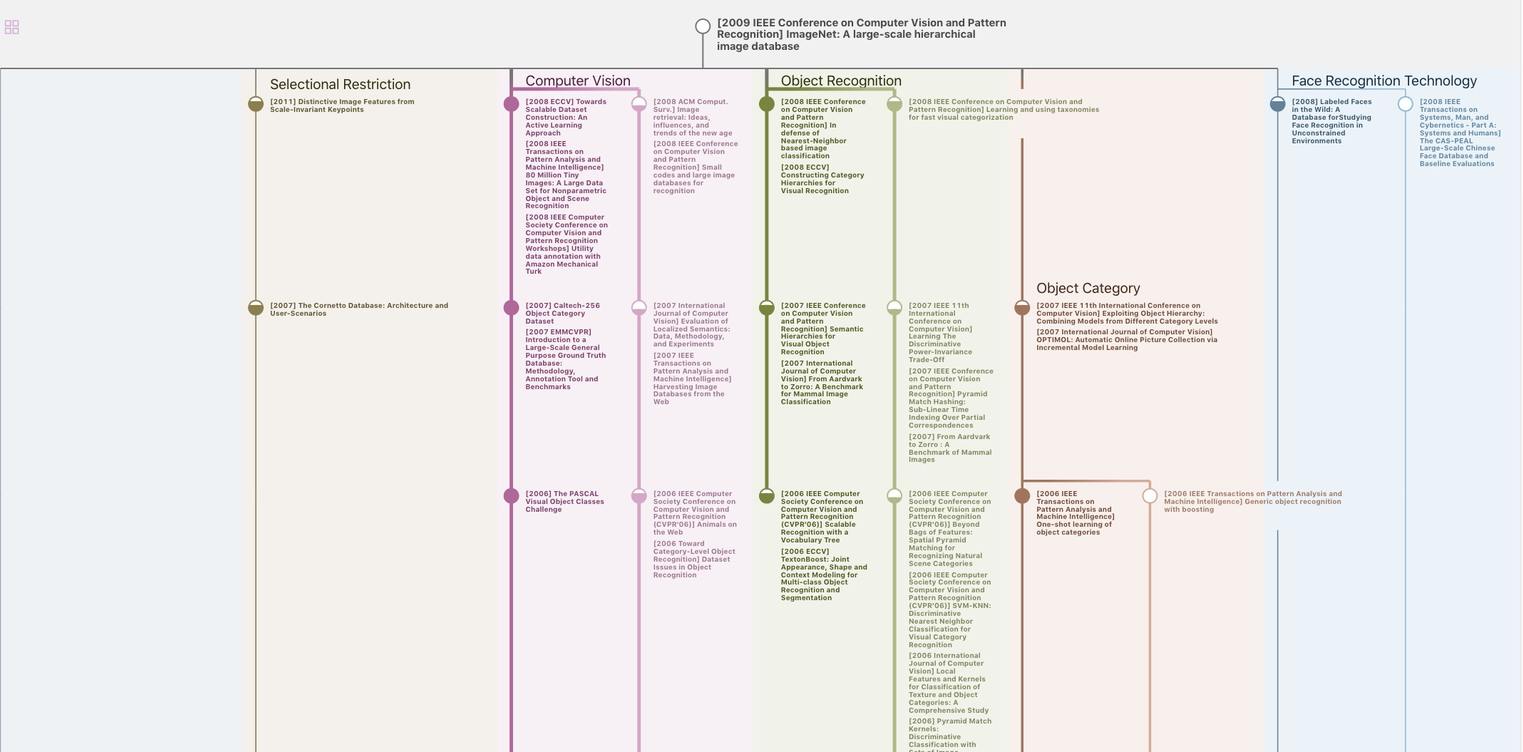
生成溯源树,研究论文发展脉络
Chat Paper
正在生成论文摘要