Robust Multi-View Hashing For Cross-Modal Retrieval
2019 IEEE INTERNATIONAL CONFERENCE ON MULTIMEDIA AND EXPO (ICME)(2019)
摘要
Existing hashing methods barely explore the information loss problem during learning the common semantic subspace, thus retrieval performance may be degraded. Besides, these methods mainly rely on the inter-modality or intra-modality correlations separately and fail to exploit the full structure reflected by these correlations. To address these problems, we present a novel cross-modal hashing method, namely Robust Multi-View Hashing (RMVH). To learn a robust latent semantic subspace, we enforce the learnt representations to well reconstruct original features such that more important information can be retained. To comprehensively exploit the relationship between representations of multiple modalities, we utilize Multi-View Learning to construct an affinity matrix to guide the learning of common latent semantic subspace, which can preserve both inter-modality and intra-modality similarities. Instead of relaxing the binary constraints, we leverage the label information to learn hash codes discretely which can avoid the large quantization error and preserve the semantic similarity. Experimental results on three benchmark datasets show that the proposed RMVH achieves superior performance compared with other state-of-the-art methods.
更多查看译文
关键词
Cross-modal retrieval, similarity learning, hashing, discrete optimization
AI 理解论文
溯源树
样例
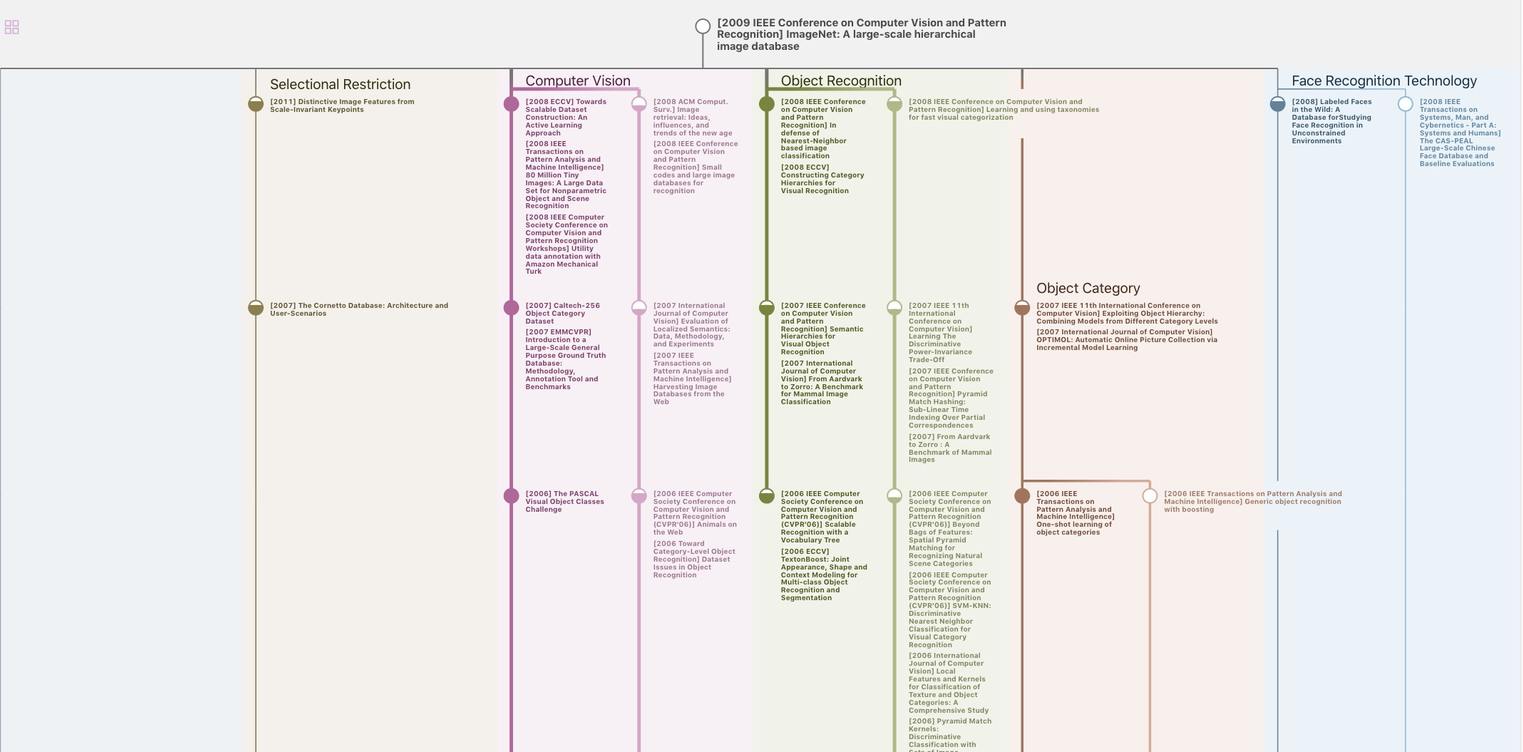
生成溯源树,研究论文发展脉络
Chat Paper
正在生成论文摘要