Multi-View Learning for Vehicle Re-Identification
2019 IEEE International Conference on Multimedia and Expo (ICME)(2019)
摘要
Vehicle re-identification (ReID) aims to identify a target vehicle in different cameras with non-overlapping views, and it plays an important role when the car licence plate recognition is unavailable or unreliable. Compared with face recognition and person ReID tasks, it is difficult to train an effective vehicle ReID model due to two reasons: the different views greatly affect the visual appearance of a vehicle, and different vehicles may exhibit fairly similar visual appearance when their images are captured from one unified single view. To handle these training difficulties, we introduce several latent groups to represent multiple views. Then, the vehicle ReID problem is modeled as two sub tasks, including matching vehicles in a same view and across different views. A fine-grain ranking loss and a relative coarse-grain ranking loss are proposed to each task respectively. Extensive experimental analyses and evaluations on two benchmarks demonstrate the proposed method can achieve state-of-the-art performance.
更多查看译文
关键词
Vehicle re-identification,Multi-grain loss,Multi-view learning
AI 理解论文
溯源树
样例
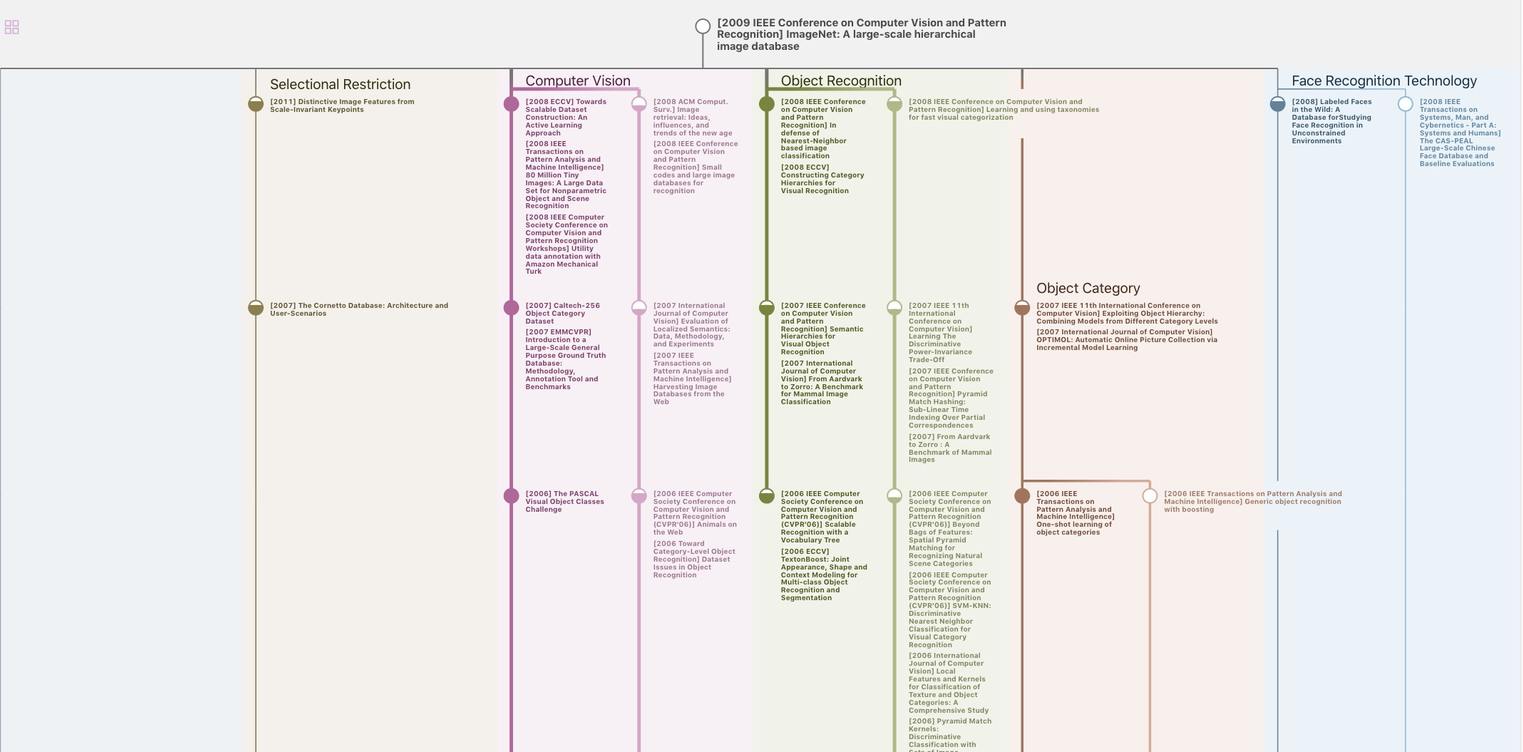
生成溯源树,研究论文发展脉络
Chat Paper
正在生成论文摘要