Prediction of hERG K+ Channel Blockage Using Deep Neural Networks.
CHEMICAL BIOLOGY & DRUG DESIGN(2019)
摘要
Human ether-a-go-go-related gene (hERG) K+ channel blockage may cause severe cardiac side-effects and has become a serious issue in safety evaluation of drug candidates. Therefore, improving the ability to avoid undesirable hERG activity in the early stage of drug discovery is of significant importance. The purpose of this study was to build predictive models of hERG activity by deep neural networks. For each combination of sampling methods and descriptors, deep neural networks with different architectures were implemented to build classification models. The optimal model M15 with three hidden layers, undersampling method, and 2D descriptors yielded the prediction accuracy of 0.78 and F1 score of 0.75 on the test set as well as accuracy of 0.77 and F1 score of 0.34 on the external validation set, outperforming the other 35 models including 9 random forest models. Particularly, the optimal model M15 achieved the highest F1 score and the second highest accuracy when compared with other five methods from four groups using different machine learning algorithms with the same external validation set. It can be believed that this model has powerful capability on prediction of hERG toxicity, which is of great benefit for developing novel drug candidates.
更多查看译文
关键词
deep neural networks,drug discovery,hERG toxicity,random forest
AI 理解论文
溯源树
样例
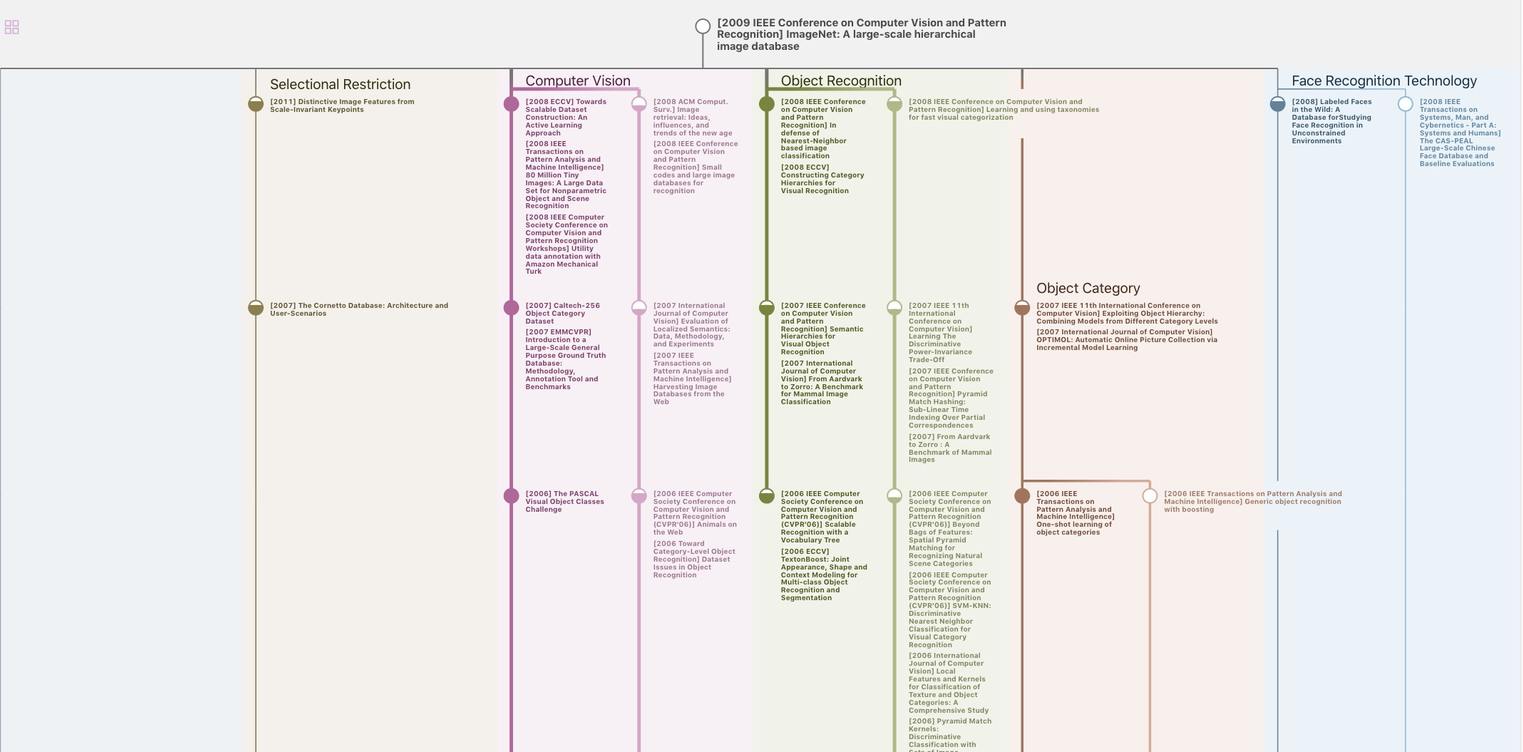
生成溯源树,研究论文发展脉络
Chat Paper
正在生成论文摘要