An Effective Framework for Identifying Good XML Feedback Fragments.
ADVANCES IN COMPUTER SCIENCE AND UBIQUITOUS COMPUTING(2018)
Abstract
In Pseudo relevance feedback, It is often crucial to identify those good feedback documents from which useful expansion terms can be added to the query. For Extensible Markup Language (XML) data, this paper proposes an approach for identifying good feedback fragments by a complete framework in which two phrases are included. (1) The first phase is about XML search results clustering. We performed a k-medoid clustering algorithm to XML fragments based on an extended latent semantic indexing model. (2) The second phase is a two-stage ranking. Cluster ranking is performed in the first stage to select relevant clusters on the basis of cluster labelling, which is determined by extracted fragment keywords based on a combination of weight and context; fragment ranking is performed during the second stage where multiple features are used to identify high quality fragments from the previously obtained relevant clusters. Experimental results on standard INEX test data show that the proposed approach achieves statistically significant improvements over a strong original query results mechanism, ensuring high quality fragments for feedback.
MoreTranslated text
Key words
XML fragment,Clustering search results,Two-stage ranking model,Pseudo relevance feedback
AI Read Science
Must-Reading Tree
Example
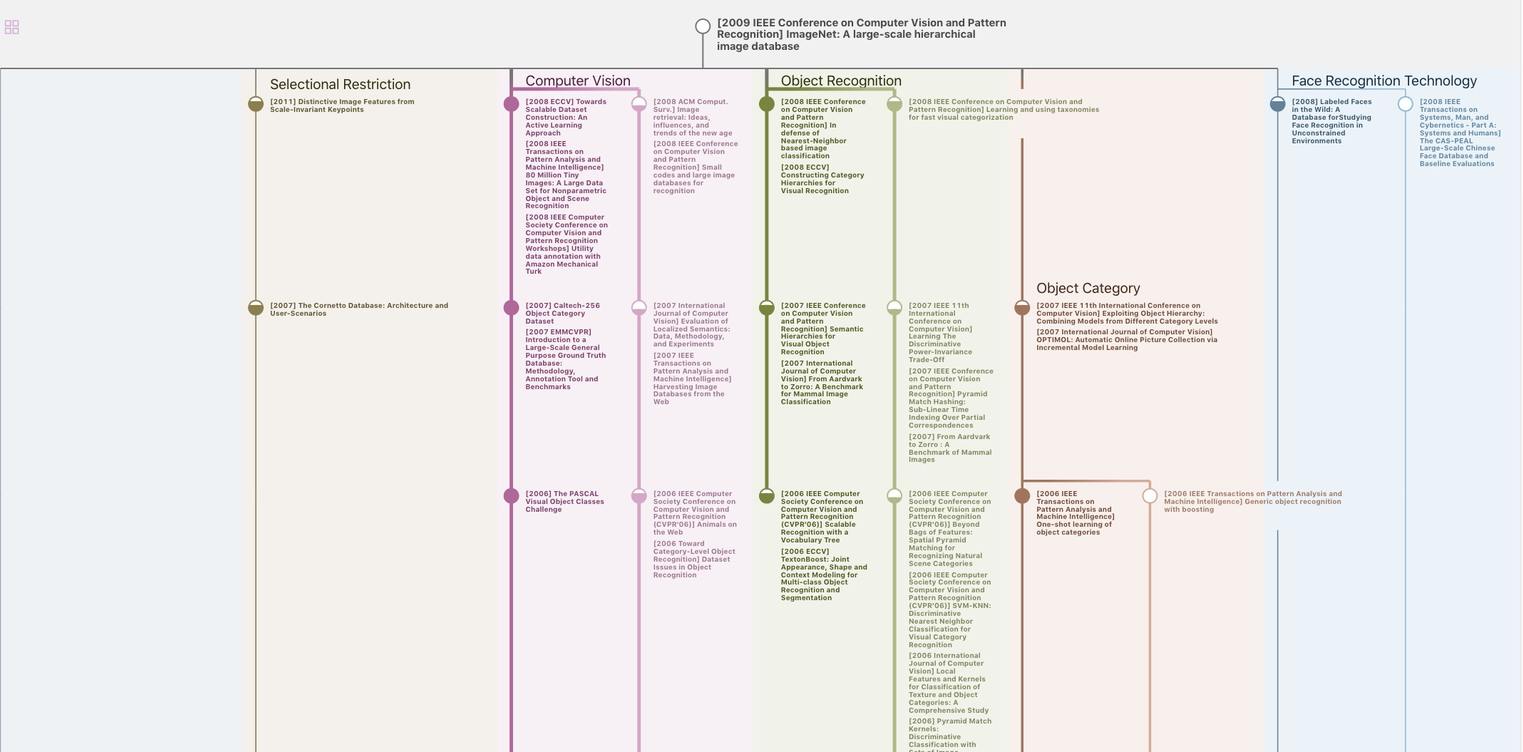
Generate MRT to find the research sequence of this paper
Chat Paper
Summary is being generated by the instructions you defined