BN-invariant Sharpness Regularizes the Training Model to Better Generalization.
IJCAI(2019)
摘要
It is arguably believed that flatter minima can generalize better. However, it has been pointed out that the usual definitions of sharpness, which consider either the maxima or the integral of loss over a $\delta$ ball of parameters around minima, cannot give consistent measurement for scale invariant neural networks, e.g., networks with batch normalization layer. In this paper, we first propose a measure of sharpness, BN-Sharpness, which gives consistent value for equivalent networks under BN. It achieves the property of scale invariance by connecting the integral diameter with the scale of parameter. Then we present a computation-efficient way to calculate the BN-sharpness approximately i.e., one dimensional integral along the "sharpest" direction. Furthermore, we use the BN-sharpness to regularize the training and design an algorithm to minimize the new regularized objective. Our algorithm achieves considerably better performance than vanilla SGD over various experiment settings.
更多查看译文
关键词
generalization,training model,bn-invariant
AI 理解论文
溯源树
样例
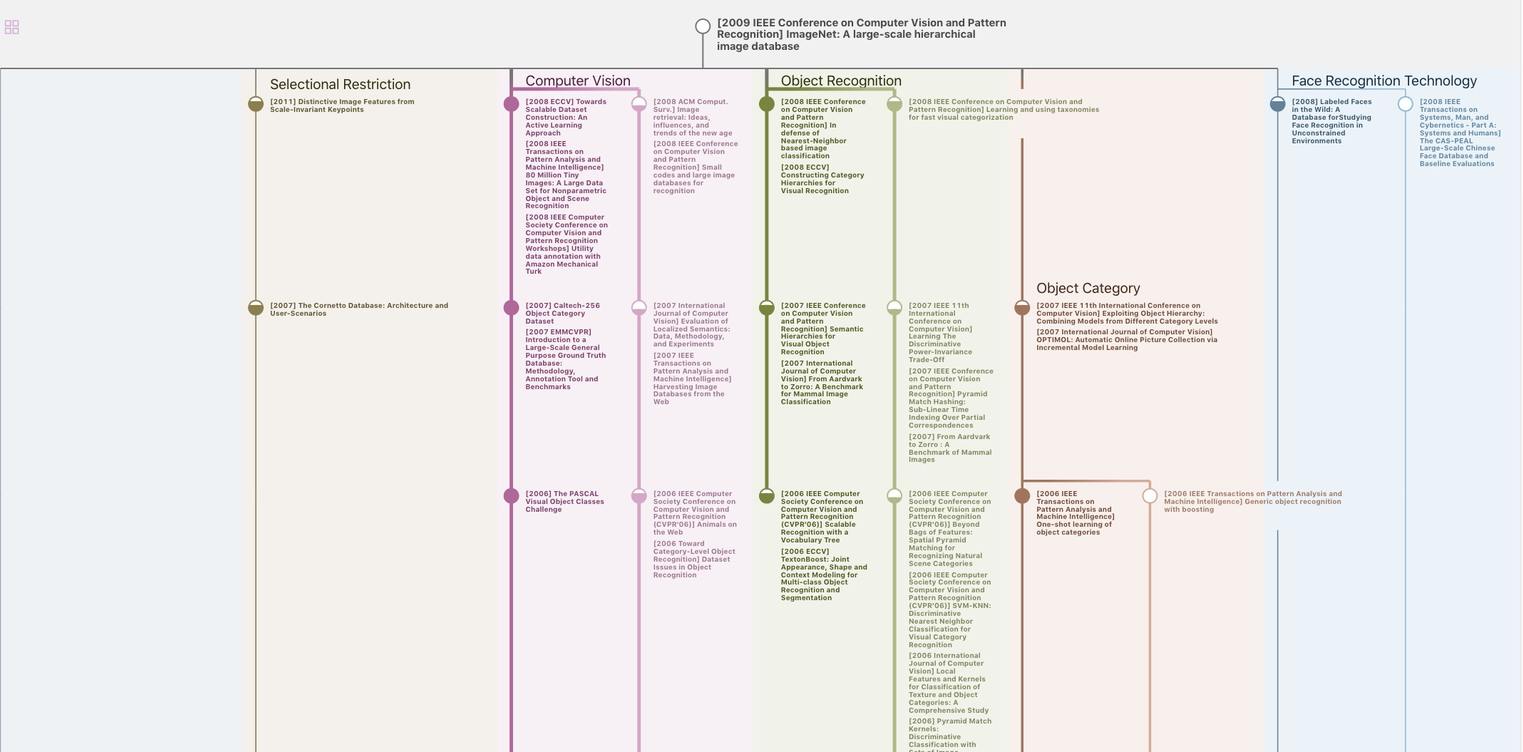
生成溯源树,研究论文发展脉络
Chat Paper
正在生成论文摘要