SSFD+: A Robust Two-Stage Face Detector
IEEE Transactions on Biometrics, Behavior, and Identity Science(2019)
摘要
Face detectors based on deep learning have demonstrated great progress in detecting multi-scale faces by using multi-scale feature maps and input pyramids. However, using input pyramids and multi-scale feature maps increases the training difficulty and complexity of the network. In this paper, we focus on achieving comparable performance and simplifying the network architecture for detecting multi-scale faces. To enable network learning of multi-scale facial features from a single-scale feature map and a single-scale input image: 1) we conducted a comparative study to investigate which layer contributes more to detecting multi-scale faces and 2) we designed and implemented a simple network structure to improve the performance of detecting multi-scale faces by incorporating additional contextual information. SSFD
+
achieves mAPs of (91.3%, 90.3%, 83.1%) and (92.4%, 90.9%, 83.7%) on the (easy, medium, and hard) subsets of the WIDER FACE validation and testing datasets, respectively, and promising results on the FDDB, PASCAL Faces, and AFW datasets.
更多查看译文
关键词
Face,Feature extraction,Detectors,Convolution,Facial features,Training
AI 理解论文
溯源树
样例
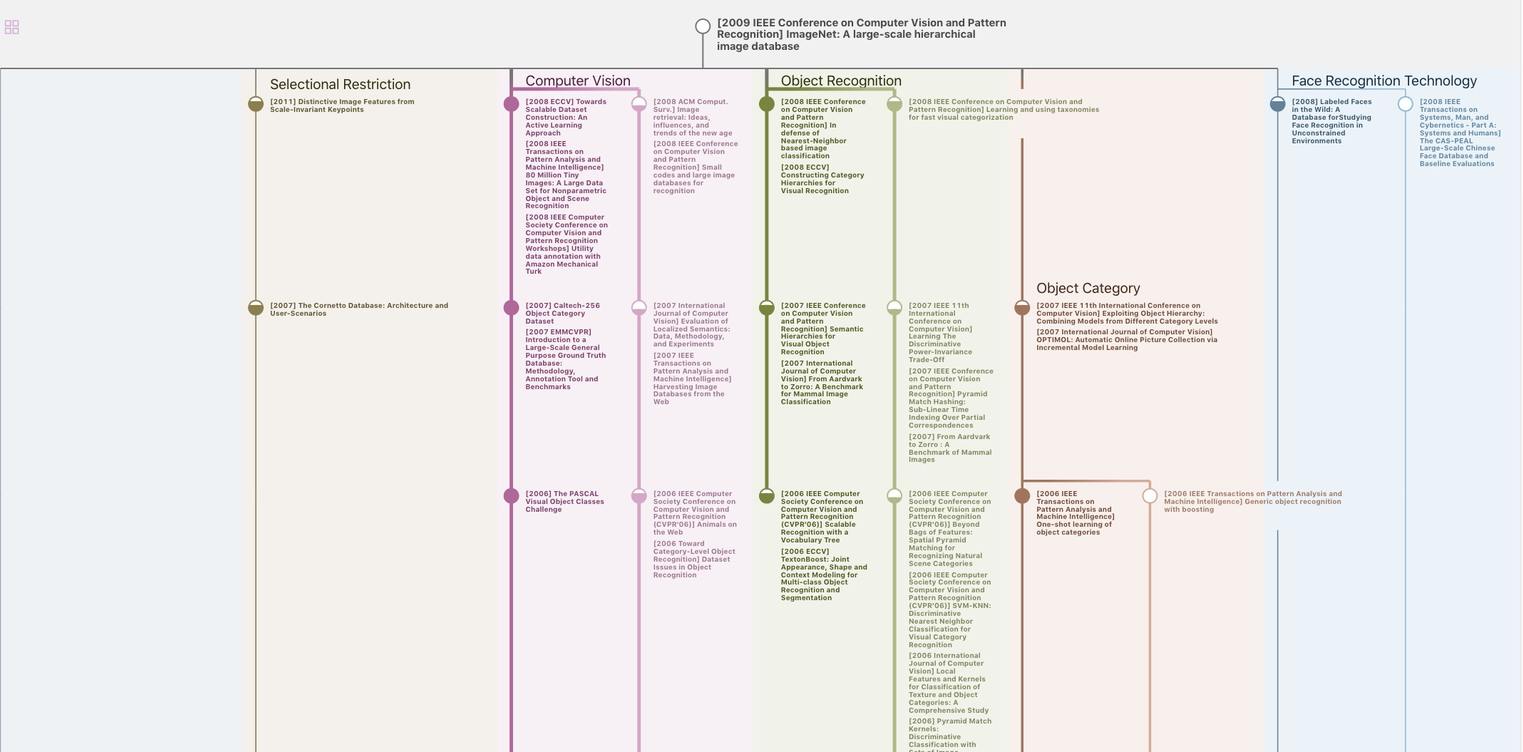
生成溯源树,研究论文发展脉络
Chat Paper
正在生成论文摘要