Scaling Geo-Distributed Network Function Chains: A Prediction and Learning Framework
IEEE Journal on Selected Areas in Communications(2019)
Abstract
Geo-distributed virtual network function (VNF) chaining has been useful, such as in network slicing in 5G networks and for network traffic processing in the WAN. Agile scaling of the VNF chains according to real-time traffic rates is the key in network function virtualization. Designing efficient scaling algorithms is challenging, especially for geo-distributed chains, where bandwidth costs and latencies incurred by the WAN traffic are important but difficult to handle in making scaling decisions. Existing studies have largely resorted to optimization algorithms in scaling design. Aiming at better decisions empowered by in-depth learning from experiences, this paper proposes a deep learning-based framework for scaling of the geo-distributed VNF chains, exploring inherent pattern of traffic variation and good deployment strategies over time. We novelly combine a recurrent neural network as the traffic model for predicting upcoming flow rates and a deep reinforcement learning (DRL) agent for making chain placement decisions. We adopt the experience replay technique based on the actor–critic DRL algorithm to optimize the learning results. Trace-driven simulation shows that with limited offline training, our learning framework adapts quickly to traffic dynamics online and achieves lower system costs, compared to the existing representative algorithms.
MoreTranslated text
Key words
Reinforcement learning,Heuristic algorithms,Artificial neural networks,Data centers,Wide area networks,Adaptation models,Predictive models
AI Read Science
Must-Reading Tree
Example
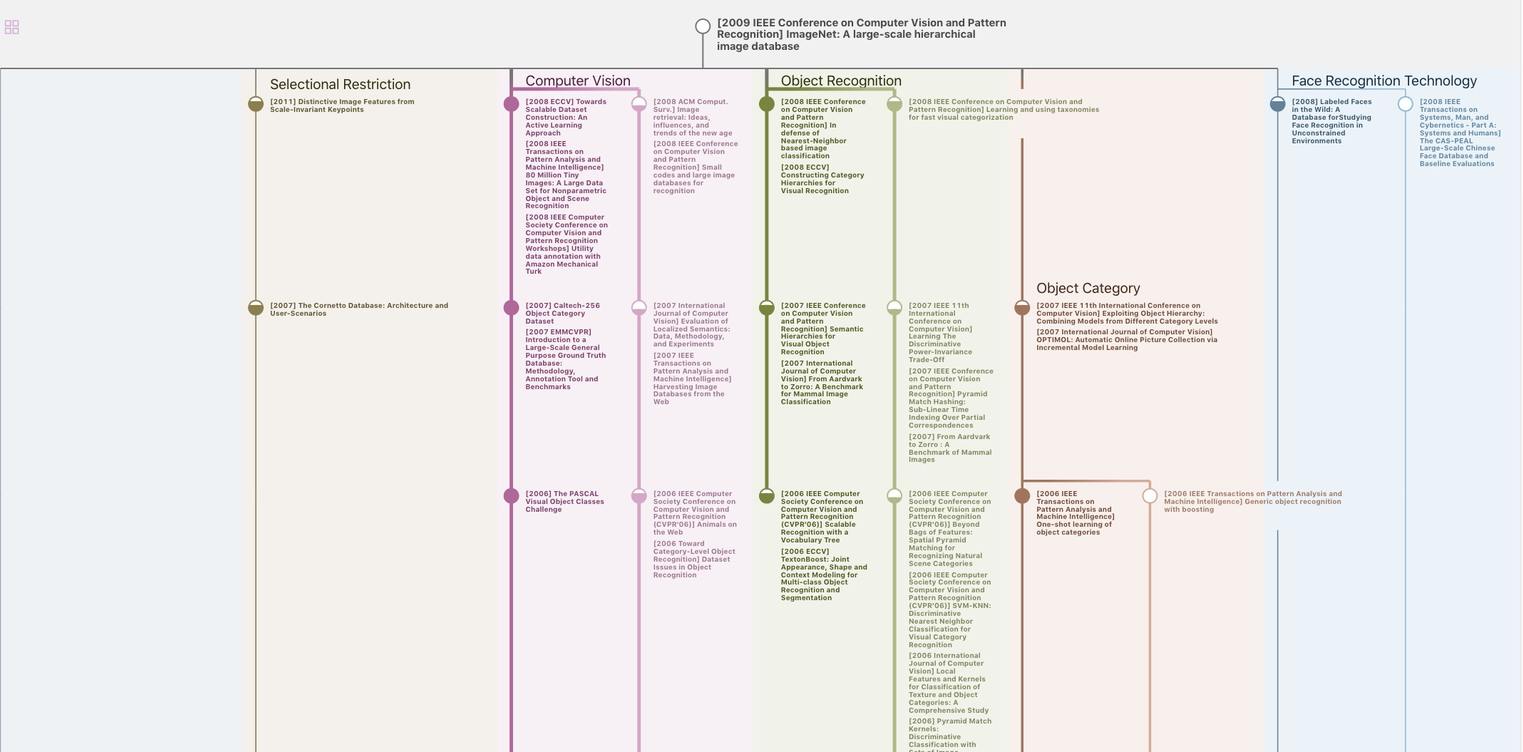
Generate MRT to find the research sequence of this paper
Chat Paper
Summary is being generated by the instructions you defined