Toward Efficient In-memory Data Analytics on NUMA Systems
arxiv(2019)
摘要
Data analytics systems commonly utilize in-memory query processing techniques to achieve better throughput and lower latency. Modern computers increasingly rely on Non-Uniform Memory Access (NUMA) architectures in order to achieve scalability. A key drawback of NUMA architectures is that many existing software solutions are not aware of the underlying NUMA topology and thus do not take full advantage of the hardware. Modern operating systems are designed to provide basic support for NUMA systems. However, default system configurations are typically sub-optimal for large data analytics applications. Additionally, achieving NUMA-awareness by rewriting the application from the ground up is not always feasible. In this work, we evaluate a variety of strategies that aim to accelerate memory-intensive data analytics workloads on NUMA systems. We analyze the impact of different memory allocators, memory placement strategies, thread placement, and kernel-level load balancing and memory management mechanisms. With extensive experimental evaluation we demonstrate that methodical application of these techniques can be used to obtain significant speedups in four commonplace in-memory data analytics workloads, on three different hardware architectures. Furthermore, we show that these strategies can speed up two popular database systems running a TPC-H workload.
更多查看译文
AI 理解论文
溯源树
样例
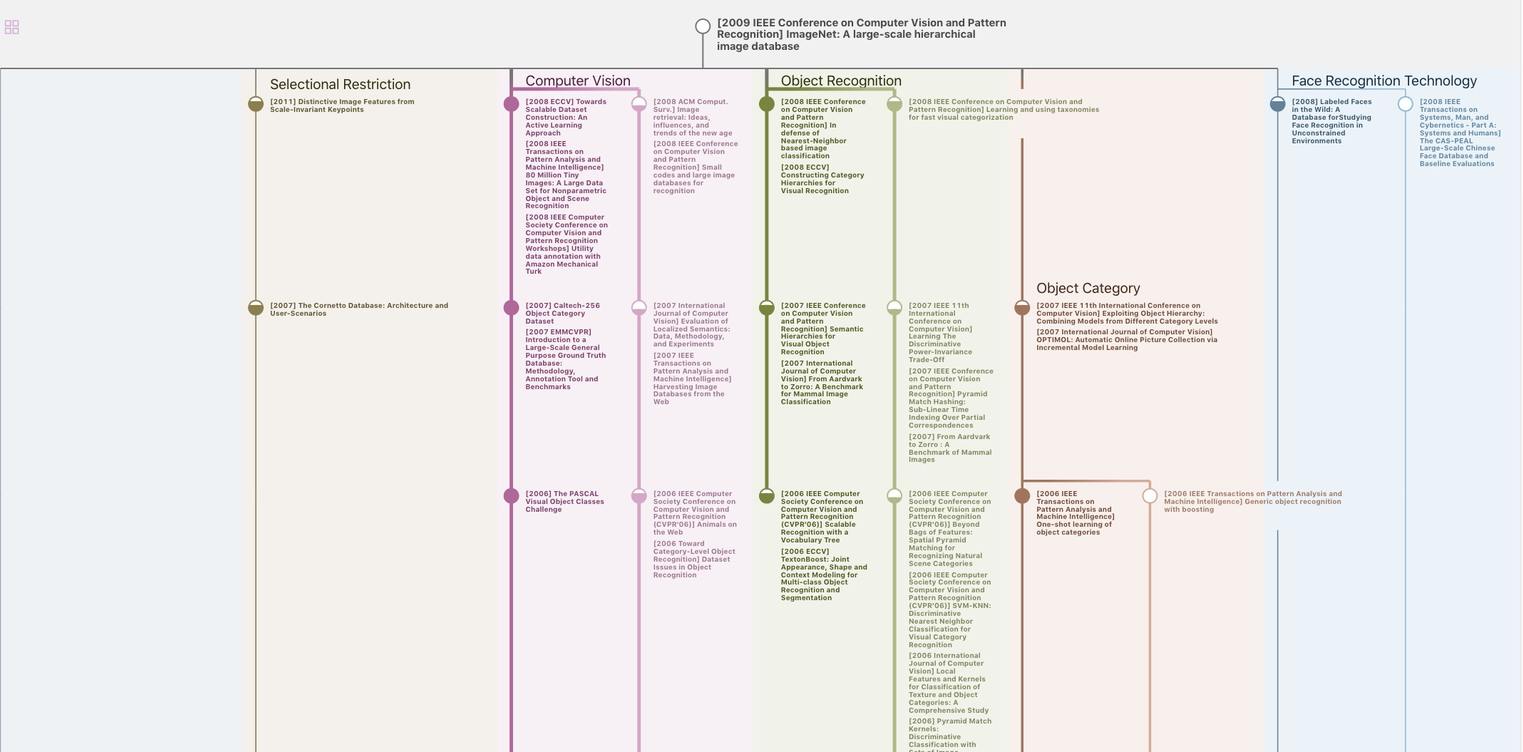
生成溯源树,研究论文发展脉络
Chat Paper
正在生成论文摘要