Recommending Points of Interest in LBSNs Using Deep Learning Techniques
2019 IEEE International Symposium on INnovations in Intelligent SysTems and Applications (INISTA)(2019)
摘要
The representation of real-life problems by using k-partite graphs introduced a new era in Machine Learning. Moreover, the merge of virtual and physical layers through Location Based Social Networks (LBSN s) offers a different meaning into the constructed graphs. To this point, multiple models introduced in literature that aim to support users with personalized recommendations. These approaches represent the mathematical models that aim to understand users' behaviour by finding patterns on users' check-ins, reviews, ratings, friendships, etc. With this paper we describe and compare 20 of those state-of-the-art deep learning models to bring into the surface some of their strengths and shortcomings. First, we categorize them according to: data factors or features they use, data representation, methodologies used and recommendation types they support. Then, we highlight the existing limitations that tackles their performance. Finally, we introduce research trends and future directions.
更多查看译文
关键词
Deep learning,Location Based Social Networks,survey,limitations,new directions
AI 理解论文
溯源树
样例
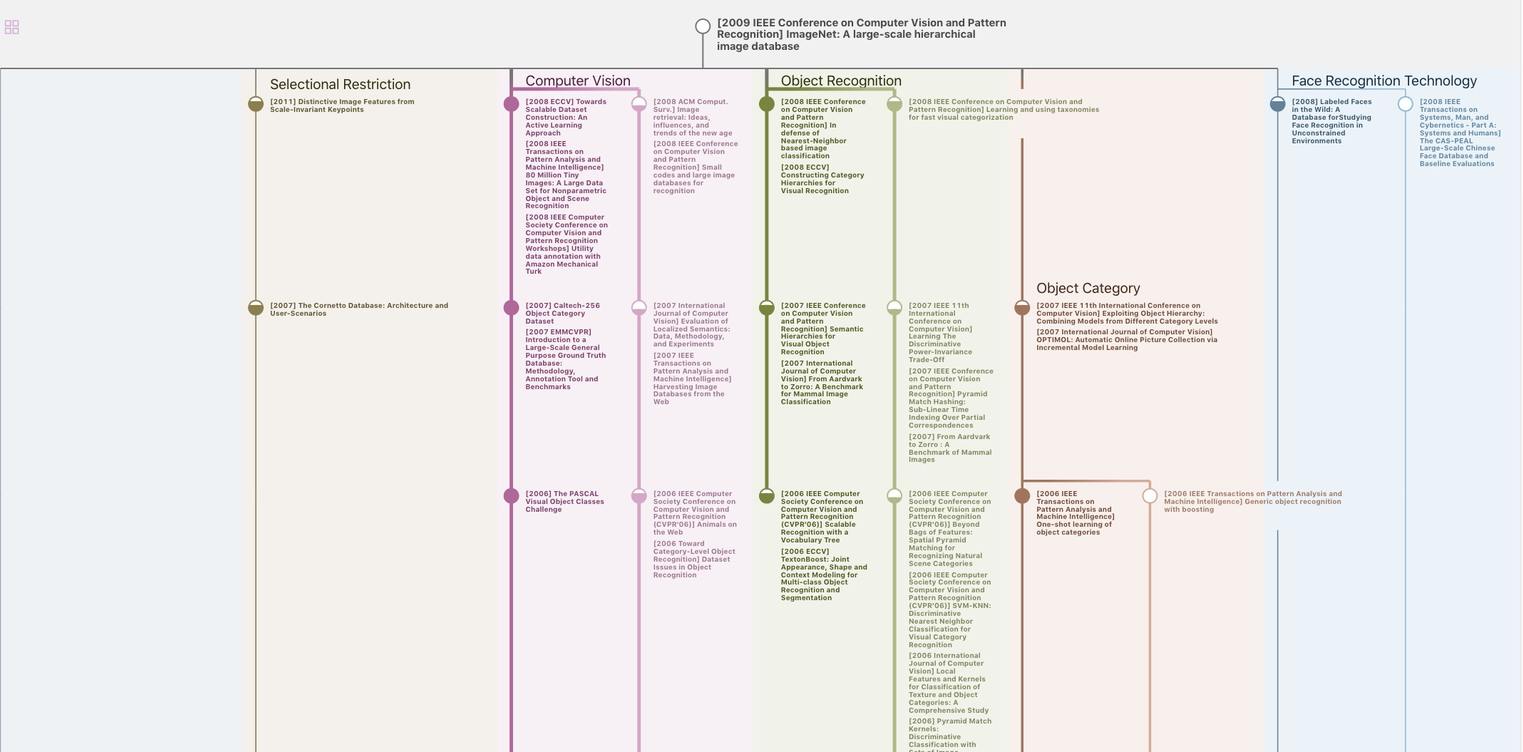
生成溯源树,研究论文发展脉络
Chat Paper
正在生成论文摘要