V-Awake: A Visual Analytics Approach for Correcting Sleep Predictions from Deep Learning Models.
COMPUTER GRAPHICS FORUM(2019)
摘要
The usage of deep learning models for tagging input data has increased over the past years because of their accuracy and high-performance. A successful application is to score sleep stages. In this scenario, models are trained to predict the sleep stages of individuals. Although their predictive accuracy is high, there are still mis classifications that prevent doctors from properly diagnosing sleep-related disorders. This paper presents a system that allows users to explore the output of deep learning models in a real-life scenario to spot and analyze faulty predictions. These can be corrected by users to generate a sequence of sleep stages to be examined by doctors. Our approach addresses a real-life scenario with absence of ground truth. It differs from others in that our goal is not to improve the model itself, but to correct the predictions it provides. We demonstrate that our approach is effective in identifying faulty predictions and helping users to fix them in the proposed use case.
更多查看译文
AI 理解论文
溯源树
样例
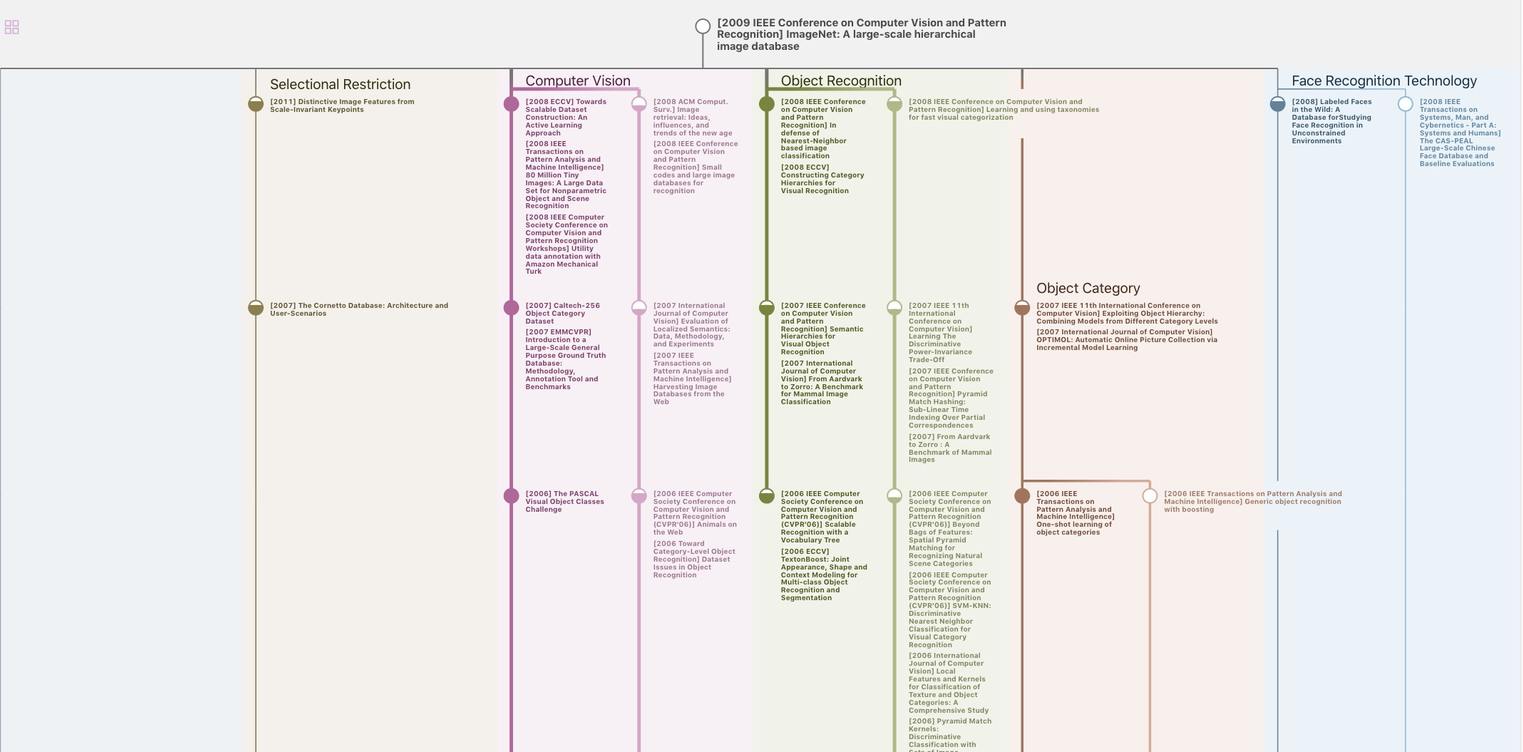
生成溯源树,研究论文发展脉络
Chat Paper
正在生成论文摘要